Deep learning based 4D synthetic CTs for daily proton dose calculations in lung cancer patients
Adrian Thummerer,
Germany
PO-1598
Abstract
Deep learning based 4D synthetic CTs for daily proton dose calculations in lung cancer patients
Authors: Adrian Thummerer1, Carmen Seller Oria1, Paolo Zaffino2, Kim Veldman1, Arturs Meijers1, Joao Seco3,4, Robin Wijsman1, Johannes Albertus Langendijk1, Antje Christin Knopf1,5, Maria Francesca Spadea2, Stefan Both1
1University Medical Center Groningen, Department of Radiation Oncology, Groningen, The Netherlands; 2Magna Graecia University, Department of Experimental and Clinical Medicine, Catanzaro, Italy; 3Deutsches Krebsforschungszentrum (DKFZ), Department of Biomedical Physics in Radiation Oncology, Heidelberg, Germany; 4Heidelberg University, Department of Physics and Astronomy, Heidelberg, Germany; 5University Hospital of Cologne, Center for Integrated Oncology Cologne, Cologne, Germany
Show Affiliations
Hide Affiliations
Purpose or Objective
Recently deep
neural networks have shown promising results in correcting image quality
deficiencies of CBCT images in multiple anatomical locations. Corrected CBCT
images, also referred to as synthetic CTs (sCT), can enable daily adaptive
proton therapy workflows, which rely on a recalculation of treatment plans on an
up-to-date patient anatomy. Compared to 3D-CBCTs, 4D-CBCTs allow to assess the
patients breathing and tumour motion on a daily basis but suffer from
additional artifacts due to the reconstruction with a very limited projection
number. In this study we focused on extending previous deep learning approaches
to generate 4D-synthetic CTs using 4D-CBCTs reconstructed from projections acquired
with a 3D-protocol. The suitability of 4D-sCTs for proton dose calculations in
lung cancer patients was evaluated in terms of image quality and dosimetric
accuracy.
Material and Methods
CBCT projections
and 4D-CTs of 50 lung cancer patients, treated with proton therapy, were used
to reconstruct 4D-CBCTs and train a U-net like convolutional neural network to
generate 4D-sCTs. Projection phase binning was performed using the Amsterdam
Shroud method, and the binned projections were reconstructed into six breathing
phases using the MA-ROOSTER reconstruction algorithm. A same-day 4D-CT (ten
phases) was used as ground truth image for training and image-quality/dosimetric
evaluation. Training of the neural network was performed with deformably
registered pairs of 0% phase images of 4D-CBCT and 4D-CT. The dataset was split
into a training (25 patients), validation (5) and test set (20). In the test
set, image quality (mean absolute error, MAE and mean error, ME) and dosimetric
accuracy (3%/3mm gamma analysis) were evaluated on the 0% (inhale) and 50%
(exhale) phases. The proton dose distributions were generated by recalculating
clinical 2-3 field IMPT treatment plans. Furthermore, we also compared MAE, ME
and gamma results of 4D-sCTs to 3D-sCTs from a previous study with thorax
patients.
Results
Figure 1 shows an
overview of the 0% phase images of the 4D-CBCT, 4D-CT, and the 4D-sCT. An average
MAE of 51.5 ± 5.6 HU and ME of 0.2 ± 5.2 HU were observed between 0% phase of 4D-sCT
and 4D-CT. Evaluation of the 50% phase resulted in similar results (MAE: 52.4 ±
6.0, ME: 3.3 ± 5.3). The recalculation of clinical treatment plans resulted in
average gamma pass ratios of 93.2 ± 3.0 % for the 0% phase and 92.5 ± 3.6 %. Figure
2 shows MAE and Gamma results of 0% and 50% phase for each patient. For
comparison, 3D-sCTs resulted in an average MAE of 34.7 ± 7.2 HU and a gamma
pass ratio of 93.9 ± 4.6 %.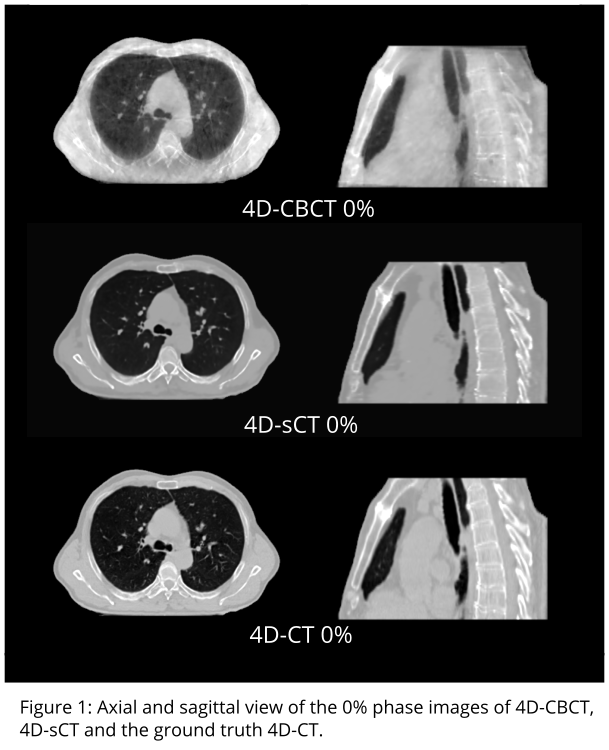
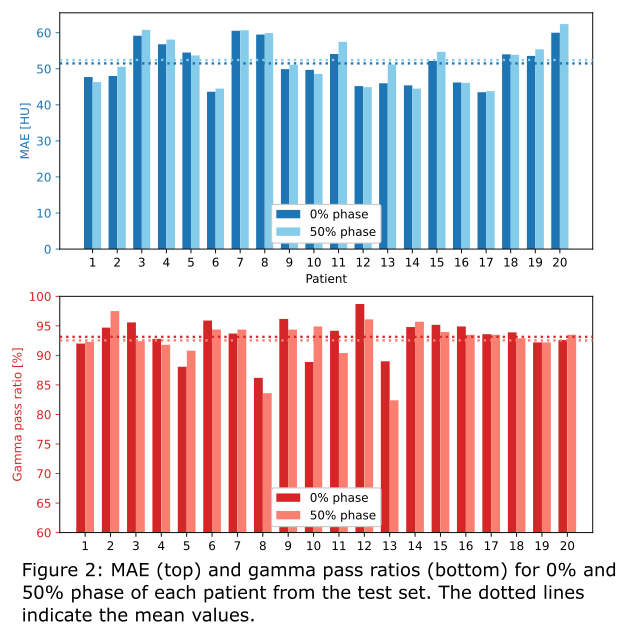
Conclusion
This study indicates the potential suitability of
4D-sCTs for proton dose calculations in adaptive proton therapy of lung cancer
patients. Despite the lower image quality of 4D-CBCTs, dosimetric accuracy of
4D-sCTs is comparable to 3D-sCTs. However further studies are required to investigate
reliability and clinical usability of 4D-sCTs.