Regularized distributed Cox regression: a model for federated feature selection in survival analysis
BENEDETTA GOTTARDELLI,
Italy
PO-1768
Abstract
Regularized distributed Cox regression: a model for federated feature selection in survival analysis
Authors: Benedetta Gottardelli1, Carlotta Masciocchi2, Antonella Martino3, Luca Boldrini3, Ciro Mazzarella3, Giulio Grassi4, Mariangela Massaccesi3, Vincenzo Valentini3, Andrea Damiani2
1Università Cattolica del Sacro Cuore, Diagnostica per Immagini, Radioterapia Oncologica ed Ematologia, Rome, Italy; 2Fondazione Policlinico Universitario Agostino Gemelli, Gemelli Generator Real World Data, Rome, Italy; 3Fondazione Policlinico Universitario Agostino Gemelli, Diagnostica per Immagini, Radioterapia Oncologica ed Ematologia, Rome, Italy; 4King’s College London, Computer Science, Rome, Italy
Show Affiliations
Hide Affiliations
Purpose or Objective
Radiomic studies typically involve a large
number of features that makes it difficult for a single institution or even
multiple centres in a distributed learning setting to meet the adequate number
of patients for statistically significant outputs for survival analysis. In
these cases, feature selection methods can be very helpful to reduce the study
variables to a smaller group, including only the most outcome-relevant ones,
thus loosening the constraint on the cohort size. The aim of this work is to
develop and validate, both on simulated and on real world data, a new algorithm
performing feature selection for Cox Proportional Hazard survival analysis in a
privacy-preserving setting through distributed learning.
Material and Methods
Lasso
regularization was chosen as feature selection method due to its capability of
gradually reducing, during model training, the absolute values of the
coefficients of less significant covariates, setting to 0 the coefficients of
non-significant or redundant features. The algorithm for the distributed Cox
regression with Lasso regularization was implemented in Python 3.7; we also
designed and developed an automated testing & validation platform to
generate survival data, simulate the federated learning and compare the result
of the new algorithm with those of any given ground truth solution. We assessed
the algorithm performances calculating the mean absolute error (MAE) between its
regression coefficients and those obtained from Python centralised
state-of-the-art algorithm from the “sksurv” library.
The first step of the validation process involved
testing the algorithm on several simulated survival datasets with a number of
covariates ranging from 10 to 100 varying the regularization parameter (α) from 0.1 to 0.7. Secondly, we used for further
validation a real dataset of 20 radiomic features extracted from RT-planning CT
scans of patients affected by Lung Cancer using Overall Survival as model outcome.
Results
We report,
in Table 1, 17 tests, 15 of which were done on simulated datasets and two on
real world data. For the simulated data, MAE was overall lower than < 0.01. For
the real world data testing, the algorithm was trained on 189 lung-cancer
patients with two different regularization parameters. Real world data had
coefficients in the distributed model, for both values of α, slightly more different than the centralised ground
truth resulting in a larger MAE compared to the simulated data, but overall
lower than 0.03. As expected, for both types of data, the number of excluded
variables (0-coefficients) increases together with α.
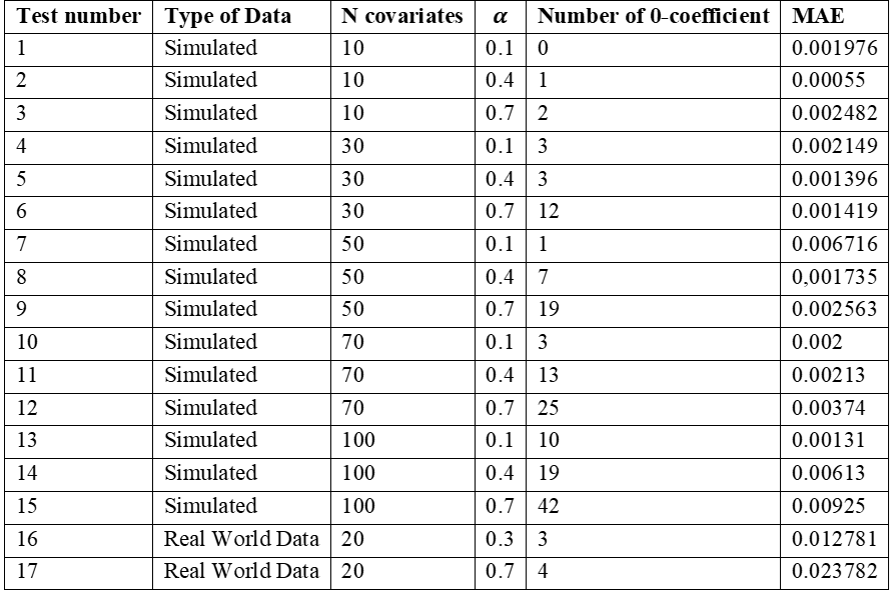
Conclusion
To the best
to our knowledge, this is the first implementation of a Cox Proportional Hazard
model with Lasso features selection for federated learning. In the near future,
the model will be tested in a non-simulated distributed setting on a real
multi-institution retrospective study.