Virtual brain MRI missing sequences creation using machine learning generative adversarial networks
Edoardo Giacomello,
Italy
PO-1641
Abstract
Virtual brain MRI missing sequences creation using machine learning generative adversarial networks
Authors: Edoardo Giacomello1, Damiano Dei2,3, Nicola Lambri2, Pietro Mancosu4, Daniele Loiacono1
1Politecnico di Milano, Dipartimento di Elettronica, Informazione e Bioingegneria, Milano, Italy; 2Humanitas University, Department of Biomedical Sciences, Pieve Emanuele, Milano, Italy; 3IRCCS Humanitas Research Hospital, Department of Radiotherapy and Radiosurgery, Rozzano-Milano, Italy; 4IRCCS Humanitas Research Hospital, Medical Physics Unit, Radiotherapy and Radiosurgery Department, Rozzano-Milano, Italy
Show Affiliations
Hide Affiliations
Purpose or Objective
Deep learning (DL) has been successfully applied to segment regions
of interest (ROI) from magnetic resonance imaging (MRI). These
segmentation models are often designed to work with multi-modal images
as input to exploit the most from the different characteristics among
the various sequences and generate better segmentations. However, when
applying a segmentation model to a new patient, all the sequences
required by the model might not be available. This can happen, for
example, in a multicenter context, use of different scanners,
specificity of the clinical protocols, prohibitive scan times, or
allergies to contrast media. Generative Adversarial Networks (GANs) is a
promising learning paradigm in DL that has proven very effective in
solving image-to-image translation tasks. In this study, we propose a
way to virtually generate missing sequences from existing ones using
GAN.
Material and Methods
We trained three GANs - pix2pix (P2P), MI-pix2pix (MI-P2P), and
