Early detection of brain metastases using diffusion weighted imaging radiomics and machine learning
Joseph Madamesila,
Canada
PO-1762
Abstract
Early detection of brain metastases using diffusion weighted imaging radiomics and machine learning
Authors: Joseph Madamesila1, Ekaterina Tchistiakova1,2,3, Nicolas Ploquin1,2,3
1University of Calgary, Department of Physics and Astronomy, Calgary, Canada; 2University of Calgary, Department of Oncology, Calgary, Canada; 3Alberta Health Services, Department of Medical Physics, Calgary, Canada
Show Affiliations
Hide Affiliations
Purpose or Objective
To develop a machine learning (ML)
model for early detection of brain metastases based on diffusion imaging
radiomics.
Material and Methods
Diffusion weighted MRI from 40
patients previously treated at our institution were retrospectively analyzed. Clinical
target volume contours from 193 metastases were extracted from radiosurgery
planning CTs and rigidly registered to corresponding Gd-T1 MRI and Apparent
Diffusion Coefficient (ADC) maps. Control volumes were generated using contralateral
contours located in healthy brain tissue to enable ML binary classification.
The ML input dataset consisted of: 1)
ADC-based radiomic features calculated within target volumes using Pyradiomics,
2) linear slopes and intercepts of each radiomic feature calculated using
timepoints before the metastasis manifested on conventional Gd-T1, 3) primary
cancer site and 4) anatomical target volume location data, identified by registering
images to the MNI152 T1 dataset and applying standard cortical and subcortical
atlases.
Correlation
analysis was performed and any features with >95% Pearson correlation were excluded.
The dataset was divided into training and validation sets using an 80/20 split
with stratification and scaled using Scikit-Learn’s StandardScaler. Five classification algorithms (SVM: Support Vector Machine, RF: Random Forest, MLP:
Multi-layer Perceptron, ADA: AdaBoost, XGB: XGBoost) performed supervised
learning using a 10-fold cross validation (CV) training set, with data labeled
as either ‘control’ or ‘metastasis’. Grid search was used to tune hyperparameters
for each algorithm (CV = 10), optimizing towards classifier balanced accuracy
score. Receiver-operator curve area (AUC) scores were calculated along with
accuracy, recall, and precision.
Results
ML algorithm performance is
summarized in Table 1. Gradient boosting-based algorithms XGBoost and AdaBoost showed
superior accuracy (XGB: 0.764 ± 0.053 and 0.790 ± 0.099, ADA:
0.746 ± 0.050 and 0.880 ± 0.074) for both training and validation sets, respectively. SVM, RF and MLP all
performed lower during training but remained comparable to other models when
tested against the validation set.
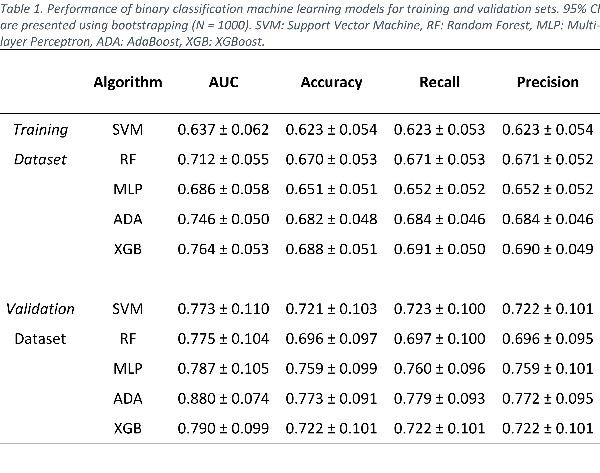
Twenty of the 25 most important ADA features
all involved the change in radiomic feature values within clinical target
volumes. Twenty-three of the 25 features were derived using wavelet filtered or
edge enhanced ADC images.
Conclusion
Gradient-boosting based ML
algorithms showed encouraging results in differentiating healthy brain tissue
from metastases when using diffusion images prior to the lesion detection on
conventional T1 MRI. ADA and XGB performed better than RF and SVM-based models
when detecting malignant tissue based on changes in diffusion weighted imaging
radiomic features. Future work will use these findings to further refine the
model by adding more patients and test cases, improving classification accuracy,
and increasing the model’s overall clinical applicability.