AI-based OAR delineation in brain T1w-MRI: Overcoming Inter- and Intra-observer variability
PO-1890
Abstract
AI-based OAR delineation in brain T1w-MRI: Overcoming Inter- and Intra-observer variability
Authors: Gorkem Gungor1, Guillaume Klausner2, Gokhan Gur3, Ilkay Serbez1, Bilgehan Temur1, Alexandre Caffaro4, Leo Hardy4, Sanmady Kandiban4, Ayoub Oumani4, Basile Bertrand4, Kumar Shreshtha5, Thais Roque6, Banu Atalar3, Nikos Paragios7,4, Enis Ozyar1
1Acibadem MAA University, Maslak Hospital, Department of Radiation Oncology, Istanbul, Turkey; 2Hôpitaux Universitaires de Genève (HUG) , Radiation Oncology, Genève, Switzerland; 3Acibadem MAA University, Maslak Hospital, Department of Radiation Oncology, Istanbul, Turkey; 4TheraPanacea, Research and Development, Paris, France; 5TheraPanacea, AI Research Department, Paris, France; 6TheraPanacea, Clinical and Partnerships Affairs, Paris, France; 7CentraleSupelec, University of Paris-Saclay, Compute Sciences and Applied Mathematics, Gif-sur-Yvette, France
Show Affiliations
Hide Affiliations
Purpose or Objective
Organ-at-risk (OAR) delineation is a key step for radiotherapy treatment
(RT) planning. Manual delineation of OARs is a tedious process, time consuming,
and prone to errors due to intra- and inter-observer variations. The management
of brain tumors typically involves RT planning based on computed tomography
(CT) and magnetic resonance imaging (MRI). MRI is acquired for detailed tumor
localization and delineations of the target and OARs thanks to its excellent
soft-tissue contrast. In this study, we propose to use an automatic artificial intelligence-based
OAR segmentation to support MR-based treatment planning for brain.
Material and Methods
ART-Net®, a CE-marked, FDA-cleared anatomically preserving
deep-learning ensemble architecture for automatic contouring (AC) of OAR,
was retrained on T1w-MRIs following the EPTN 2018 guidelines. For a total of 80
patients, automatic annotations of 25 OARs were performed and compared against
the inter-expert variability between three expert annotators. In addition, 9
(chiasma, encephalon, left cochlea, left cornea, left eye lens, midbrain,
posterior cerebellum, and right lacrimal gland) out of the 25 OARs were
randomly selected and submitted to two independent observers for evaluation.
Experts’ contours used for RT delivery were blended with the ones delineated by
ART-Net® at a 50%-50% ratio. Random blending at the patient level was performed
guaranteeing that, among contours being evaluated per patient and OAR, the
50%-50% split was satisfied. Experts were asked to score ACs and the contours
from clinical experts (CE) as A/acceptable, B/ acceptable after minor
corrections, and C/ not acceptable for clinical use. To avoid any bias, experts
were blind to the origin of the contours (manual or AI).
Results
Average Dice score coefficient (DSC) for ART-Net® on the testing set was
72.6% while that of the inter-expert variability was 69.1%. ART-Net® achieved
mean dice greater than the inter-expert variability for all 25 organs (Tab.1).
Encephalon reported the highest DSC 97.45 ± 0.50, while left vestibular and semi-circular
canals (VSCC) reported the lowest DSC of 52.58 ± 14.55. These organs are
normally not visible on T1Gd sequences, which might explain these results. 4
out of the 9 organs were evaluated as A for 100% of the cases. All 9 OARs achieved
a 100% A+B score for the AI contours. A+B score for the manual delineation from
experts was 100% for all but one organ (left eye lens).
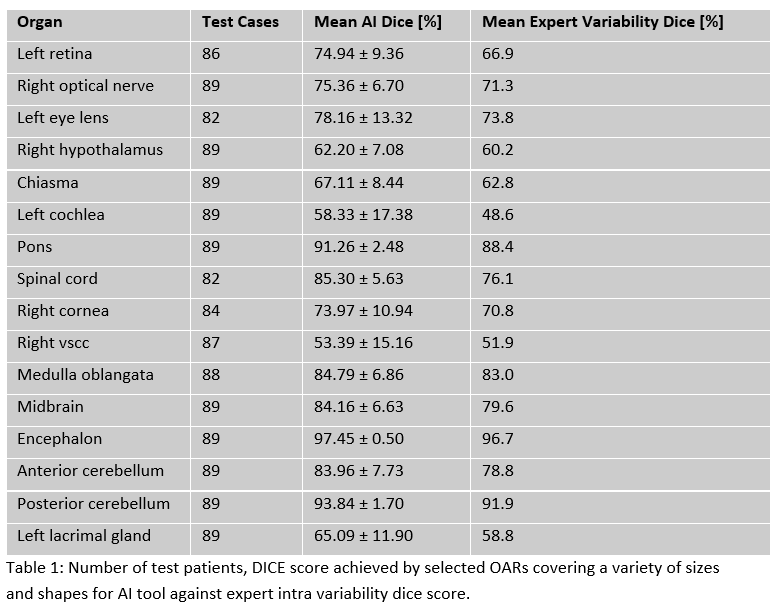
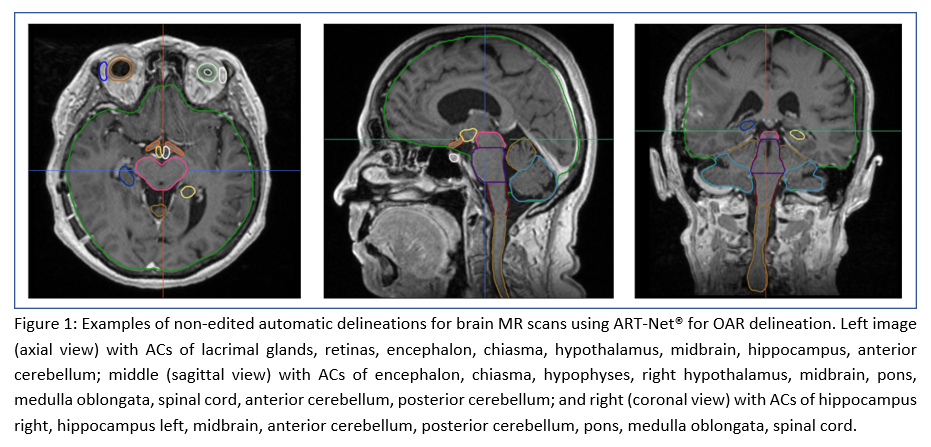
Conclusion
In this study, we investigated the
advantages of using a CE-marked,
FDA-cleared anatomically
preserving deep-learning
ensemble architecture for automatic segmentation
of OARs for brain RT on MRI. This work illustrates the strength of using
ART-Net® for segmentation to support MR-based treatment planning in the brain
as it consistently generates delineations with high clinical acceptability which
can lead to reduction in variability of practice. Future work will include
dosimetric evaluations.