Validation of deep learning auto-segmentation in pelvic organs at risk: a preliminary analysis
PO-1887
Abstract
Validation of deep learning auto-segmentation in pelvic organs at risk: a preliminary analysis
Authors: Domenico Piro1, Marco Marras1, Andrea D'Aviero1, Althea Boschetti1, Claudio Votta1, Alessia Re1, Francesco Catucci1, Davide Cusumano2, Carmela Di Dio1, Sebastiano Menna2, Martina Iezzi3, Flaviovincenzo Quaranta1, Chiara Flore1, Eleonora Sanna1, Danila Piccari1, Gian Carlo Mattiucci2, Vincenzo Valentini2
1Mater Olbia Hospital, Radiation Oncology Unit, Olbia (SS), Italy; 2Fondazione Policlinico Universitario Agostino Gemelli IRCCS, Dipartimento di Diagnostica per Immagini, Radioterapia Oncologica ed Ematologia, Roma, Italy; 3Istituto di Radiologia, Dipartimento di Diagnostica per Immagini, Radioterapia Oncologica ed Ematologia, Roma, Italy
Show Affiliations
Hide Affiliations
Purpose or Objective
Organs at risk (OARs) delineation is a crucial
step of treatment planning workflow.Time consuming and inter-observer
variability are main issues in manual OARs delineation. Deep-learning based
auto-segmentation is a promising strategy to improve OARs contouring in
radiotherapy departments.
A comparison of deep-learning-generated auto-contours
(AC) with manual contours (MC) was
performed by expert Radiation
Oncologists from a single center.
Material and Methods
Planning computed tomography (CT) scans of patients undergoing RT treatments in pelvic region were
considered.
CT scans were processed by a commercial deep learning
auto-segmentation based software to generate AC. Pelvic
protocol was used to perform AC, structure set include ano-rectum,
bladder, bowel bag and femoral heads. Manual Contours of OARs were delineated
by expert radiation oncologists following the same contouring guidelines used by AC software.
The AC and MC were compared
using the Dice Similarity Coefficient (DSC) and 95% Hausdorff distance
transform (DT).
Results
Twenty-three CT scan were included in the analysis; ano-rectum and femoral
heads contours were not compared when considered for Clinical target Volumes in
5 and 2 cases respectively.
DSC and DT results are showed in Fig. 1 and 2.
Minimal differences were showed if we consider DSC of femoral heads (0.93
for both left and right femoral heads and DT 12.42 and 11.68 mm respectively).
The comparison of hollow organs was slightly worse; probably regardless to
differences in analysing density of hollow organs content. Mean DSC and 95% DT for
bladder were 0.88 and 14.31 mm respectively. The comparison of ano-rectum and
bowel-bag delineations resulted in DST of 0.83 and 0.86 respectively, and a DT of 29
mm for ano-rectum and 36.9 for bowel-bag.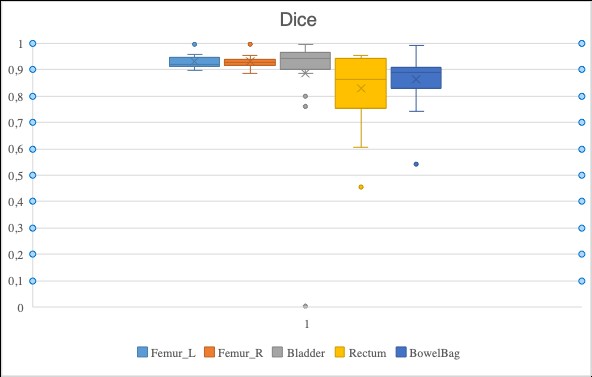
Conclusion
In this
preliminary analysis deep-learning auto-segmentation seems to provide acceptable
pelvic OARs delineations. For less accurate organs, AC could be considered a starting
point for review and manual adjustment. Our results suggest that AC could become a
useful time-saving tool to optimize workload and resources in RT departments. Further
experiences with larger data numbers are needed to confirm its clinically
viability are needed.