Prediction of recurrence from post-operative MRI in GBM: Are we reaching limits of Deep-Learning?
PO-1771
Abstract
Prediction of recurrence from post-operative MRI in GBM: Are we reaching limits of Deep-Learning?
Authors: Alexandre CARRÉ1,2, Guillaume Klausner1,2, Samir Achkar1,2, Théo Estienne2,4, Théophraste Henry3, Angela Rouyar1,2, Roger Sun1,2, Grégoire Fournier1,2, Frédéric Dhermain1,2, Eric Deutsch1,2, Charlotte Robert1,2
1Gustave Roussy Cancer Campus, Department of radiation oncology, Villejuif, France; 2Université Paris-Saclay, Gustave Roussy Cancer Campus, U1030 Radiothérapie Moléculaire et Innovation Thérapeutique, Villejuif, France; 3Gustave Roussy Cancer Campus, Department of radiology, Villejuif, France; 4Université Paris-Saclay, CentraleSupélec, Mathématiques et Informatique pour la Complexité et les Systèmes, Gif-sur-Yvette, France, France
Show Affiliations
Hide Affiliations
Purpose or Objective
Glioblastomas (GBM) are the most common primary
brain tumors in adults with a high lethality. Recurrences in this tumor
histology are mostly local, despite aggressive treatments combining surgery, chemotherapy,
and radiotherapy (RT). Today, RT is prescribed using a “one fits all” concept,
i.e., the same dose is prescribed in the whole Planning Target Volume without
consideration of local tumor aggressiveness. We made the hypothesis that GBM
would benefit from voxel-scale dose painting. We thus investigated whether
deep-learning models could predict recurrence sites based on post-operative
anatomical MR images.
Material and Methods
All
adult patients treated for a histologically proven GBM between 2008 and 2015 in
a single institution using a conventional normofractionated RT scheme within
STUPP protocol were included. An additional inclusion criterion was the
availability of the following sets of images at both baseline (TBaseline)
and recurrence (TRecurrence) times: a T1-w axial MRI sequence, a
T1-w axial MRI sequence with gadolinium injection, and a T2-w axial FLAIR
