A new machine learning-based clinical tool for vertebral level verification and treatment planning
PO-1652
Abstract
A new machine learning-based clinical tool for vertebral level verification and treatment planning
Authors: Tucker Netherton1, Callistus Nguyen1, Carlos Cardenas2, Caroline Chung3, Ann Klopp3, Lauren Colbert3, Dong Joo Rhee1, Christine Peterson4, Rebecca Howell1, Ajay Aggarwal5, Hannah Simonds6, Laurence Court1
1University of Texas MD Anderson Cancer Center, Radiation Physics, Houston, USA; 2University of Alabama Birmingham , Radiation Physics, Birmingham, USA; 3University of Texas MD Anderson Cancer Center, Radiation Oncology, Houston, USA; 4University of Texas MD Anderson Cancer Center, Biostatistics, Houston, USA; 5Guy's and St. Thomas Hospital London, Radiation Oncology, London, United Kingdom; 6Stellenbosch University, Radiation Oncology, Cape Town, South Africa
Show Affiliations
Hide Affiliations
Purpose or Objective
To develop a palliative treatment
planning paradigm that verifies all vertebral bodies and creates a treatment plan
on diagnostic and simulation CT images.
Material and Methods
Vertebrae from any CT and of
any length are labeled (C1-L5) using two intendent deep learning models to mirror
two different experts labeling the spine. Then, a UNet++ architecture was trained,
validation, and tested so that each resulting vertebrae could be contoured using
(n=220 CTs). Features from labeling and from contours were input into a random forest
classifier to predict whether vertebrae were correctly labeled. This classifier
was trained using CBCT, PET-CT, diagnostic, and simulation CT images (n=56 CTs,
752 contours). Autoplans were generated via scripting using auto-verified contours.
Each model was combined into a framework to make a fully-automated treatment planning
clinical tool. Three radiation oncologist scored plan quality on a new cohort of
CT scans (n=60) on a 5-point scale. CTs varied in scan length, presence of
surgical implants, imaging protocol, and metastatic burden.
Results
Accuracy for automatic labeling
was 94% with < 2.2mm error. Mean Dice-Similarity Coefficient was 85.0%(cervical), 90.3%(thoracic),
and 93.7%(lumbar). The random forest classifier predicted mislabeling across various CT
scan types with precision-recall-AUC=0.82. All contouring and
labeling errors (11/11) within treatment regions,
including errors from patient plans (6/6) with atypical
anatomy (e.g. T13, L6) were detected. Radiation oncologists
scored 98% of simulation CT- and 92% of diagnostic
CT-based plans as clinically acceptable or needing minor edits for
patients with typical anatomy. End-to-end treatment planning time of the clinical
tool was less than 8 minutes on average.
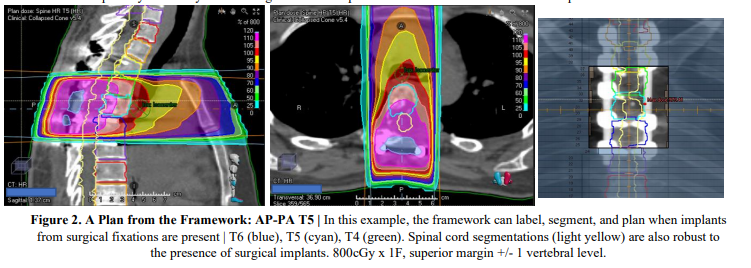
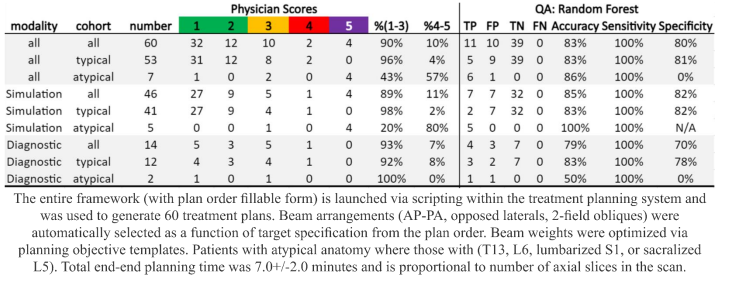
Conclusion
This novel method to automatically verify,
contour, and plan palliative spine treatments is efficient, effective,
and safe across various CT scan types.