Comparison of Deep Learning synthetic CT methods for CBCT-guided adaptive prostate radiotherapy
Paul van Haaren,
The Netherlands
PO-1599
Abstract
Comparison of Deep Learning synthetic CT methods for CBCT-guided adaptive prostate radiotherapy
Authors: Yvonne de Hond1, Camiel Kerckhaert1, Paul van Haaren1, Rob Tijssen1
1Catharina Hospital, Radiotherapy, Eindhoven, The Netherlands
Show Affiliations
Hide Affiliations
Purpose or Objective
To improve CBCT quality, several deep-learning (DL) networks have been proposed to generate synthetic CTs (sCTs). These networks learn a transform between CBCT and planning CT (pCT) scans. While these networks are successful in areas like head & neck and thoracic cancer, the variable bladder and rectal filling poses considerable challenges for pelvic tumor sites. It is therefore unknown which type of DL architecture is most suitable for prostate cancer. In this study, paired and unpaired DL networks were assessed with focus on the structural agreement of the synthetic CT with the daily CBCT.
Material and Methods
A previously published Unet [1] and a CycleGAN [2] were trained assessed on pCT and first fraction CBCT images of 57 prostate cancer patients, acquired on a Philips big bore CT and Elekta XVI system respectively. Average interval between CBCT and pCT scan was 20 days (min 2, max 56). Preprocessing: signal intensities were normalized. PCT was registered to CBCT rigidly (rpCT) and deformed (dpCT) with Elastix registration toolbox v5. Training: Unet was trained with CBCT and rpCT as paired input and dpCT as ground truth (GT). Images with no acceptable deformable image registration (DIR) between pCT and CBCT were excluded in training, which resulted in 2236 training, 1073 validation and 482 test images. CycleGAN was trained with unpaired input CBCT and GT dpCT, with a number training/validation/test images of 3928/1073/482, respectively. Both models were trained for 200 epochs and lowest validation error epoch was used. Evaluation: sCT images were evaluated on the mean absolute error (MAE), structural similarity index measure (SSIM) between sCT and dpCT (GT). In addition, the rectal volume was determined to assess structural agreement with the CBCT.
Results
Fig. 1 shows a typical example from our test set. DIR was, even after fine-tuning, not always capable to reflect the anatomy changes due to variable rectal filling in the CBCT. This anatomical mismatch was also observed in the sCT generated by the Unet. Conversely, the CycleGAN was able to better preserve the anatomy of the CBCT, which is also reflected by the absolute differences in rectal volume between the CBCT and sCT (Fig 2). The MEA and SSIM, however, favored the Unet (MAE: 21.8±7.0 HU and 37.5±9.2 HU, and SSIM: 0.97±0.01 and 0.94±0.02 for Unet and CycleGAN, respectively). These differences were mainly driven by the fact that the Unet (incorrectly) aligned better with the dpCT, thus mimicking the registration errors between the dpCT and CBCT.
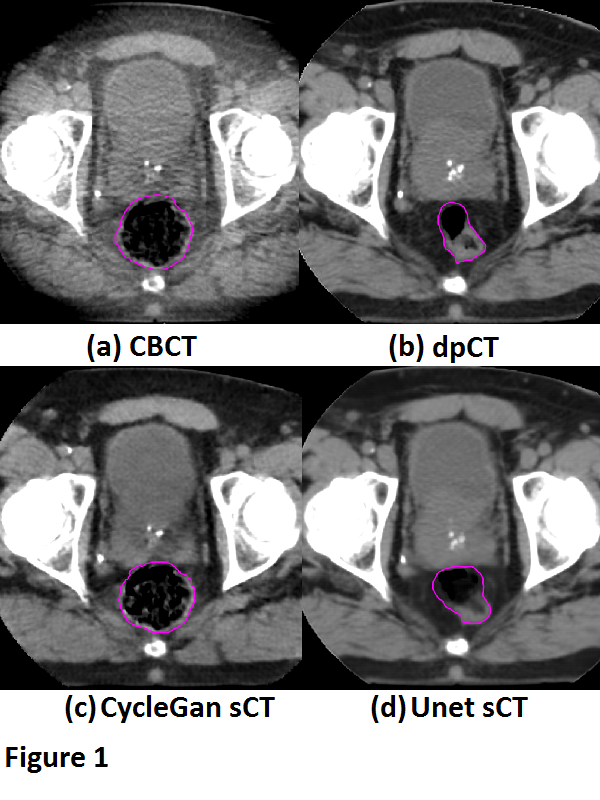
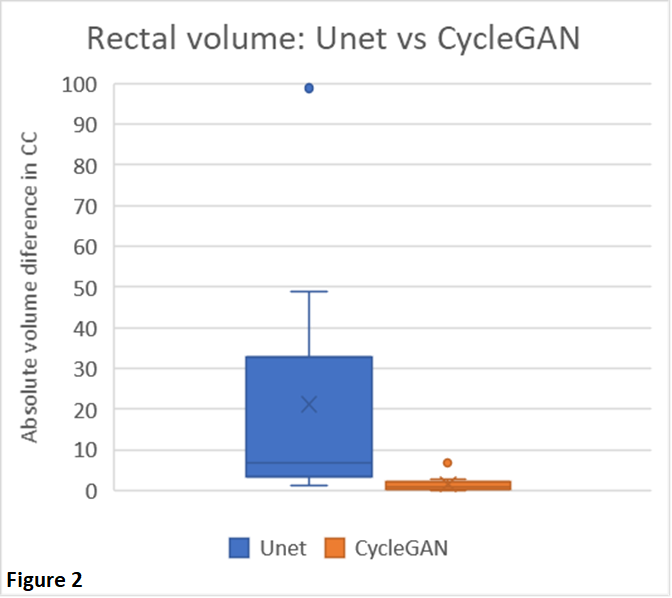
Conclusion
Paired training networks are challenged by the residual registration errors when forming GT data. The output of the Unet showed large anatomical deviations from the daily CBCT. However, the unpaired trained CycleGAN architecture performed much better in preserving the structural information of the daily CBCT and is therefore preferred for CBCT-guided adaptive prostate radiotherapy.
[1] DOI: 10.1002/mp.13978
[2] DOI: 10.1016/j.phro.2020.04.002