Meta-optimization of Radiation Therapy Treatment Plans
PO-1745
Abstract
Meta-optimization of Radiation Therapy Treatment Plans
Authors: Charles Huang1, Yusuke Nomura2, Yong Yang2, Lei Xing2
1Stanford University, Bioengineering, Stanford, USA; 2Stanford University, Radiation Oncology, Stanford, USA
Show Affiliations
Hide Affiliations
Purpose or Objective
Radiation therapy treatment planning is a
time-consuming and labor-intensive process. Typically, planners perform manual, iterative adjustments of
hyperparameters until a clinically acceptable plan has been generated. To automate the treatment planning process,
we propose a meta-optimization framework, called MetaPlanner
(MP).
Material and Methods
The proposed MP algorithm performs optimization of treatment planning hyperparameters using a two-loop optimization approach. In the outer loop, the algorithm uses a derivative-free method (i.e. parallel NelderMead simplex search) to search for weight configurations that
minimize a meta-scoring function. In the inner loop, traditional inverse planning optimization (i.e. fluence map optimization) is performed. Meta-scoring is performed by
constructing a tier list to rank planner preferences and mimic the
clinical decision-making process. The source code for the MP algorithm is publicly available through github (https://github.com/chh105/MetaPlanner).
Results
The proposed
MP method is retrospectively evaluated on two datasets (21 prostate cases and 6
head and neck cases) collected as part of clinical workflow. MP is
applied to both IMRT and VMAT planning and compared to a
baseline of manual VMAT plans. MP in both IMRT and VMAT
scenarios has comparable or better performance than manual
VMAT planning for all evaluated metrics (e.g. dose conformity, dose homogeneity, OAR sparing, etc.).
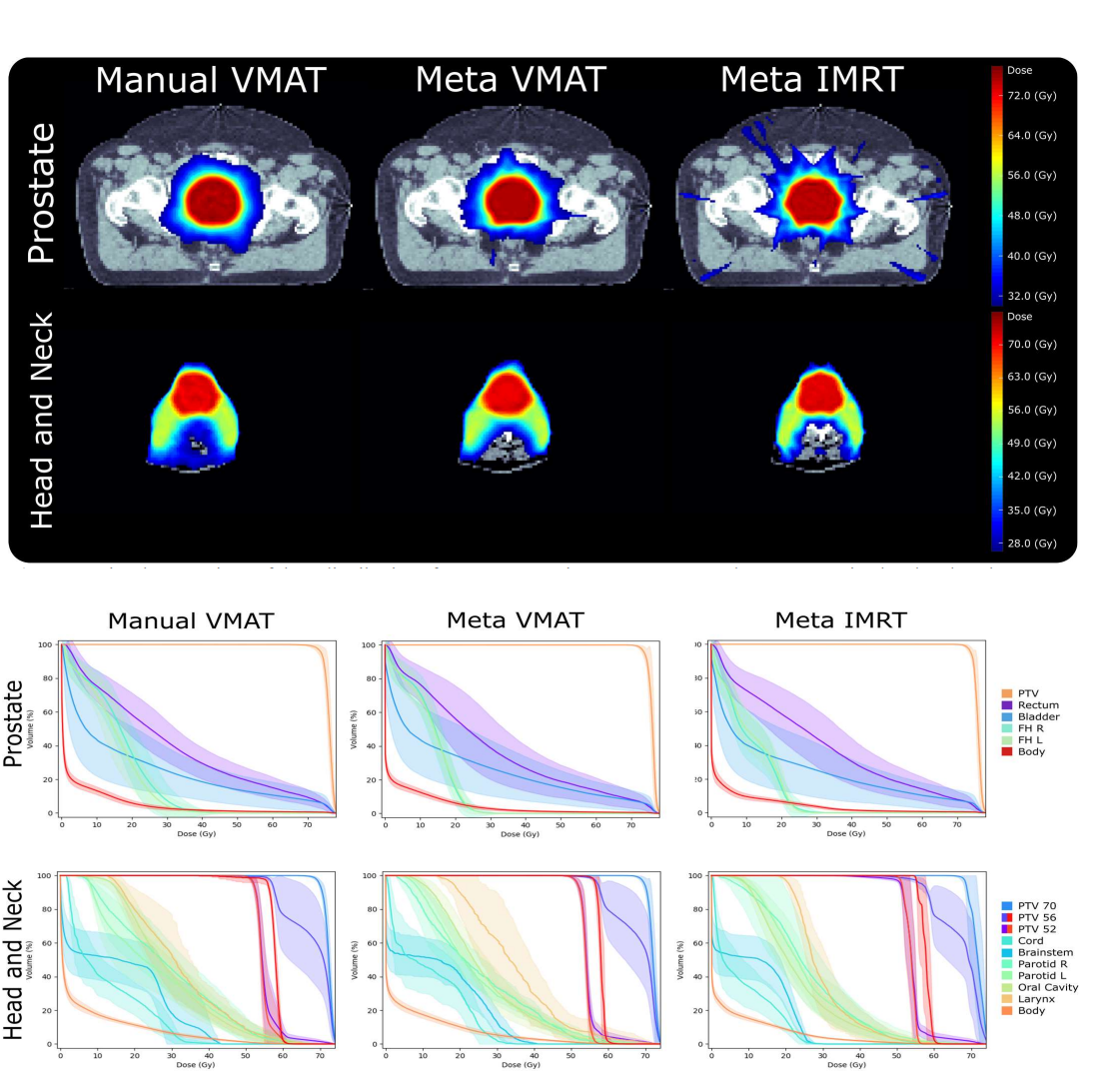
Conclusion
The MP method is a general framework for automated treatment planning with the potential to substantially reduce planning workload while maintaining or improving plan quality.