Multiparametric optimization of MR imaging sequences for MR guided radiotherapy
Hafiz Muhammad Fahad,
Germany
PO-1638
Abstract
Multiparametric optimization of MR imaging sequences for MR guided radiotherapy
Authors: Hafiz Muhammad Fahad1,2,3, Stefan Dorsch1,3, Moritz Zaiß4,5, Christin Peter Karger1,3
1German Cancer Research Center DKFZ, Medical Physics in Radiation Oncology, Heidelberg, Germany; 2University of Heidelberg, Faculty of Medicine, Heidelberg, Germany; 3National Center for Radiation Research in Oncology, Heidelberg Institute for Radiation Oncology HIRO, Heidelberg, Germany; 4Friedrich-Alexander Universität Erlangen-Nürnberg (FAU), Neuroradiology, University Clinic Erlangen, Erlangen, Germany; 5Magnetic Resonance Center, Max- Planck Institute for Biological Cyberrnetics, Tübingen, Germany
Show Affiliations
Hide Affiliations
Purpose or Objective
Magnetic
Resonance Imaging (MRI) is being routinely used for treatment planning in MR-guided
radiotherapy, however, the sequences available in MR-Linacs may not be perfectly
optimized in terms of contrast and noise, which can facilitate
tumor and organ-at-risk delineation, registration and synthetic CT calculation.
These parameters can be optimized by a variety of MR sequence parameter sets
(SPS), which directly affect the image quality in terms of signal-(SNR) or contrast-to-noise
ratio (CNR). Depending on the sequence and clinical objective, these SPS can
include up to 30 individual parameters. This work aims to
develop a software tool for the optimization of SNR and CNR in MRI sequences
based on the applied SPS. Here we present the preliminary results of the
evaluation of two different regression techniques for the SNR and CNR
prediction.
Material and Methods
Initially,
two different models to predict the quality parameters (SNR/CNR), depending on
the applied SPS, were investigated and trained. Training data sets were
acquired at a 1.5 T MRI (Aera, Siemens) with a dedicated phantom with in-house
fabricated anthropomorphic contrast inserts of different concentration of
agarose and Ni-DTPA. Measurements were performed with a turbo spin echo
sequence with spatial resolution of 0.4 x 0.4 x 5 mm³, Bandwidth 186 Hz/Pixel
and 4 different, varying parameters (repetition time (TR), echo time (TE),
turbo factor (TF) and flip angle (FA)) to generate a total of 1114 different
SPS-combinations. The models used for regression were a deep learning (DL)
based method with five hidden layers with Relu activation function and one
input and output layer, and a generalized additive model (GAM) based on spline
functions. The models were evaluated on training (90% of
the total data set) and test datasets (10% of the total data set) with two
different standard loss functions for mean absolute error (MAE) and mean square
error (MSE).
Results
The comparison of
the two different models (Figure 1, Table 1) shows that the DL based model yields a higher accuracy (MSE and
MAE) compared to the GAM for the test data. While the GAM performs well on
smaller data sets and the training data, the DL-based model outperforms the GAM
for larger training data sets and the subsequent application on the test data
set.
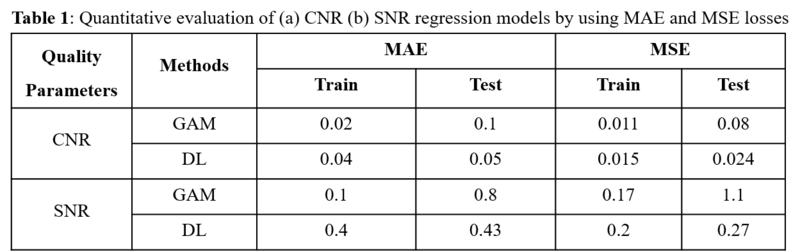
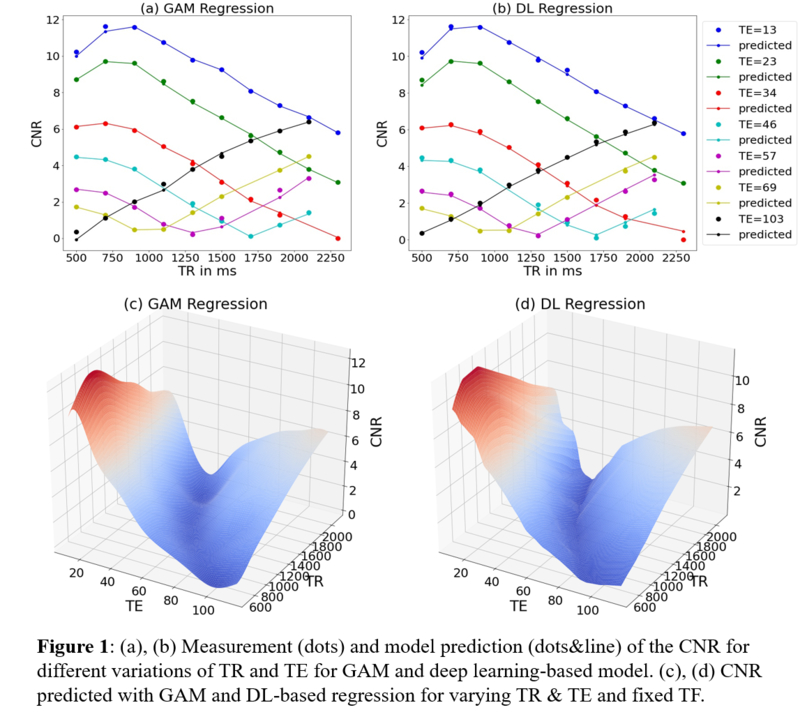
Conclusion
As
a first step towards the development of an optimization tool for MR sequences,
the DL-based model maintained a higher prediction accuracy
for both SNR and CNR in the validation data set. The next step is
to develop and implement multi-objective optimization methods with regards to
SNR and CNR based regression.