MRI-Based Synthetic CT Generation for Head and Neck: 2D vs 3D Deep Learning
PO-1686
Abstract
MRI-Based Synthetic CT Generation for Head and Neck: 2D vs 3D Deep Learning
Authors: Behzad Asadi1, Jae Hyuk Choi2, Peter Greer1, James Welsh3, Stephan Chalup2, John Simpson1
1Calvary Mater Newcastle Hospital, Radiation Oncology, Newcastle, Australia; 2The University of Newcastle, School of Information and Physical Sciences, Newcastle, Australia; 3The University of Newcastle, School of Engineering, Newcastle, Australia
Show Affiliations
Hide Affiliations
Purpose or Objective
The problem of MR-only radiation treatment planning is addressed where co-registered synthetic CT (sCT) scans are generated from MR images using a deep learning network. Possible improvements due to spatial correlation among MR slices are investigated by considering a 3D approach and comparing it with a 2D approach.
Material and Methods
A head-and-neck dataset was utilized consisting of images from 148 retrospective patients treated using the standard workflow. For each patient, two MR T1 and T2 dixon axial in-phase sequences, and one CT scan were available all scanned in the treatment position. Bias-field correction, and standardization were performed on MR images. Different sequences were also registered together considering T1 sequences as fixed images. The dataset was randomly split into training and test dataset. Four fifths of the dataset was used as the training dataset to optimize deep learning networks, and one fifth as the test dataset to measure their generalization performance.
First, a 2D UNet was trained where the input to the network is a pair of T1 and T2 slices, and the output is the generated sCT slice. The network was optimized using the mean squared error between generated sCT slices and reference CT slices as the loss function. Next, a 3D UNet was trained where the input is a pair of 3D T1 and T2 slice sets, and the output is the generated 3D sCT slice set. Each set consists of 16 axial slices. In our training dataset, only around 10 percent of patients have slices from the upper part of the chest while all patients have slices from the head area. To compensate for this imbalance problem, the number of samples from the chest area was increased by creating copies of available samples. Online data augmentation was employed to prevent the network from memorizing samples where the network sees a randomly transformed version of samples in each iteration.
Results
Using the 2D UNet, a mean absolute error (MAE) with the mean of 67.5 and standard deviation of 14.3 across patients was achieved on the test dataset. An example of reference CT and generated sCT scans is shown in Fig. 1. Using the 3D UNet, a better MAE with the mean of 65.3 and standard deviation of 14.2 across patients was obtained on the test dataset. By looking at sagittal views, it was found that the 3D approach can reduce the discontinuity error in sCT scans compared to the 2D approach as shown and marked in Fig. 2. This is important for the head-and-neck site as it has complex anatomical variations in superior-inferior direction compared to other sites such as prostate.
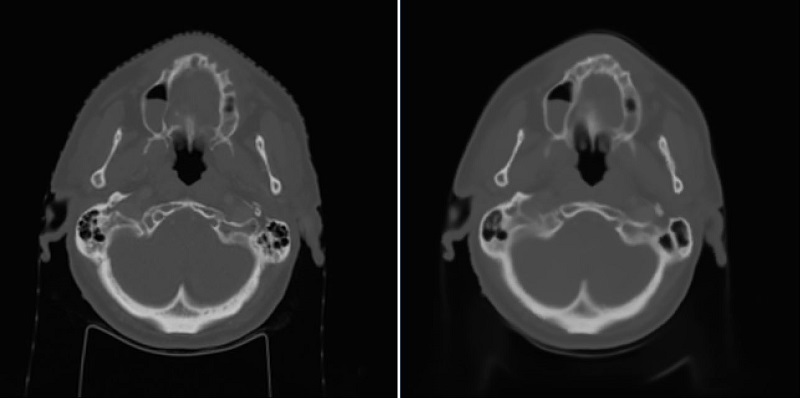
Fig. 1: Left) Reference CT, Right) sCT by the 2D approach.
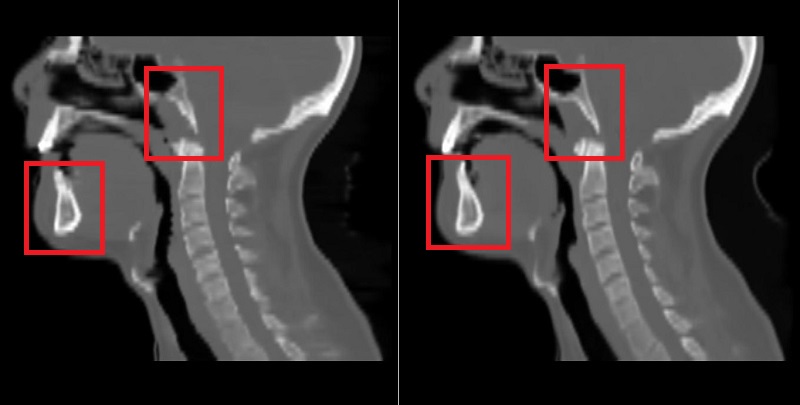
Fig. 2: Left) sCT by the 2D approach, Right) sCT by the 3D approach.
Conclusion
A 3D approach can outperform a 2D approach by reducing the MAE and the discontinuity error in generated sCT scans. This is because the network utilizes spatial correlation among MR slices during the sCT generation process.
Acknowledgement: This work is part of a grant supported by Varian Medical Systems.