sequence. Primary surgery consisted of either stereotactic biopsy, subtotal resection,
or safe wide resection. In all cases, RT delivered a total dose of 60 Gy in 30
fractions, using either 3D conformal RT or intensity-modulated RT. Recurrence
was defined as the confirmed appearance of new contrast uptake at recurrence
time compared to baseline. A simple task learning strategy based on a modified 3D
U-Net architecture was implemented first, with the goal to identify recurrence
areas based on the 3 baseline MRI. Sequential and parallel multitask models
aiming at segmenting the enhancing part of the tumor at TBaseline in
addition to recurrence areas were considered also. Dice Loss, Binary
Cross-Entropy (BCE) Loss, and Dice/BCE Loss were used for optimization. The
dataset was randomly split into training (159 patients) and testing (40
patients) sets. Models were trained from scratch using a five-fold cross-validation
procedure. Ensembling of the
models obtained for the five folds was used for inference on the test set.
Networks’ performances were evaluated using the Dice coefficient (DSC) as well
as balanced accuracy, sensitivity, and specificity metrics.
Results
Among the 199 patients evaluated, 140 had local
recurrence (70%), and 175 (88%) had at least part of the recurring tumor
developing from the edema. Table 1 summarizes the results obtained on the test
set. The best model obtained a DSC of 0.201. Models exploiting multitasking did
not improve performance. The use of a sum of the two losses (Dice + BCE) did
not show either a significant contribution whatever the model.
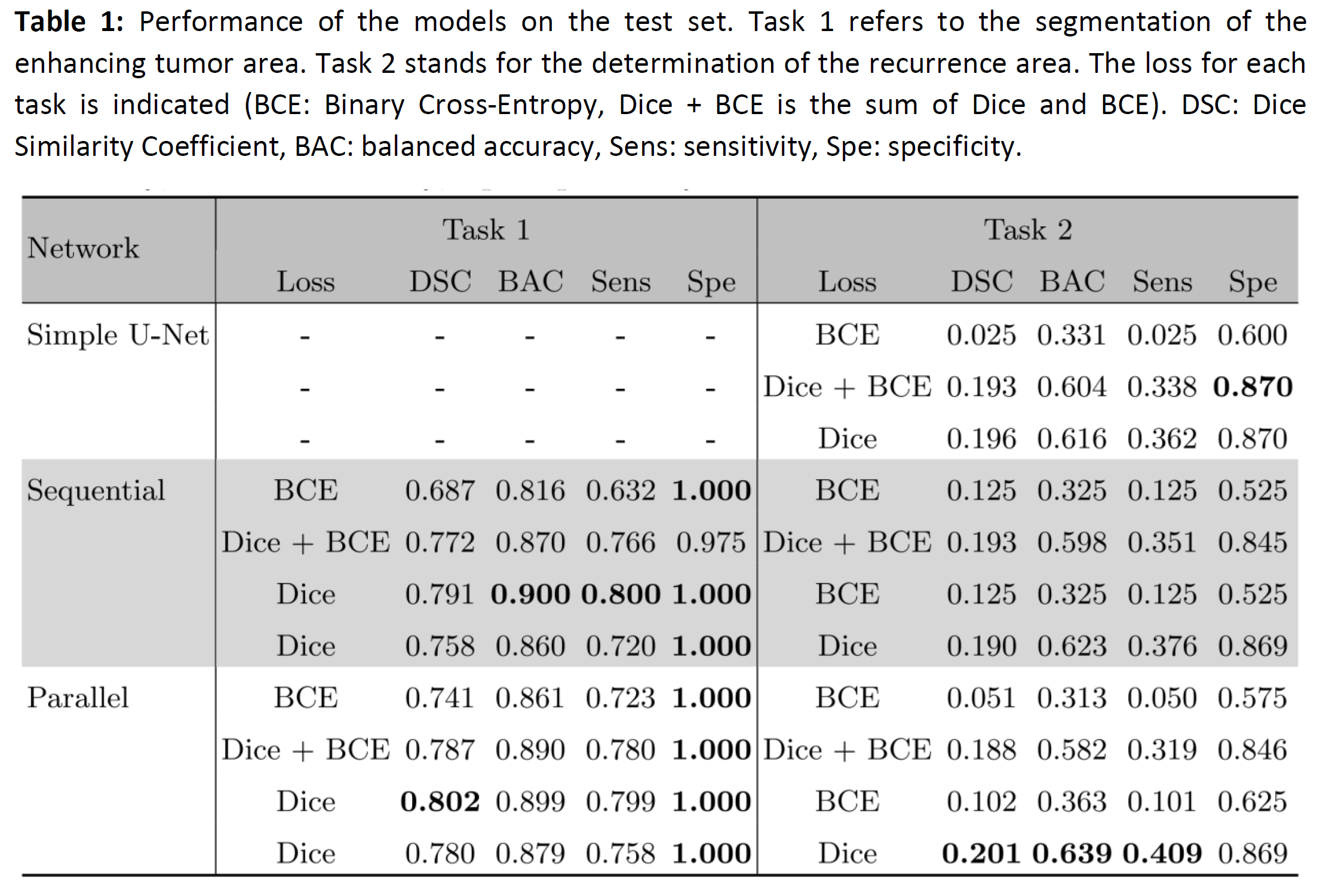
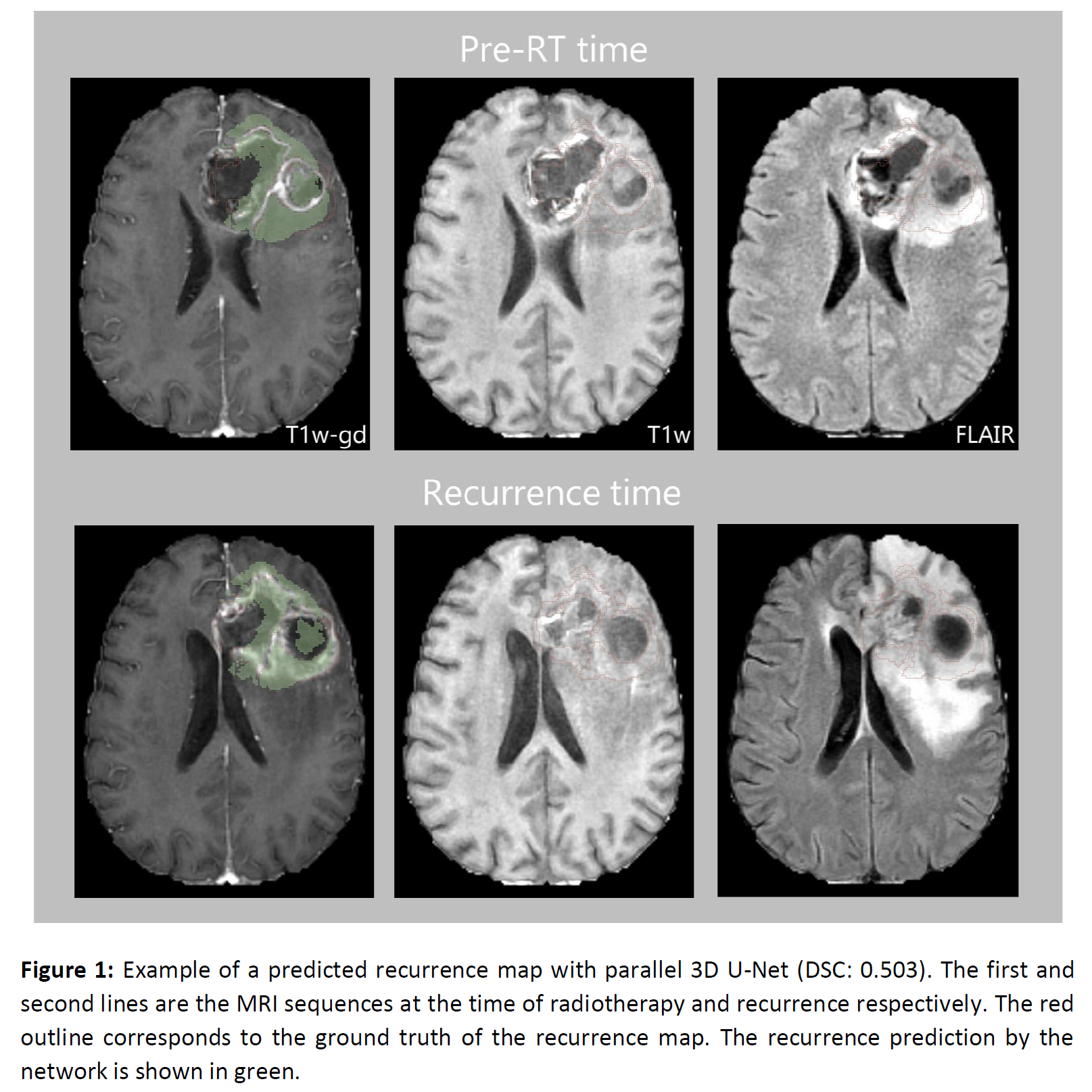
Conclusion
The poor performance highlights the major
difficulty of the task, which cannot be solved from anatomical MRI images alone,
except perhaps by drastically increasing the amount of data. The addition of
functional sequences such as PET or MRI will allow to conclude on the ability
to describe tumor aggressiveness in a pre-radiotherapy setting.