Pre-processing and feature/volume correlation in CT radiomics in non-small cell lung cancer
PO-1765
Abstract
Pre-processing and feature/volume correlation in CT radiomics in non-small cell lung cancer
Authors: Stefania Volpe1, Lars Johannes Isaksson1, Matteo Pepa1, Mattia Zaffaroni1, Sara Raimondi2, Giuliana Lo Presti2, Cristina Garibaldi3, Cristiano Rampinelli4, Giulia Marvaso1, Sara Gandini2, Marta Cremonesi3, Barbara Alicja Jereczek-Fossa1
1Istituto Europeo di Oncologia IRCCS, Radiation Oncology, Milan, Italy; 2Istituto Europeo di Oncologia IRCCS, Experimental Oncology, Milan, Italy; 3Istituto Europeo di Oncologia IRCCS, Radiation Research Unit, Milan, Italy; 4Istituto Europeo di Oncologia IRCCS, Radiology, Milan, Italy
Show Affiliations
Hide Affiliations
Purpose or Objective
Radiomics
is increasingly used to implement clinically-based prognostic models for
non-small cell lung cancer (NSLCL). However, no evidence supports the choice of
specific imaging pre-processing methodologies. Admittedly, dedicated
investigations could contribute to refining both the reproducibility and the
performance of radiomic studies. As the volume of the primary tumor is a
well-recognized prognosticator, our purpose is to assess how pre-processing may
impact the feature-volume dependency in computed tomography (CT) images of
NSCLC patients treated with radiotherapy.
Material and Methods
Images
were retrieved from the publicly available repository NSCLC-Radiomics of The
Cancer Imaging Archive (TCIA). Four hundred eighteen images were included in
the analysis following manual inspection and editing of the segmentations;
nodal disease- if any- was not included. Pyradiomics was used to extract 93
features; which were grouped as follows: first-order, shape-based (3D),
shape-based (2D), gray level co-occurrence matrix (GLCM), gray level run length
matrix (GLRLM), gray level size zone matrix (GLSZM), neighboring gray tone
difference matrix (NGTDM) and gray level dependence matrix (GLDM). Twenty
built-in pre-processing methods (filters) were applied, including wavelet and
its possible permutations, Laplacian of gaussian, and local binary pattern (LPB);
each feature except those belonging to the shape category was computed once per
filter, and on the original CT image. The Spearman correlation coefficient (ρ) was
used; with thresholds of ≥0.7
and ≤0.5 defining strong and weak correlations, respectively.
Results
Overall,
features of the GLCM category were the least correlated with volume (ρ= 0.5).
The feature/volume correlation was found to be filter-dependent: the highest correlation
was found when lpb-3D-m1 was applied (ρ= 0.82), while the lowest correlations
with volume were identified for the HHL and HHH-wavelet filters, and for the
exponential method (ρ= 0.35, 0.30 and 0.18, respectively). These results were confirmed
when features computed per each pre-processing modality were compared to the
original image. An overview of the results is displayed in Figure 1.
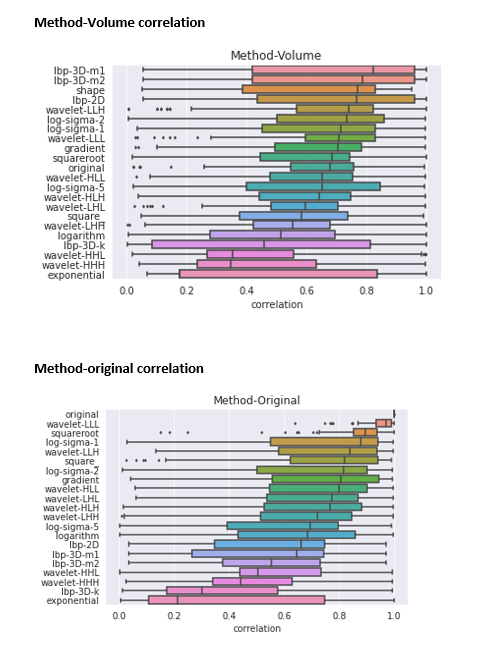
Conclusion
Our
results support the hypothesis that pre-processing does impact features
values; and provide a proof of concept that further standardization is
warranted for radiomic studies. Further- currently ongoing analyses- will focus on how these
findings impact the performance of radiomic-based survival models.