CNN-based multi-task learning for tumor segmentation and T-Stage classification in NPC MRI
PO-1612
Abstract
CNN-based multi-task learning for tumor segmentation and T-Stage classification in NPC MRI
1Fudan University Shanghai Cancer Center, Department of Radiation Oncology, Shanghai, China
Show Affiliations
Hide Affiliations
Purpose or Objective
Automatic tumor delineation has been one of the most popular topics in deep learning. T-Stage is determined by tumor size and tumor location, classification of which can help physicians to make treatment decision and predict patients’ prognosis. In recent years, multi-task deep learning has been widely used in many fields. It may get better results by integrating different types but related information. As far as we know, no attempt has been made to combine tumor segmentation and T-Stage classification. In this study, we aimed to find a CNN-based multi-task architecture to obtain results for both tumor segmentation and T-Stage classification.
Material and Methods
Six-hundred-forty-four patients were enrolled in this study, each of which had 3 MR images with T1-weighted, T2-weighted, and contrast-enhanced T1-weighted sequence. The T-Stage (T0:181, T1:76, T2:140, T3:115, T4:132) was extracted from EMR system and reviewed by physician. Tumors were manually contoured on MR images. MR images were input into a supervised CNN to output both T-Stage classification and tumor segmentation. We analyzed three types of CNN architectures: DeepLab (figure 1(a)), Trival Unet (figure 1(b)) and Modified Unet (figure 1(c)). The first one was based on DeepLab V3 and the last two were based on the Unet. The difference between Trival Unet and Modified Unet was that in order to strengthen the connection between T-Stage classification and tumor segmenation, we feedback the prediction results of T-Stage classification to tumor segmenation in Modified Unet. Performance of CNN architecture was assessed by Dice coefficient (DSC) for segmentation task and accuracy for T-Stage classification.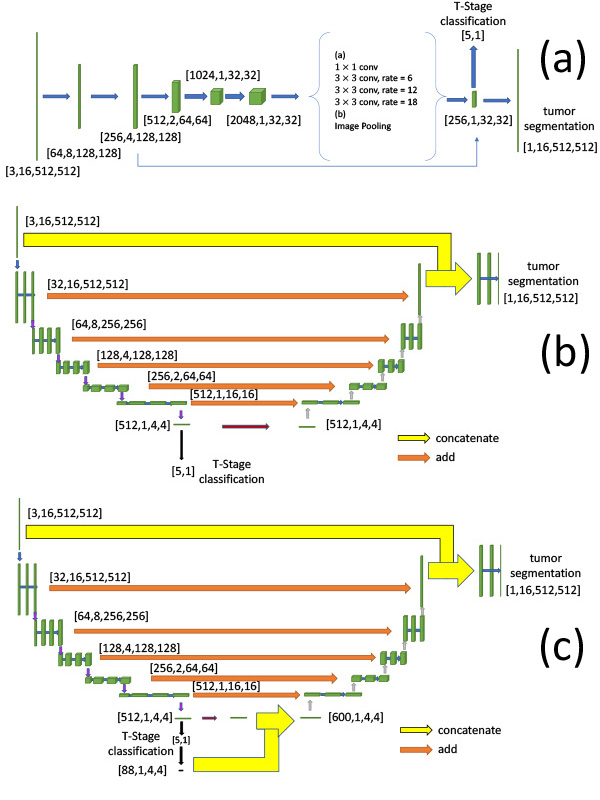
Figure 1. Structures of different multi-task learning architectures
Results
For tumor segmentation, Trival Unet obtained the best result (DSC=0.800), result of Modified Unet was close to the former (DSC=0.791), and DeepLab performed the worst (DSC=0.647). For T-Stage classification, Modified Unet obtained the best result (Accuracy=85.7%), Trival Unet was second (Accuracy=81.4%), and DeepLab still performed the worst (Accuracy=72.0%). In order to know whether multi-task method got better results, we investigated the performance of both tasks in single task situation. For tumor segmentation, single-task Unet performed the best over all the trials, of which DSC was 0.807, 0.007 larger than Trival Unet’s. For T-Stage classification, we used Resnet for training. It performed poorly (Accuracy=58.6%).
Conclusion
In this study, we compared multi-task performance of different CNN architectures: DeepLab, Trival Unet and Modified Unet. Our results showed that T-Stage classification was a difficult task for CNN. Such difficulty contributed to the unusual result: tumor segmentation did not perform better in multi-task than in single-task situation. Despite the difficulty, multi-task method obtained considerable results. Thus, using multi-task method to solve tasks which may be difficult for general single task CNN method is worth considering.