Generating synthetic hypoxia images from FDG-PET using Generative Adversarial Networks (GANs)
PO-1609
Abstract
Generating synthetic hypoxia images from FDG-PET using Generative Adversarial Networks (GANs)
Authors: Alberto Traverso1, Chinmay Rao1, Alexia Briassouli2, Andre Dekker1, Dirk De Ruysscher1, Wouter van Elmpt1
1Maastro Clinic, Department of Radiotherapy, Maastricht, The Netherlands; 2Maastricht University, Department of Knowledge Engineering , Maastricht, The Netherlands
Show Affiliations
Hide Affiliations
Purpose or Objective
Hypoxia imaging can provide important information on tumour biology such as radio resistance. However, hypoxia imaging based on HX4-PET (Positron Emission Tomography) is not routinely acquired because of its higher costs and acquisition times compared to standard FDG-PET.
We used deep learning models based on GANs to synthesise HX4-PET images from FDG-PET and Compute Tomography (CT) scans.
Material and Methods
We applied image translation GANs to synthesise HX4-PET images from FDG-PET and panning CT scans of 34 non small cell lung cancer patients from two clinical trials: the PET-Boost trial (registration number NCT01024829, n = 15) and the Nitroglycerin trial (registration number NCT01210378, n=19). The original HX4-PET images in the first cohort were used for the development of the models; the second cohort for testing.
We explored both paired and unpaired translation approaches. We used the paired Pix2Pix, which is state-of-the art, as the reference method. We introduced unpaired translation with CycleGANs. Since naively applying the default CycleGAN system to our use case is a flawed strategy because the invertibility assumption of CycleGAN is seriously violated here, we proposed a design modification to CycleGAN training for circumventing this issue.
Image pre-processing included image-registration, SUV standardisation (Figure 1A), and cropping/resampling (Figure1B).
We performed an extensive evaluation of the GANs by first testing on a simulated translation task, followed by comprehensively evaluating on the above-mentioned datasets. We, additionally performed a set of clinically relevant downstream tasks (e.g. hypoxia score in the tumour region) on the synthetic HX4-PET images to determine their clinical value.
Quantitative analysis included a variety of image similar similarity metrics, and an additional visual inspection of the images to identify artificially generated artefacts.
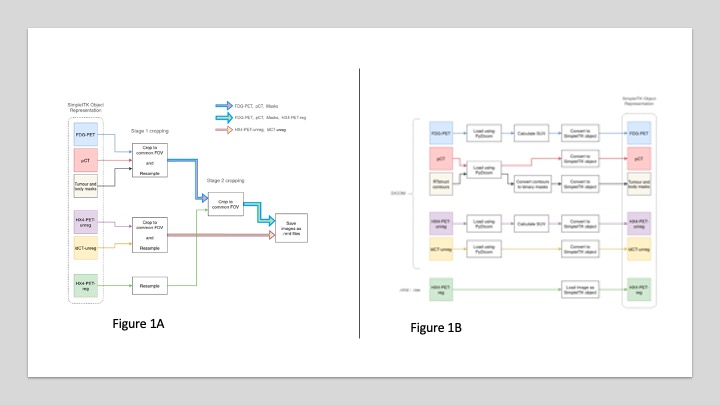
Results
Our experiments show that the modified CycleGAN attains high image-level performance, close to the ones of Pix2Pix (Figure 2).
Furthermore, our adapted cycle GAN performed better in the downstream task of reproducing hypoxia levels in the tumour regions.
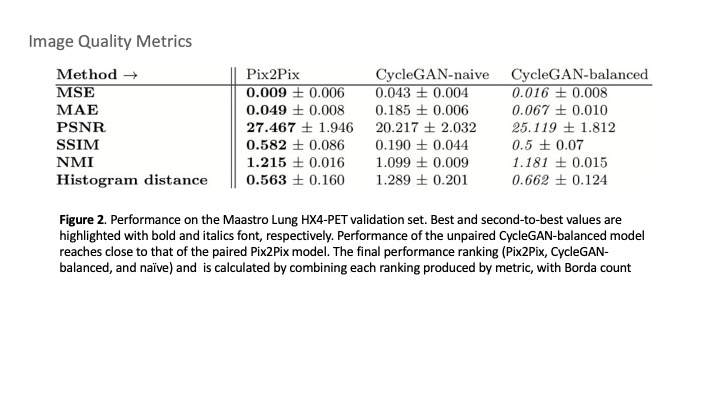
Conclusion
Our experiments show that the modified CycleGAN attains high image-level performance, close to that of Pix2Pix, and although our synthetic HX4-PET images may not yet meet the clinical standard, the results suggest that unpaired translation approaches could be more suitable for the task due to their immunity to noise induced in the training data by spatial misalignments.
It is worth noticing that we used only 15 images for models' development, compared to conventional deep learning algorithms employing datasets that are at least an order of magnitude larger.