An efficient training approach for brain paediatrics synthetic CT generation for protontherapy
Francois de Kermenguy,
France
PO-1621
Abstract
An efficient training approach for brain paediatrics synthetic CT generation for protontherapy
Authors: François de Kermenguy1, Emilie Alvarez Andres1, Ludovic De Marzi2, Lucas Fidon3, Alexandre Carré1, Stéphanie Bolle4, Nikos Paragios3, Eric Deutsch1, Samy Ammari4, Charlotte Robert1
1Gustave Roussy, UMR 1030 Radiothérapie Moléculaire et Innovation Thérapeutique, Villejuif, France; 2Institut Curie, Proton Therapy Centre, Orsay, France; 3TheraPanacea, Research department, Paris, France; 4Gustave Roussy, Department of radiotherapy, Villejuif, France
Show Affiliations
Hide Affiliations
Purpose or Objective
The
increasing use of MRI in radiotherapy workflow has led to the development of "MRI-only" treatment planning methods, especially based on synthetic CT generation (sCT). Deep learning algorithms are the most attractive methods
today for generating sCTs. However, the use of these algorithms requires a
large amount of data, which can be critical in the case of paediatric patients,
where cohorts are often small even when gathered from several centres and
imagers. Thus, this study aims to compare four training methods based on a 3D
HighResNet deep neural network architecture to generate sCTs for paediatric
patients with brain tumours treated with protontherapy. The impact of using a
learning strategy based on scans converted to relative stopping power (RSP) to
avoid imager dependency while increasing cohort size was also studied.
Material and Methods
A cohort of
394 adult patients including CT/MRI brain pairs (199 T1, 195 T1Gd) and a cohort
of 198 paediatric patients including CT/MRI brain pairs (64 T1, 134 T1Gd) were
used to train, validate and test a 3D HighResNet neural network. Pre-processing
was applied to the images (N4 bias field correction, CT to MRI rigid
registration, Z-score normalisation, intensity-clip, volume resampling). Except for
method (1_HU), scan Hounsfield Units (HU) from 3 different devices set at 2
different high voltages (120 kVp and 135 kVp) were converted to RSP using
stoechiometric calibration curves. Four methods of training and validation of
the network were then compared: (1) paediatric-only (1_RSP and 1_HU), (2)
adult-only, (3) mixed adult and paediatric, and (4) transfer learning with
pre-training on adult patients before optimizing weights on the paediatric
patients. The paediatric test cohort remained unchanged among the different
methods and included 40 children. The Mean Absolute Error (MAE) was used to
evaluate sCTs and as loss function of the network. Early stopping on the
validation set was used as stopping criterion. Wilcoxon tests were performed to
assess the significance of the observed differences with a threshold value of 5%.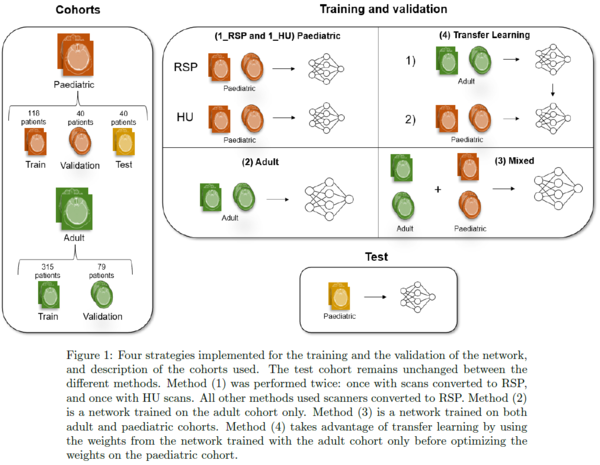
Results
The average
MAE within heads were respectively equal to 107 ± 20 HU, 143 ± 20 HU, 106 ± 19 HU
and 102 ± 19 HU for (1_RSP), (2), (3) and (4). Training with RSP rather than HU in method (1) showed an improvement in average MAE within heads from 121 ± 22 HU
to 107 ± 20 HU. Wilcoxon tests showed that these differences were significant (p<0.0001),
except between methods (1_RSP) and (3) (p >0.25).
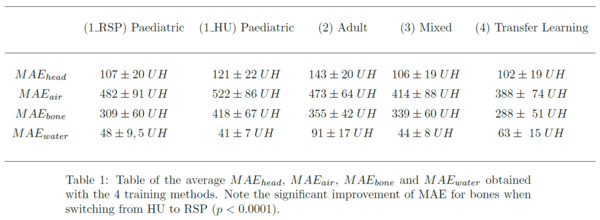
Conclusion
Our
analysis confirms the difficulty of generating paediatric sCTs directly from an
adult model. The transfer learning method combined with a transformation of the
scans into RSPs proposed in this study is an effective strategy to overcome the
lack of patients in paediatric cohorts, and is extendable to adult patients.
Dosimetric differences resulting from the different strategies have to be
quantified in a near future.