Prostate cancer radiogenomics machine learning classification for predicting disease progression
Ross Murphy,
United Kingdom
PO-1769
Abstract
Prostate cancer radiogenomics machine learning classification for predicting disease progression
Authors: Ross Murphy1, Neree Payan1, Sarah Osman1,2, Kevin Prise1, Alan Hounsell1,2, Joe O'Sullivan1,3, Conor McGarry1,4, Suneil Jain5,6
1Queen's University Belfast, Patrick G. Johnston Centre for Cancer Research, Belfast, United Kingdom; 2Belfast Health and Social Care Trust, Department of Radiotherapy Physics, Belfast, United Kingdom; 3Belfast Health and Social Care Trust, Department of Clinical Oncology, Belfast, United Kingdom; 4Department of Radiotherapy Physics, Belfast Health and Social Care Trust, Belfast, United Kingdom; 5Queen's Unversity Belfast, Patrick G. Johnston Centre for Cancer Research, Belfast, United Kingdom; 6Department of Clinical Oncology, Belfast Health and Social Care Trust, Belfast, United Kingdom
Show Affiliations
Hide Affiliations
Purpose or Objective
More than 30% of prostate cancer patients will relapse within five years following radiotherapy treatment. Clinicopathological features do not have the ability to predict patients that will relapse and benefit from treatment intensification. Radiogenomics classification models have also demonstrated prognostic value in various cancer types. However, radiogenomics classification models in prostate cancer have yet to be developed. Our study aimed to investigate the prognostic ability of clinicopathological, radiomics, gene expression, and radiogenomics classification models for predicting disease progression in prostate cancer patients who have received radiotherapy plus androgen deprivation therapy (ADT).
Material and Methods
184 prostate cancer patients had their tumour transcriptome captured via microarray with 19453 features, and 5983 radiomics features were extracted from their CT scans before treatment. A feature selection/random forest pipeline, and generalized linear models (GLMs) with feature selection via the least absolute shrinkage and selection (LASSO) and ElasticNet, were used as classification models. Biochemical recurrence (BCR) and metastatic disease were used as classification labels, with 43 patients having had BCR, and 18 patients with metastatic disease. Stratified partitioning on these classification labels randomly assigned two thirds of the cohort into the training dataset, and one third into testing dataset. For the radiomics features, pairwise Pearson correlations were calculated, and highly correlated features were removed (r > 0.8). For the gene expression features, differentially expressed genes (DEGs; p-value < 0.01) were identified for both the classification labels to be used as the gene expression input data.
Results
1429 robust radiomics features were identified following ICC, and 154 radiomics features were selected following pairwise correlation filtering to be used as radiomics input data. 195 DEGs were associated with BCR and 233 DEGs were associated with metastatic disease. The results of the clinicopathological, radiomics, gene expression, and radiogenomics models for both BCR and metastatic disease prediction are presented in Table 1 and Figure 1.
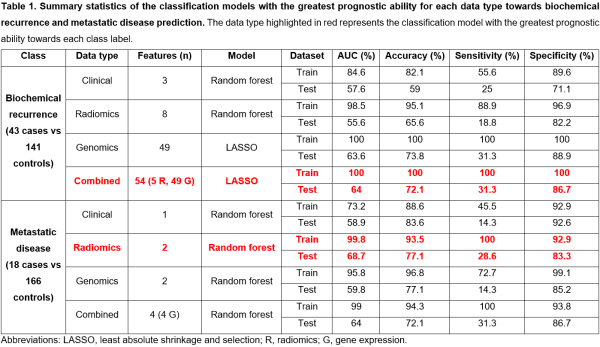
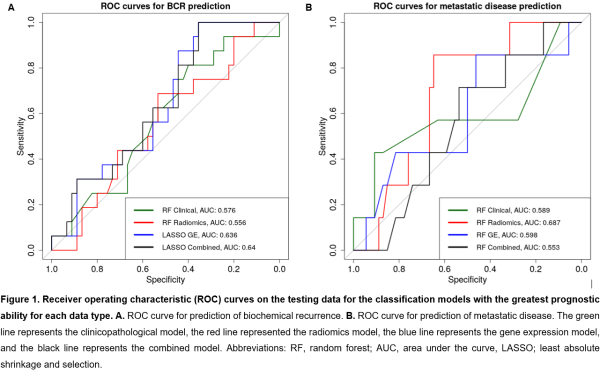
A combined radiogenomics model has the greatest prognostic ability for BCR, despite performing only slightly better than the gene expression model. For metastatic disease prediction, the radiomics model had the greatest prognostic ability.
Conclusion
This study has utilised machine learning classification models to assess the predictive ability of a novel radiogenomics signature in prostate cancer disease progression. Radiogenomics models had the greatest predictive ability for BCR in comparison with clinicopathological, radiomics, and gene expression models. The availability of a larger prostate cancer radiation response cohort with more disease progression events in the future could provide a pathway to the identification of a robust radiogenomics prognostic signature.