Dosimetric evaluation of AI-based synthetic CTs for MRI-only brain radiotherapy
PO-1661
Abstract
Dosimetric evaluation of AI-based synthetic CTs for MRI-only brain radiotherapy
Authors: Cristina Veres1, Kumar Shrestha2, Thais Roque2, Emilie Alvarez-Andres1, Anne Gasnier1, Frédéric Dhermain1, Nikos Paragios2, Eric Deutsch1, Charlotte Robert1
1Gustave Roussy Cancer Campus, Department of radiation oncology, Villejuif, France; 2TheraPanacea, TheraPanacea, Paris, France
Show Affiliations
Hide Affiliations
Purpose or Objective
The adoption of MRI
in support of radiation treatment (RT) planning has increased dramatically. Due
to its excellent soft tissue contrast, MRI is considered standard for target
and some OAR definition in brain oncology. The CT images, with their electron
density (ED) information, are needed for dose calculations in photon RT.
MRI-only radiotherapy eliminates registration errors and reduces patient
discomfort, workload and cost. The aim of this study was to evaluate the
dosimetric accuracy of an innovative self-supervised generative adversarial
neural networks synthetic-CT (sCT) generation from diagnosis MR images for
MRI-only workflow for IMRT of brain gliomas.
Material and Methods
T1w-MRI
and planning CT images were retrospectively collected for 25 patients for
dosimetry evaluation. sCTs were generated using a self-supervised generative
adversarial deep learning (DL) approach, trained on a dataset of 1242 T1w diagnosis
MRI scans and the corresponding CT scans from multiple devices and
manufacturers. The original CT (oCT) images were rigidly registered and
resampled on MR images and the patient immobilization mask cleaned on warped CTs
(wCTs) applying a mask designed from sCTs based on an erosion/dilatation approach.
A comparison between sCTs and wCTs in terms of mean absolute error (MAE) of
Hounsfield Units (HU) in 4 different areas (air, bone, water, and head) was
carried out. The
sCTs and the wCTs were registered on oCTs and the dose matrices were
re-calculated using plan transfer using a commercial collapsed cone algorithm. Calculations
were also performed on oCT, i.e. without immobilization mask cleaning, to
assess the discrimination capabilities of the indices. The absolute differences
in DVH-parameters (D2, D50, D95 and D98) for PTV and (Dmax and Dmean) for OARs were
calculated. Dose distributions were in addition compared with 2%/2mm global and
local gamma index criteria.
Results
The
size of tumors varied between 7 cm3 and 705 cm3 with an
average of 226 cm3. Qualitative results are shown in
Figure 1 and illustrate the crucial role of immobilization mask modeling. Mean
MAE of 67HU+/-10HU, 175HU+/-21HU, 188HU+/-40HU and 30HU+/-3HU were obtained for
the whole head, bone, air and water areas, respectively, in the independent
institutional cohort. The dosimetric results are summarized in Table 1.
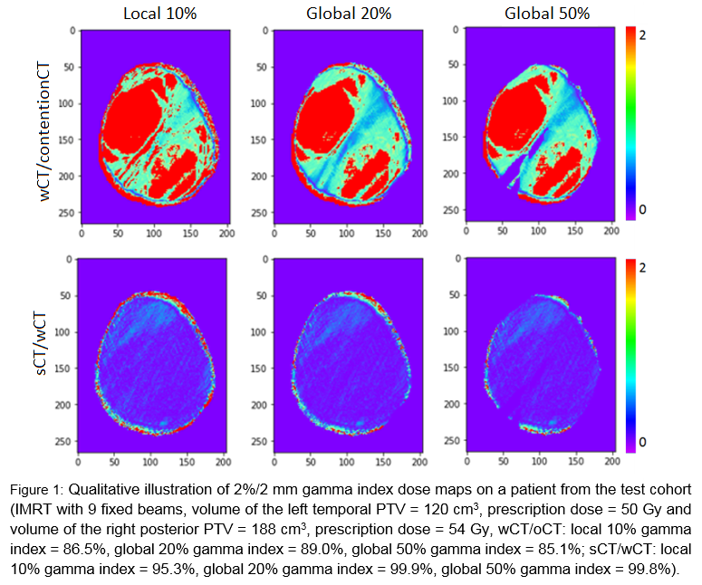
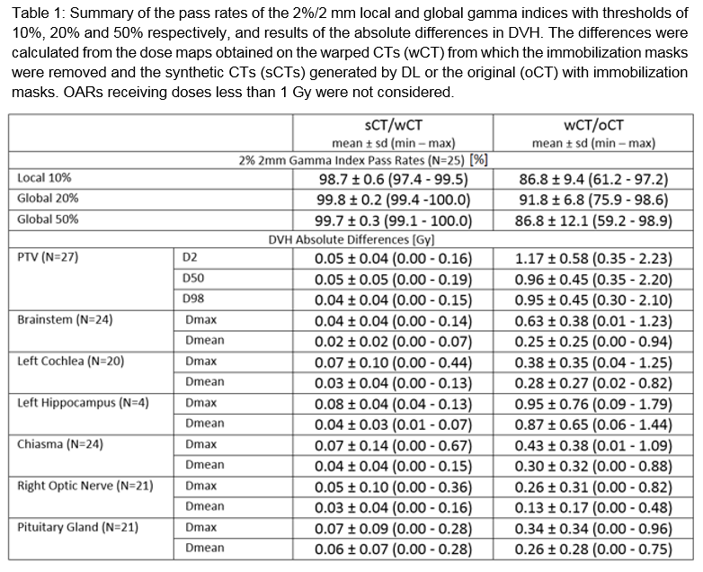
Conclusion
This
work successfully evaluated a self-supervised DL based software for sCT
generation that allows for superior alignment of training data and makes it
possible to train a generative model even with diagnostic MRIs, bypassing the
need for patients to be in treatment position on the MRIs. Dosimetric
differences were minimal and clinically insignificant for both PTVs and OARs. sCT
based MRI-only planning can be feasible to use for RT planning of brain
tumours. Future work will investigate feasibility
of mask immobilization reconstruction and the accuracy of using sCT for daily
CBCT position verification.