Radiomic and dosiomic prediction of biochemical failure after Iodine-125 prostate brachytherapy
PO-1788
Abstract
Radiomic and dosiomic prediction of biochemical failure after Iodine-125 prostate brachytherapy
Authors: Masahiro Nakano1, Hiromichi Ishiyama1, Shogo Kawakami1, Akane Sekiguchi1, Takuro Kainuma1, Hideyasu Tsumura2, Masatoshi Hashimoto3, Tomoyuki Hasegawa3, Yuichi Tanaka4, Takeo Katakura4, Yuu Murakami5
1Kitasato University School of Medicine, Department of Radiation Oncology, Sagamihara-shi, Japan; 2Kitasato University School of Medicine, Department of Urology, Sagamihara-shi, Japan; 3Kitasato University School of Allied Health Sciences, Department of Medical Engineering and Technology, Sagamihara-shi, Japan; 4Kitasato University, Graduate School of Medical Sciences, Sagamihara-shi, Japan; 5Cancer Institute Hospital, Radiation Oncology Department, Koto-ku, Japan
Show Affiliations
Hide Affiliations
Purpose or Objective
The
aim of this study was to compare prediction performance of biochemical failure
for prostate cancer patients treated with low dose rate brachytherapy using Iodine-125
seeds between Machine Learning (ML) models using radiomic and dosiomic
features.
Material and Methods
Ninety-nine prostate cancer patients treated with
Iodine-125 seed implantation between October 2009 and March 2013 were included
in this study. Fourteen patients were observed with biochemical failure (BF)
and 85 were not. CT scans with 2-mm slice thickness were examined one month
after implantation and dose distribution (DD) was calculated on the CT slices
using Variseed Ver. 9.0.2 software provided by Varian Medical Systems (Palo
Alto, CA). Radiomic and dosiomic features within region-of-interest (ROI) of
prostate were extracted from CT slices and DDs, and 8 kinds of their wavelet-transformed
images and Laplacian of Gaussian (LoG) filtered images (Sigma = 1.0 and 1.5),
using PyRadiomics Ver. 3.0.1. Obtained
1038 feature values were normalized with z-score. Pearson’s correlation
coefficient with the threshold of 0.8 was used in order to remove highly
correlated features, and then features which p-values of Mann Whitney’s U-test
were less than 0.05 were employed in ML modeling. Those feature selection
methods were implemented sequentially in each trial of leave-one-out cross-validation
(LOOCV) flamework. ML methods used in this study were logistic regression with L1
regularization term (LRL1) and L2 term (LRL2), and random forest classifier (RFC),
provided in Scikit-Learn (Ver. 0.23.1) ML library. Prediction models were validated
in LOOCV framework and compared using area under the curve (AUC) values of the
receiver operator characteristic analysis. Importance of features in RFC and
normalized absolute weight values for features in logistic regression models
were also obtained and discussed.
Results
The AUC values of LRL1, LRL2, and RFC using
radiomic features were 0.513, 0.624, and 0.645, respectively, and the ones
using dosiomic features were 0.650, 0.922, and 0.697, respectively. Ninety-nine
times of feature selection process and modeling trials were implemented in
LOOCV modeling cycles, and 8 dosiomic features out of 1038 were selected more
than 90 times. Analysis of normalized importance in RFC and absolute weight
values in LRL1 and LRL2 for 8 dosiomic features shows that
wavelet-LLH_NGTDM_Busyness and Strength shows higher importance in LRL1 and RFC
whereas importance are widely distributed, including volume of prostate and
Busyness of LoG filtered image, in the case of LRL2.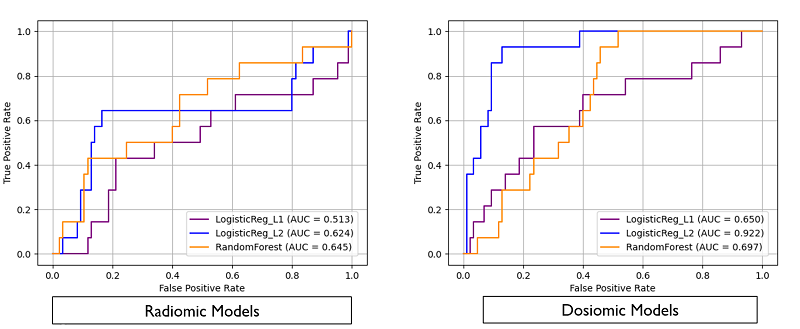
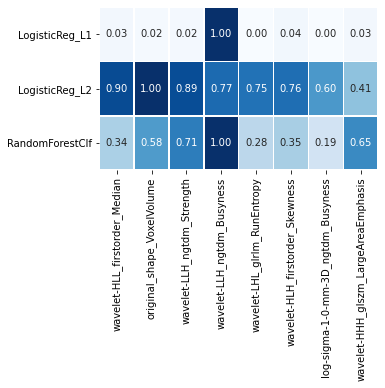
Conclusion
Dosiomic
prediction models were predicting biochemical failure superior than radiomic
models. Logistic regression model with L2 regularization using dosiomic
features were resulting the AUC value of 0.922, which was the best in this
study.