Methodological Quality of Machine Learning Quantitative Image Analysis Studies in Esophageal Cancer
Zhen Zhang,
The Netherlands
PO-1782
Abstract
Methodological Quality of Machine Learning Quantitative Image Analysis Studies in Esophageal Cancer
Authors: Zhen Zhang1, Leonard Wee2, Zhenwei Shi3, Andre Dekker2
1MAASTRO, Radiation oncology, Maastricht, The Netherlands; 2MAASTRO, Radiation Oncology, Maastricht, The Netherlands; 3Guangdong Provincial People's Hospital, Radiology, Guangdong, China
Show Affiliations
Hide Affiliations
Purpose or Objective
Studies based on
machine learning-based quantitative imaging techniques have gained much
interest in cancer research. The aim of this review is to critically appraise
the existing machine learning-based quantitative imaging analysis studies
predicting outcomes of esophageal cancer after concurrent chemoradiotherapy in
accordance with PRISMA guidelines.
Material and Methods
A systematic review was
conducted in accordance with PRISMA guidelines. The citation search was
performed via PubMed and Embase Ovid databases for literature published before
April 2021. From each full-text article, study characteristics and model
information were summarized. We proposed an appraisal matrix with 13 items to
assess the methodological quality of each study based on recommended best-practices
pertaining to quality.
Results
Out of 244 identified
records, 37 studies met the inclusion criteria. Study endpoints included
prognosis, treatment response, and toxicity after concurrent chemoradiotherapy
with reported discrimination metrics in validation datasets between 0.6 and
0.9, but with wide variation in quality. A total of 30 studies published in the
last five years were evaluated for methodological quality and we found 11 studies
with at least 6 “Good” item ratings.
Table 1. Assessment of
methodological quality of included studies.
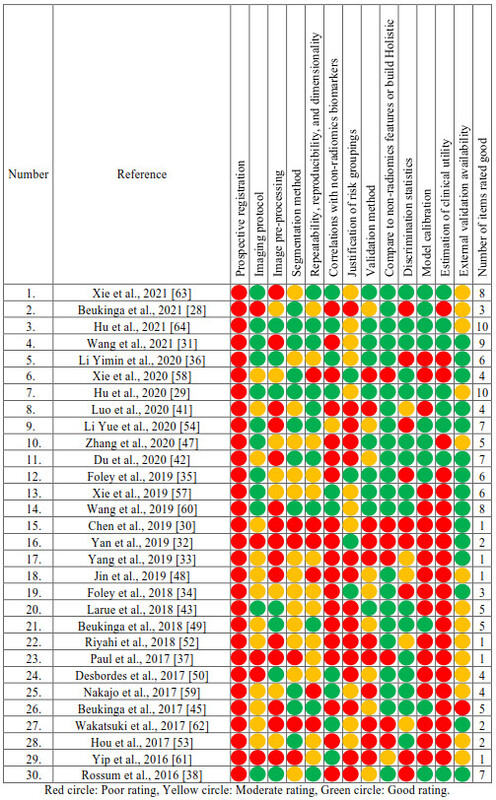
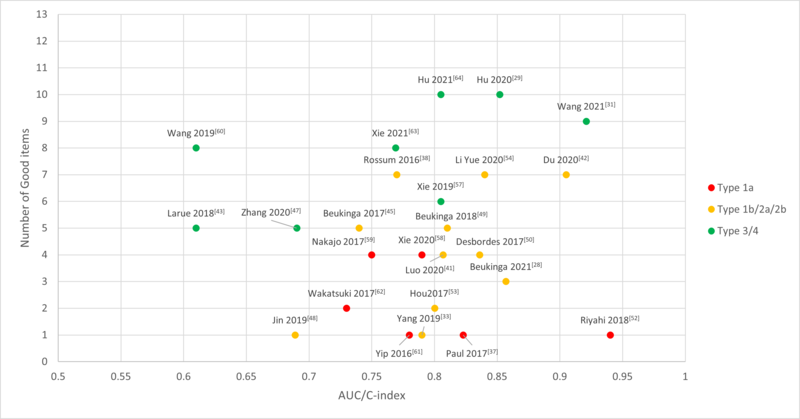
Figure 1. Reported AUC/C-index of the included studies with number of good items
were classified by Transparent Reporting of a multivariable prediction model
for Individual Prognosis Or Diagnosis (TRIPOD). Type 1a: Development only; Type 1b:
Development and validation using resampling; Type 2a: Random split-sample development
and validation; Type 2b: Nonrandom split-sample development and validation; Type
3: Development and validation using separate data; Type 4: Validation only.
Conclusion
A substantial number of
studies lacked prospective registration, external validation, model
calibration, and support for clinical use. To further improve the predictive
power of machine learning-based models and translate into real clinical
applications in cancer research, appropriate methodologies, prospective
registration and multi-institution validation are recommended.