Influence of training data variability on deep learning dose prediction robustness for MR-guided RT
PO-1637
Abstract
Influence of training data variability on deep learning dose prediction robustness for MR-guided RT
Authors: Marcel Nachbar1, Simon Gutwein1, Moritz Schneider1, Daniel Zips2, Christian Baumgartner3, Daniela Thorwarth1
1University Hospital and Medical Faculty, Eberhard Karls University Tübingen, Section for Biomedical Physics, Department of Radiation Oncology, Tübingen, Germany; 2University Hospital and Medical Faculty, Eberhard Karls University Tübingen, Department of Radiation Oncology, Tübingen, Germany; 3Eberhard Karls University Tübingen, Cluster of Excellence “Machine Learning”, Tübingen, Germany
Show Affiliations
Hide Affiliations
Purpose or Objective
Deep learning
(DL) based dose predictions are promising for real-time dose calculation and
open thus new possibilities towards adaptive radiotherapy. However, the
accuracy of pretrained DL models remains unclear if conditions between training
data and applied data change. Different conditions may include tumor entity,
source-to-surface distance (SSD), field size and shape, gantry angle and tissue
density. In this work, we therefore developed a DL dose prediction model and
investigated the robustness and risks of dose prediction on unseen data.
Material and Methods
A DL framework was developed to predict dose distributions based
on patient CT and radiation field information. Two training datasets were
defined based on clinical MR-Linac treatment plans of (A) 40 primary prostate
cancer patients and (B) 40 patients with either prostate, head & neck, mamma
or liver cancers. Both training sets corresponded to ~2000 individual segments
from different angles. Gold standard dose distributions, used as target for
model training and testing, were obtained by segment-wise Monte Carlo (MC) dose
simulation using a dedicated EGSnrc MR-Linac model [1]. The training datasets
were used to train two separate 3D U-Net for dose prediction. For
evaluation, both trained models were applied to data representing three
different conditions: (1) A set of 5 unseen prostate plans, (2) 5 head &
neck, mamma and liver cancer plans each and (3) 15 lymph-node plans, for which
the conditions were unseen by both models. The DL dose predictions were
compared against gold standard using gamma analysis (3mm/3%, 40% cutoff) and
evaluated by Wilcoxon signed-rank test.
Results
Both DL models were successfully trained and allowed for segment-wise
dose prediction (fig. 1).
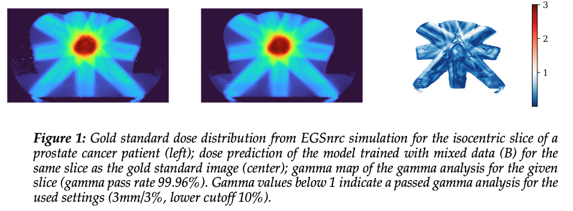
Training dataset (A) compared against (1) presented with a median
[range] pass rate of 98.0% [85.3-100%], whereas for the changed test sets it
decreased to 72.4% [53.8-91.6%] (2) and 85.9% [63.8-98.2%] (3) (fig. 2).
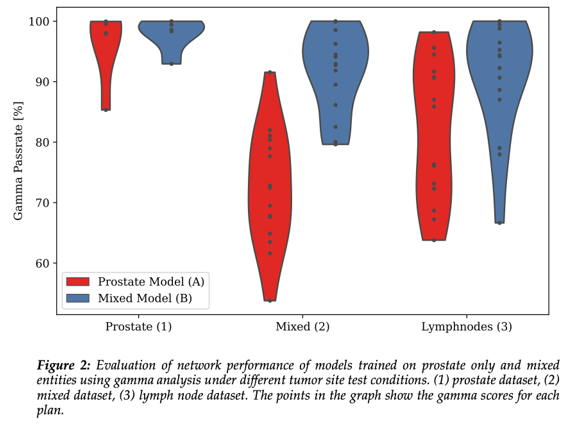
In comparison
the mixed model (B) applied to the test datasets (1), (2) and (3) yielded
median gamma pass rates of 98.6%
[92.9-100%], 92.9% [79.6-100%] and 94.2% [66.6-100%], respectively, resulting in
an improvement of 0.6% (p=n.s.), 20.5% (p<0.001) and 8.3% (p=0.001).
Conclusion
This
study showed that accuracy of DL dose predictions strongly depends on the
conditions represented in the training dataset. While for seen conditions the
DL model predicted dose distributions correctly, unseen conditions may pose a
risk. However, with increasing diversity within the training dataset, even
unseen conditions might be better predictable. Therefore, potential next steps
are to increase diversity within the training data by deviating from clinically
used segments and defining artificial segments to increase robustness.
References:
[1]
Friedel M et al. Med Phys 2019.
Funding:
German Research Council (DFG ZI 736/2-1)