The chest CT examinations of the 417 NSCLC patients included in the public LUNG1 dataset were used in the study.
For the radiomic approach, handcrafted features, extracted from the whole 3D tumour volume, were fed to 24 different pipelines formed by the combination of 4 feature selectors/reducers (i.e., ANOVA f-value, random forest, principal component analysis and feature agglomeration) and 6 classifiers (i.e., support vector machines, feed forward neural networks, nearest neighbours, bagging, random forest and extreme gradient boosting) to predict the 2-year OS.
For deep learning, both the deep features extracted from the 2D tumour slices by a convolutional auto-encoder and the inner features learnt by a pre-trained convolutional neural network (CNN) were fed to the same 24 pipelines. In addition, the direct classification of the images with 3 different CNN architectures was tested, by considering both the original CT images and the synthetic images generated with a common data augmentation technique. The classification workflow and the total number of pipelines implemented for the three approaches are depicted in Figure 1. Finally, for each pipeline and approach, the performance with and without the inclusion of clinical parameters within the feature set was also evaluated in a cross-validation scheme.
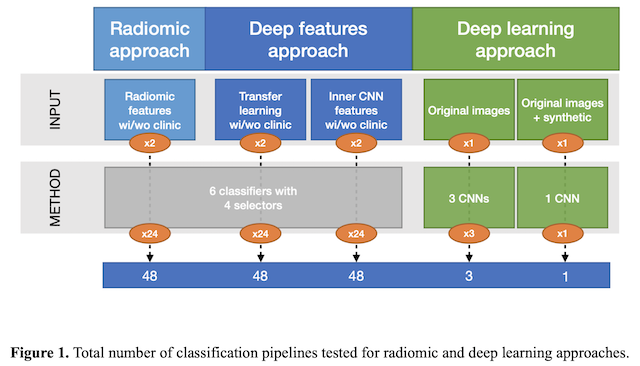