Evaluation of deep learning-based OAR segmentation in paediatric radiotherapy settings
Adam Szmul,
United Kingdom
PO-1616
Abstract
Evaluation of deep learning-based OAR segmentation in paediatric radiotherapy settings
Authors: Isabel Silva1,2, Adam Szmul1, Jessica Cantwell3, Pei Lim3, Derek D’Souza3, Syed Moinuddin3, Victor Alves2, Jennifer Gains3, Catarina Veiga1
1University College London, Department of Medical Physics and Biomedical Engineering, London, United Kingdom; 2University of Minho, Centro Algoritmi, Braga, Portugal; 3University College London Hospitals NHS Foundation Trust, Radiotherapy, London, United Kingdom
Show Affiliations
Hide Affiliations
Purpose or Objective
RT treatment of childhood cancer may lead to a plethora of side-effects in later life like second cancers, which may occur outside the primary radiation field. Comprehensive OAR segmentation enables accurate record of delivered doses for long-term studies. However, most OARs are not segmented routinely since they are not used for treatment optimisation and there is a lack of paediatric whole-body autosegmentation tools. In this work we investigate the feasibility of deep-learning segmentation (DLS) to facilitate comprehensive paediatric OAR delineation for follow-up studies of RT side-effects. We compare DLS performance to an in-house multi-atlas segmentation (MAS) algorithm.
Material and Methods
CT scans from 50 craniospinal irradiation patients (2 to 16 years old) and five manual segmentations (bladder, heart, liver, lungs and pancreas) were used to generate an automated DLS method using the open-source MONAI. The dataset was split into training, validation and test sets (64/16/20%). Five patch-based (96×96×96) 3D UNet based networks were trained independently for each organ. The networks had 4 down-sampling stages (with 256, 128, 64, 32, 16 channels at each stage respectively), two residual units, PReLU activation and batch normalisation. Before being presented to the network, the CTs were resampled to a fixed spatial resolution ((1.5, 1.5, 2.0) mm) and intensities were clipped (range variable per organ). Data augmentation including rotations, gaussian noise, contrast adjustments, elastic transformations was applied to 10% of the training set, and networks were trained for 200 epochs with Adam optimizer. Sliding window inference was performed using the best network model (based on the Dice scores on validation set). The output segmentations consisted of the largest connected component (two largest for lungs). The performance of DLS was compared with in-house MAS, where NiftyReg was used to align each unseen test case with the atlases (training and validation sets). Final labels were generated by majority fusion and post-processing. The performance of all segmentations was assessed with the Dice Similarity Coefficient (DSC) and Hausdorff Distance (HD), using as ground-truth the manual segmentations.
Results
Tab.1 shows the quantitative metrics on all organs for DLS and MAS. DLS was generally comparable or superior to MAS in the testing dataset (Fig.1), with the largest improvements observed in the bladder. The pancreas had the poorest segmentation performance for both methods, likely due to poor soft tissue contrast and high intrasubject variability in shape and position. DLS segmentation performance has only slightly decreased on the validation and testing datasets compared to the training.
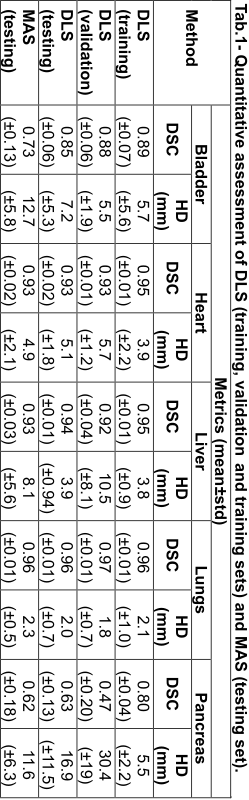
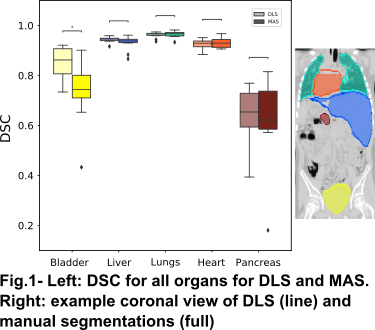
Conclusion
DLS was shown to be promising to segment several OARs in paediatric settings, achieving comparable or superior performance on a relatively small dataset compared to MAS. Further work is needed to optimise network hyperparameters to each organ and investigate performance on other OARs.