MI-GAN - for generating missing MRI sequences from the ones available.
The models have been trained on the 2015 Multimodal Brain Tumor
Segmentation Challenge (BRATS2015) dataset, which includes the MRIs of
274 patients affected by Glioma. For each patient, BRATS2015 provides
four different MRI modalities - T1, T1 with contrast, T2, and T2flair -
and the segmented volume of the tumor. The dataset was split into three
sets for (i) training (80% of the samples), (ii) validation (10% of the
samples), and (iii) testing (10% of the samples). We designed a set of
quantitative metrics to assess the quality of the generated images with
respect to the original ones. In addition, we also compared the
performance of segmentation models when generated MRIs are provided as
input instead of the real ones.
Results
Our results show that the virtual generated images are rather
accurate and realistic (see some examples in Figure 1). Our findings
(Table 1) suggest that multi-input models (MI-P2P and MI-GAN) perform
generally better than single-input ones (P2P) and MI-P2P generally
achieves the best performances overall. In addition, for all the models
trained, the generated images are much more similar to the real ones
with respect to the source images (reported as a baseline in Table 1).
Finally, our results also showed that generated images can be used to
solve segmentation tasks with an accuracy loss that is between 6% and 14% with respect to using the real images.
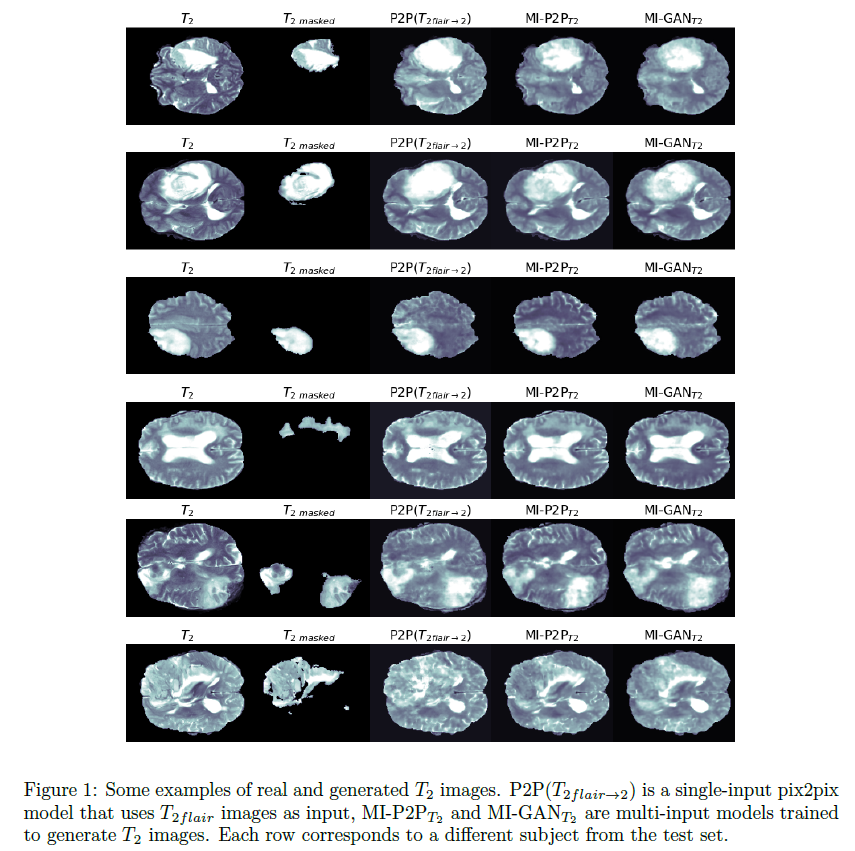
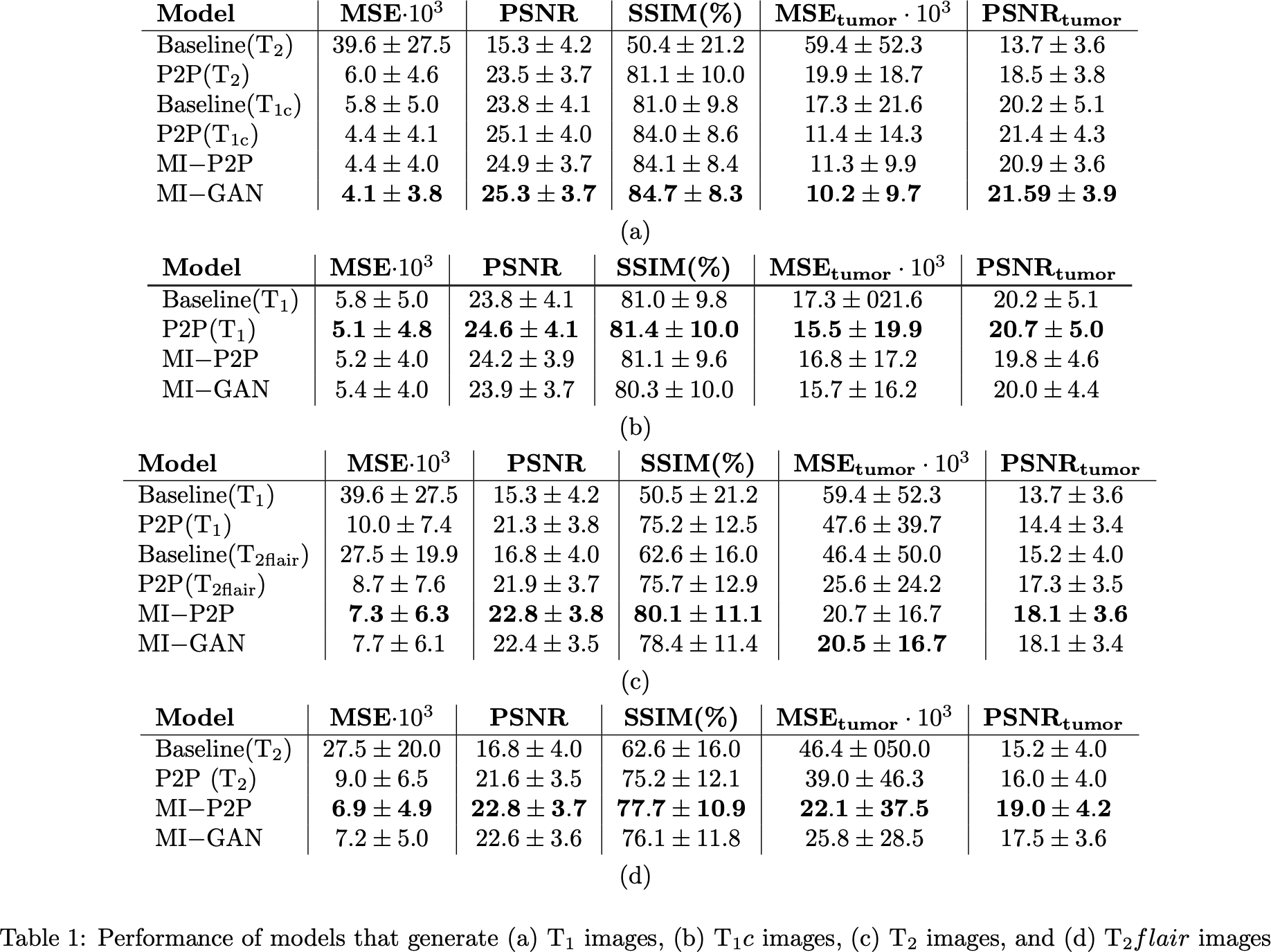
Conclusion
Both qualitative and quantitative results are encouraging and suggest
that GANs are a viable approach to deal with missing modalities when
applying multi-modal segmentation models.