A framework for in-vivo, voxel-based assessment of radiation response through multimodal imaging
PO-1757
Abstract
A framework for in-vivo, voxel-based assessment of radiation response through multimodal imaging
Authors: Mikkel Skaarup1, Michael Juncker Lundemann1, Sune Darkner2, Morten Jørgensen1, Lisbeth Marner3, Dragan Mirkovic4, David Grosshans4, Christopher Peeler4, Radhe Mohan4, Ivan Richter Vogelius1, Ane Appelt5
1Copenhagen University Hospital - Rigshospitalet, Department of Oncology, Copenhagen, Denmark; 2Copenhagen University, Department of Computer Science, Copenhagen, Denmark; 3Copenhagen University Hospital - Rigshospitalet, Department of Clinical Physiology, Copenhagen, Denmark; 4MD Anderson, Department of Radiation Physics, Houston, USA; 5St. James's University Hospital, Leeds Cancer Centre, Leeds, United Kingdom
Show Affiliations
Hide Affiliations
Purpose or Objective
Modelling
radiation response of radiotherapy is a complex task, and most models on
clinical data only consider organ level effects. Capturing local tissue
response in vivo may allow for clearer linkage of dose and biological effect.
There is lack of robust methodology to incorporate longitudinal response
measures, anatomical changes over time, and the complex structure of multilevel
data. We proposed a framework to model radiation response in vivo,
using imaging biomarkers as a surrogate, which incorporates all these
aspects. The framework is available online.
Material and Methods
We
demonstrate the framework with an example cohort of 6 paediatric patients. Change
of fractional anisotropy on diffusion tensor MRI images was used as imaging response
biomarker as a loss of voxel intensity here has previously been linked to
radiation damage. CT, PET and other MRI sequences were included in the
framework to show the multi-modality capabilities.
By
acquiring images before and at multiple timepoints after radiotherapy, a
longitudinal dataset was collected. The images were combined into hybrid
templates at each timepoint, registered internally by affine transformation.
Each follow-up template was registered to the baseline template through
deformable hybrid template image registration. As we included the planning CT
scan, we created a dataset with per-voxel information of radiation dose and
imaging change over time.
Imaging changes over time were modelled on a voxel-by-voxel level with a
generalised linear mixed regression model, accounting for inter- and
intra-patient variations through nesting. To demonstrate the modelling aspect
of our framework, we considered biological effect of radiation dose and LET of
proton radiotherapy, according to:

where
n denotes the voxel. We
modelled the dataset both in a combined model across all patients and
follow-ups, and separately for each patient and follow-up. The latter to assess
inter- and intra-patient variations.
Results
The
framework was successful in registering multimodal, longitudinal imaging data,
see Figure 1. This enabled spatial mapping and modelling of radiation response.
Both
our combined model and individual models per follow-up correctly reproduced the
expected dose-dependency signal; however, we saw no clear dependence of LET
(the c term); see Figure 2.The inter- and intra-patient variations in radio
sensitivity appeared to overshadow any LET effects on tissue radiation
response. These specific results are discussed further elsewhere [1]. The
complete framework is available online [2].

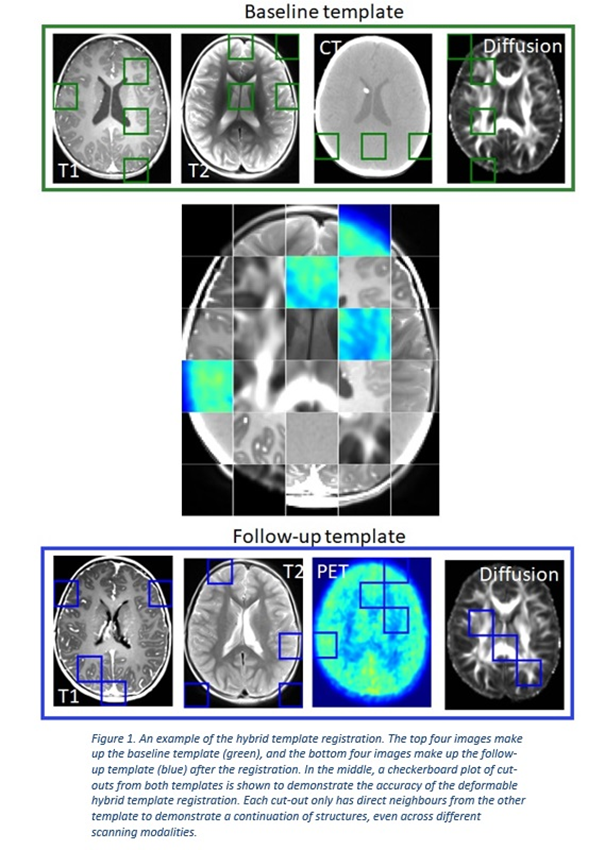
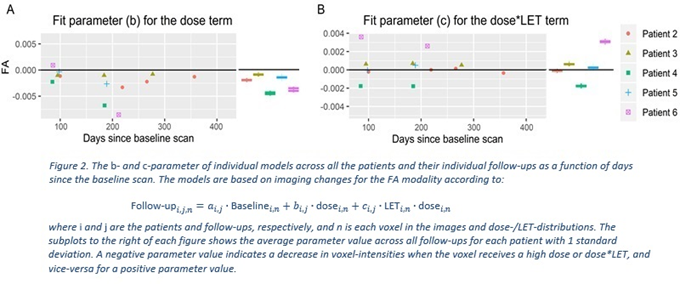
Conclusion
We
have demonstrated a powerful framework to rigorously model localised, in-vivo
radiation response effects in longitudinal datasets, while accounting for anatomical
changes over time. We demonstrated the ability to model imaging changes and
reproduce the expected result. We also saw large variations between patients,
indicating a need for accurate or composite, multimodal imaging biomarkers
which the framework is capable of handling.