Leverage radiomic and clinical data in predicting SRS treatment outcomes in patients with brain mets
PO-1783
Abstract
Leverage radiomic and clinical data in predicting SRS treatment outcomes in patients with brain mets
Authors: Gianluca Carloni1,2, Giulia Marvaso2,3, Cristina Garibaldi4, Mattia Zaffaroni2, Stefania Volpe2,3, Matteo Pepa2, Sara Raimondi5, Giuliana Lo Presti5, Vincenzo Positano1,6, Roberto Orecchia7, Barbara Alicja Jereczek-Fossa2,3
1University of Pisa, Department of Information Engineering, Pisa, Italy; 2IEO European Institute of Oncology IRCCS, Division of Radiation Oncology, Milan, Italy; 3University of Milan, Department of Oncology and Hemato-Oncology, Milan, Italy; 4IEO European Institute of Oncology IRCCS, Unit of Radiation Research, Milan, Italy; 5IEO European Institute of Oncology IRCCS, Molecular and Pharmaco-Epidemiology unit, Department of Experimental Oncology, Milan, Italy; 6National Research Council - Region of Tuscany, G. Monasterio Foundation, Pisa, Italy; 7IEO European Institute of Oncology IRCCS, Scientific Directorate, Milan, Italy
Show Affiliations
Hide Affiliations
Purpose or Objective
In this
study, advanced models integrating radiomic
features derived from magnetic resonance (MR) images and
clinical data were developed for the prediction of local control (LC), distant
progression (DP) and overall survival (OS) in patients treated with SRS for BM
from non-small cell lung cancer. By doing so, we
aimed to investigate the variability in model performance when extracting features
with different platforms, and if the empowering of clinical models with
radiomics could benefit performance.
Material and Methods
A total of 148 patients treated at the same
institution, with a total of 276 BM, were retrospectively included. Pre-treatment
T1-weighted MR images of the brain were considered. Radiomic features
were extracted from the structures of each brain lesion with two
different platforms: PyRadiomics
(PyR) and SOPHiA Radiomics (SR). A total of
1129 and 192 features were considered for statistical analysis (see Fig1),
respectively. Clinical data were collected for each patient from the follow-up
reports. Five different
models were developed for each endpoint: two radiomic models (PyR and
SR), one clinical model and two combined models (integrating clinical with PyR
and SR information respectively). Performance was
asserted in terms of Harrell’s C-index, see Fig2.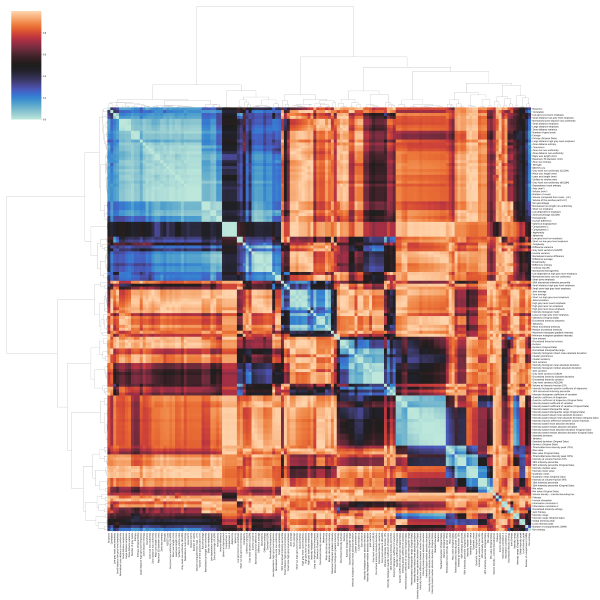
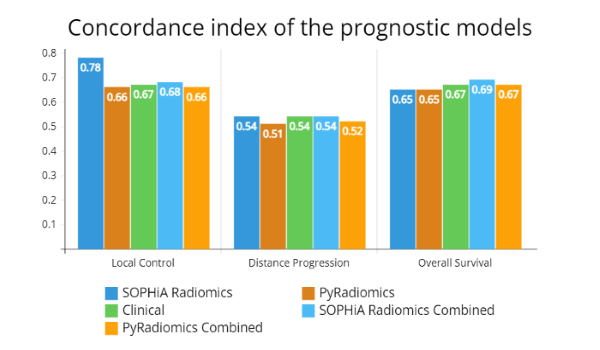
Results
In predicting LC,
the SR radiomic model outperformed the PyR one. From the
clinical model, an increase in the patient's age associated with a slight
increase in the probability of LC for his lesions. Cerebellar
lesions and concomitant therapy were associated with an increased rate of LC
compared to frontal ones and no-therapy, respectively. In
predicting DP, 6 radiomic features
were significant in the SR model, while only 1 in the PyR
radiomic model. Results from the clinical model
suggest that parietal and occipital BM are more prone to DP
than frontal ones, as are patients
with stage IV at diagnosis. In contrast, concomitant therapy resulted in lower DP
rate than no-therapy. The
combined models showed some differences. In predicting OS, both
radiomic models performed equally well. Clinical model’s performance
was slightly better than that of the radiomic models. The patient's KPS and
prescribed BED were associated with an extended OS,
while receiving not-concomitant therapy was associated with lower OS
than not receiving therapy at all. The SR
combined model
performed slightly better than the others. Again, differences exist between the
two combined models.
Conclusion
Overall, the best
performing model was the SR radiomic model for LC prediction. DP
was the least predictable endpoint in our
dataset. This study reveals the choice of radiomic platform may
result in differences in performance, and how the merge of both sources of data
might not always led to improvement. It provides some
important insights into the design of future prospective studies and suggests
that individualised assessment of LC and OS probability could be aided
by means of such models, for improved decision-making and prognostic assessment.