Comprehensive evaluation of ProtegeAI Prostate 2.0 auto-segmentation: time-gain and accuracy
PO-1676
Abstract
Comprehensive evaluation of ProtegeAI Prostate 2.0 auto-segmentation: time-gain and accuracy
Authors: Nicolas Jullian1, Zelda Paquier2,3, Manuela Burghelea2,3, Dirk Van Gestel1, Nick Reynaert2,3, Akos Gulyban2,3
1Institut Jules Bordet, Université Libre de Bruxelles (ULB), Radiation Oncology, Brussels, Belgium; 2Institut Jules Bordet, Medical Physics, Brussels, Belgium; 3Université Libre de Bruxelles (ULB), Radiophysics and MRI physics laboratory, Brussels, Belgium
Show Affiliations
Hide Affiliations
Purpose or Objective
The aim of this study was to evaluate the time gain and accuracy of the MIM
ProtegeAI 2.0 auto-segmentation solution (version 7.1.5, MIM software Inc,
Cleveland OH, USA). A second objective was to assess intra-observer variability
and familiarization bias when using auto-segmentation.
Material and Methods
Twenty-five
patients with prostate cancer were included. For each case a planning CT scan (from
vertebrae L1/2 to 3cm below the ischial tuberosity, 3mm slice thickness) was
performed, followed by auto-segmentation using the ProtegeAI Prostate 2.0 model
(AI)
and manual delineation by a single observer (Manual). Femur_L/_R, PenileBulb,
Rectum, SeminalVes, Bladder were evaluated; while another five AI-generated OARs
did not match our institutional template, hence were not evaluated. Time of AI
delineation scoring (AIscor: major/minor/no correction
needed), AI correction (AIcor), total AI (=AIscor+AIcor) and manual
delineation was measured. Time gain was also calculated per individual OAR. Half
of the cohort started with AIscor and AIcor followed by Manual,
while the other half started with Manual, followed by AIscor
and AIcor. For both groups Manual and AIcor were compared separately
to evaluate familiarization bias. For time-gain and bias evaluation t-test at
p<0.05 significance level were used. Dice Similarity Coefficient (DSC), 95% Hausdorff
(HD95) and median surface distance (MSD) were also determined for AI/AIcor,
AI/Manual
and AIcor/Manual
comparisons. AIcor/Manual was used to define intra-observer
variability as both contours were considered clinically acceptable.
Results
A total
of 235 contours were generated by AI (5 min per patient). For 20 patients, AI
failed to generate Kidney_L/_R. Major, minor or no correction was considered in
14%, 72% and 14% of delineations, respectively. Manual took on average
12:25 (min:sec; range:8:21-21:59), AIscor and AIcor 1:55 (r: 1:21-3:32) and 6:18 (r:2:49-14:14), respectively
(figure 1). AI gave up to 13:06 time gain, with an average of 4:12 (p<0.001),
although for two patients AI took more time than Manual
(3:05 and 2:08). Per OAR, the average time gain was 0:42 (r:-0.11-1:45). The
familiarization bias, observed for Manual (p=0.029), was on average
2:25 faster when AI workflow started first, while for AIcor no significant bias
was observed (p=0.168). Good DSC (>0.8) was observed for AI/AIcor,
while HD95 and MSD (figure 2) showed larger discrepancy. For Femur (AI
and AIcor)
vs. Femural Head (Manual) agreement was moderate due to difference in intended
delineation. Intra-observer (AIcor/Manual) variability was
worse for DSC and better for HD95 and MSD compared to AI/AIcor.
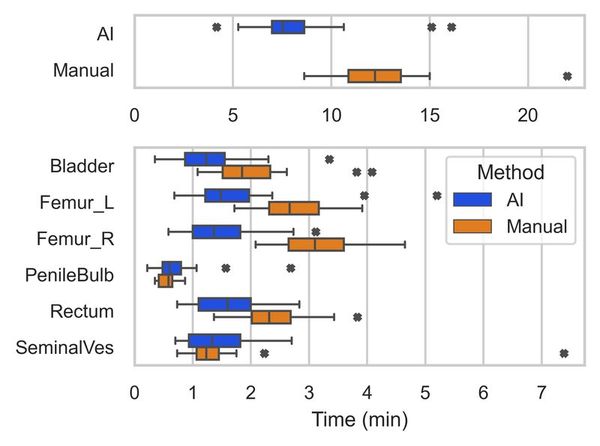
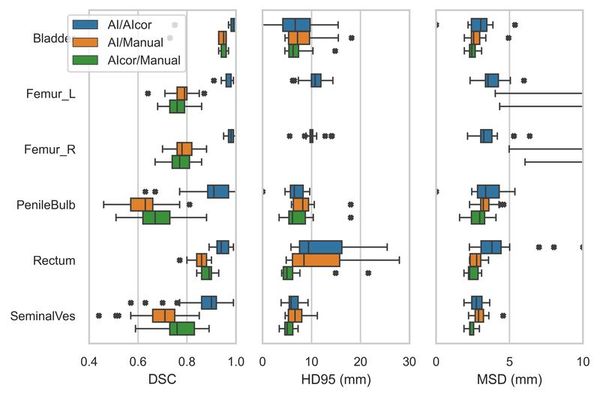
Conclusion
ProtegeAI
Prostate 2.0 auto-segmentation provides on average >4 minutes gain per
patient while requiring only minor corrections. Realistic time gain is likely
higher, as AIscor+AIcor prior manual delineation significantly reduced manual
delineation time. Intraobserver variability remains a substantial source of differences,
especially based on DSC.