Clinical evaluation of self-learning GAN based pseudo-CT generation software for low field pelvic MR
MO-0648
Abstract
Clinical evaluation of self-learning GAN based pseudo-CT generation software for low field pelvic MR
Authors: Pascal Fenoglietto1, Thierry Gevaert2, Marlies Boussaer2, Edoaurd Delasalles3, Despoina Ioannidou3, Kumar Shreshtha3, Thais Roque4, Nikos Paragios5,6, David Azria1, Enis Ozyar7, Gorkem Gungor7
1L'Institut du Cancer de Montpellier, Department of Radiation Oncology, Montpellier , France; 2UZ Brussel, Vrije Universiteit Brussel, Radiotherapy Department, Brussels, Belgium; 3TheraPanacea, AI Research Department, Paris, France; 4TheraPanacea, Clinical and Partnerships Affairs, Paris, France; 5TheraPanacea, Research and Development, Paris, France; 6Centrale Supelec, University of Paris-Saclay, Computer Science and Applied Mathematics, Gif-sur-Yvette, France; 7Acibadem MAA University, Maslak Hospital, Radiation Oncology, Istanbul, Turkey
Show Affiliations
Hide Affiliations
Purpose or Objective
MR-guided radiotherapy (MRgRT)
allows plan adaptation on the MRI of the day, offering new perspectives for
pelvic cancer care. Recent developments on pseudo-CTs (pCT) and hybrid MRI
linacs underlined the clinical feasibility and acceptance of MRgRT. However,
the low field MR for ViewRay MRIdian® TrueFISP, with a limited field of view
can affect the MR to pCT conversion with methods such as bulk electron density (ED)
overwrite. These methods do not only introduce uncertainties due to assignment
of mean EDs, but still require a planning CT. In this study an artificial
intelligence-based pseudo CTs (AICT) is proposed and clinically evaluated to
overcome these challenges and unlock the full potential of MRgRT for pelvic
cancer care.
Material and Methods
For the case of low field pelvis
MR-based daily treatment adaptation, transfer learning was applied to an
automatic synthetic-CT generation tool from unpaired pelvis MRIs that uses
ensembled self-supervised GANs. Seventeen prostate cancer patients treated on
the low field MR-Linac at three European cancer care excellence centers were
selected for this evaluation. Planning CTs were deformably registered to the
MRIs for each patient. Treatment plans were optimized on the planning CT with a clinical TPS fulfilling all clinical criteria and recalculated on the
warped CT (wCT) and the pCT for image and dosimetric evaluation. For the
analysis, wCTs and pCTs were compared based on a) mean absolute errors (MAE),
b) DVH-parameters (D2%, D50%, D95%, D98% and Dmean) for the CTV and PTV, and c)
dose distributions compared with global gamma criteria.
Results
The mean MAE for the whole body,
rectum, bladder, and prostate are 58.45+/-113.86 HU, 63.49 +/- 89.85 HU, 26.12
+/- 25.52 HU and 34.08 +/- 51.06, respectively, demonstrating good image
agreement between the wCT and the pCT. The pCT reproduced for all patients the
dose distribution based on a gamma criterion of 2mm/2% with a mean pass rate greater
than 99% (no threshold applied). The PTV DVH statistics reported were within 2%
for all cases except 3 cases from center 3, which led to a slight increase in
the DVH mean relative errors for this center (Table 1). On average, relative
errors for all PTV and CTV DVH statistics were within 2%, which is below what
is reported in the literature for bulk pCT for low field MRgRT.
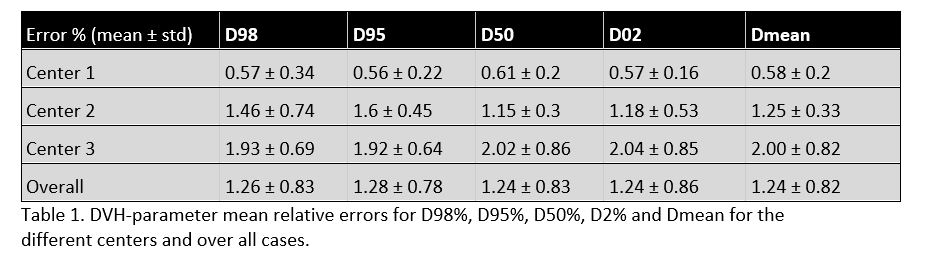
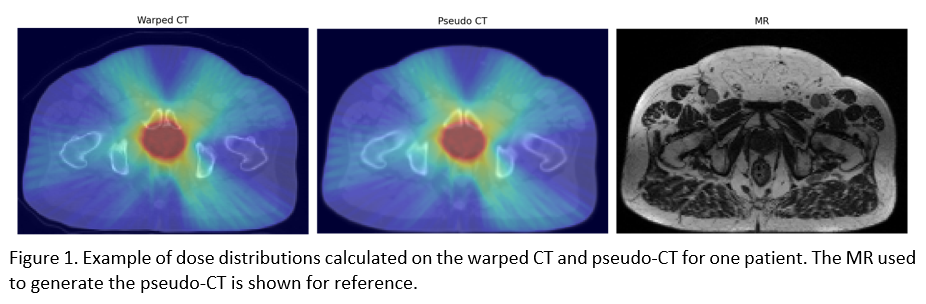
Conclusion
This study demonstrated the
feasibility of generating clinically acceptable synthetic CT using an AICT tool
from low field MR. Results were comparable for sCT and CT images in both
dosimetric and MAE evaluation. This tool which can be deployed in only seconds
to generate an pCT image and bypasses the need for a planning CT, can be
considered clinically acceptable whilst reducing imaging dose and registration
issues. Future work will investigate the accuracy of using this pCT tool
for MRgRT treatments of other anatomies.
Funding:
European Union’s Horizon 2020 research and
innovation programme (No. 880314)