DL-based OAR delineation for Head and Neck Radiotherapy: accuracy versus computational resources
PO-1632
Abstract
DL-based OAR delineation for Head and Neck Radiotherapy: accuracy versus computational resources
Authors: Lucía Cubero1, Javier Serrano2, Felipe A. Calvo2, Antoine Simon3, Joël Castelli3, Renaud De Crevoisier3, Óscar Acosta3, Javier Pascau1
1Universidad Carlos III de Madrid, Bioengineering and Aeroespace Engineering - IGT, Madrid, Spain; 2Clínica Universidad de Navarra, Departamento de Oncología Radioterápica, Madrid, Spain; 3Université de Rennes I, CLCC Eugene Marquis, INSERM, LTSI-UMR 1099, Rennes, France
Show Affiliations
Hide Affiliations
Purpose or Objective
Contouring
the organs at risk (OAR) accurately in head and neck (HN) radiation therapy
planning is crucial for reducing treatment-induced toxicity. These delineations
are time and labor-consuming and often biased by inter and intra-observer
variability. Automatic deep-learning (DL) based segmentation has proven to
overcome the limitations of manual delineation, yielding more robust,
patient-specific contours faster. Nonetheless, these algorithms have not been
integrated into the radiotherapy workflow yet, mainly constrained by the need
for extensive computational resources and technical experience. This study aims
to compare two different DL algorithms and assess this technology's potential
in HN radiotherapy.
Material and Methods
45 CT images from HN cancer patients with
manually segmented OAR (brainstem, cord, eyes and parotids) were split into
training (n = 35) and test (n = 10) sets. Two different fully convolutional
neural networks were trained to segment the 4 OAR. On the one hand, the
nnU-Net, a method that has shown great accuracy in anatomical delineation by
automatic hyperparameter configuration and 5-fold cross-validation. This
complex architecture leads, however, to long training and inference times. On
the other hand, a single-class DenseVNet was trained for each OAR, using as
input a bounding box built from a coarse mask obtained with a multiclass
3DUnet. This network presents certain architectural advantages that result in
shorter training and inference. Both algorithms were evaluated in the test set
by computing the Dice Score Coefficient (DSC) and Average Surface Distance
(ASD).
Results
Figures
1 and 2 depict the evaluation of both DL algorithms on the test set. nnU-Net
achieved slightly more accurate results for every OAR but required over 180
hours for training and 5 minutes for inference, both in an Nvidia RTX 8000 GPU.
Instead, each instance of the DenseVNet was trained in approximately 1.5 hours
on the same GPU, a total of 6 hours for all OAR, whereas the inference drops to
around 70 seconds on CPU. Moreover, DenseVNet allows for retraining one class
or introducing a new OAR independently, while nnU-Net must be retrained
entirely.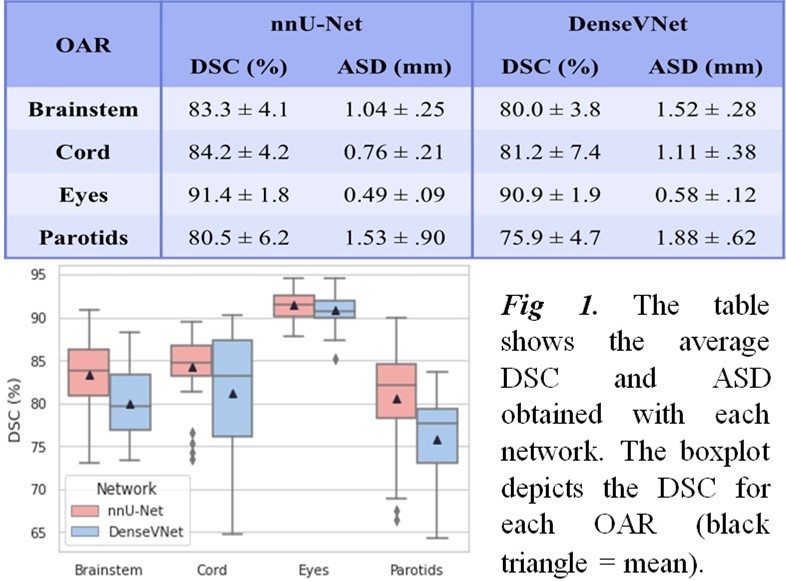
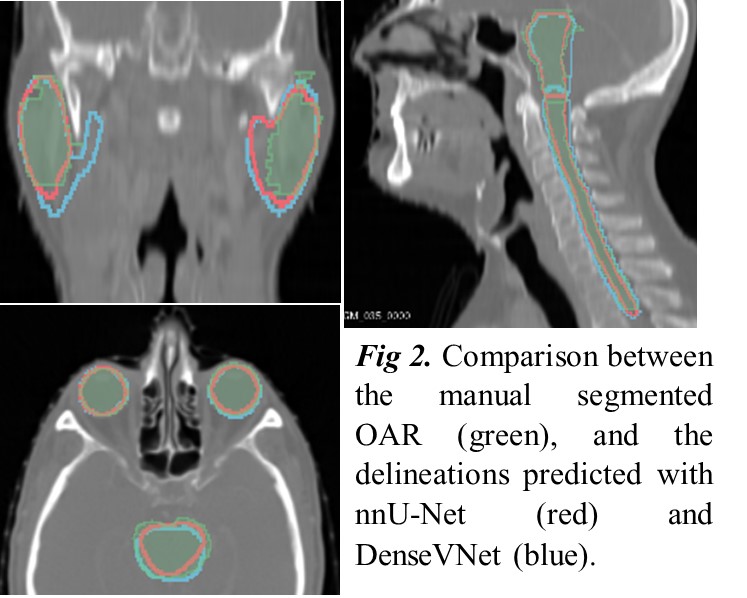
Conclusion
We
present the advantages and downsides of two DL algorithms for OAR segmentation
in HN CT images. nnU-Net performed slightly better for every OAR, yet this
superiority is only remarkable for the parotids. Training and inference times
were meaningfully longer compared to DenseVNet. The aim of DL technologies
should be to shrink the time spent in manual OAR delineation by providing fast
but good enough segmentations still flexible to be modified by an expert
without relying on too extensive computational resources. To optimize the
potential of DL techniques in the radiotherapy field, the weight between
accuracy and computational resources must be therefore carefully evaluated. It
is likely more efficient to train and update periodically with new data a
simpler network such as DenseVNet than to rely upon complex methods as nnU-Net.