Spatial Pyramid Pooling Survival Networks: Learning survival outcomes from whole slide images
Shenlun Chen,
The Netherlands
PO-1756
Abstract
Spatial Pyramid Pooling Survival Networks: Learning survival outcomes from whole slide images
Authors: Shenlun Chen1, Leonard Wee1, Andre Dekker1
1Maastricht University, Clinical Data Science, Faculty of Health Medicine and Lifesciences, Maastricht, The Netherlands
Show Affiliations
Hide Affiliations
Purpose or Objective
Learning survival outcomes on whole slide images (WSI) is a difficult
task for deep learning because the size of WSI has both characteristics of extremely large and arbitrary. In this
study, we developed spatial pyramid pooling survival networks (SPPSN) to
extract risk information from WSIs.
Material and Methods
We firstly trained a tissues classifier to
segment tissues of gland, stroma, immune cells, and other tissues (adipose,
muscle and debris). We also trained a gland formation classifier to segment
normal gland tissue and tumor with different gland formation. The two classifiers
were designed to extract information that may related to survival outcomes and
compress original WSIs into spatial heatmaps with smaller sizes for training.
Next, we used spatial pyramid pooling (SPP) to extract SPP features from
spatial heatmaps and then applied a deep survival networks after SPP features.
The total architecture was called SPPSN and was demonstrated in Fig1. SPPSN can
obtain deep survival grade form WSIs and the grade was evaluated by c index and
Kaplan-Meier curves.
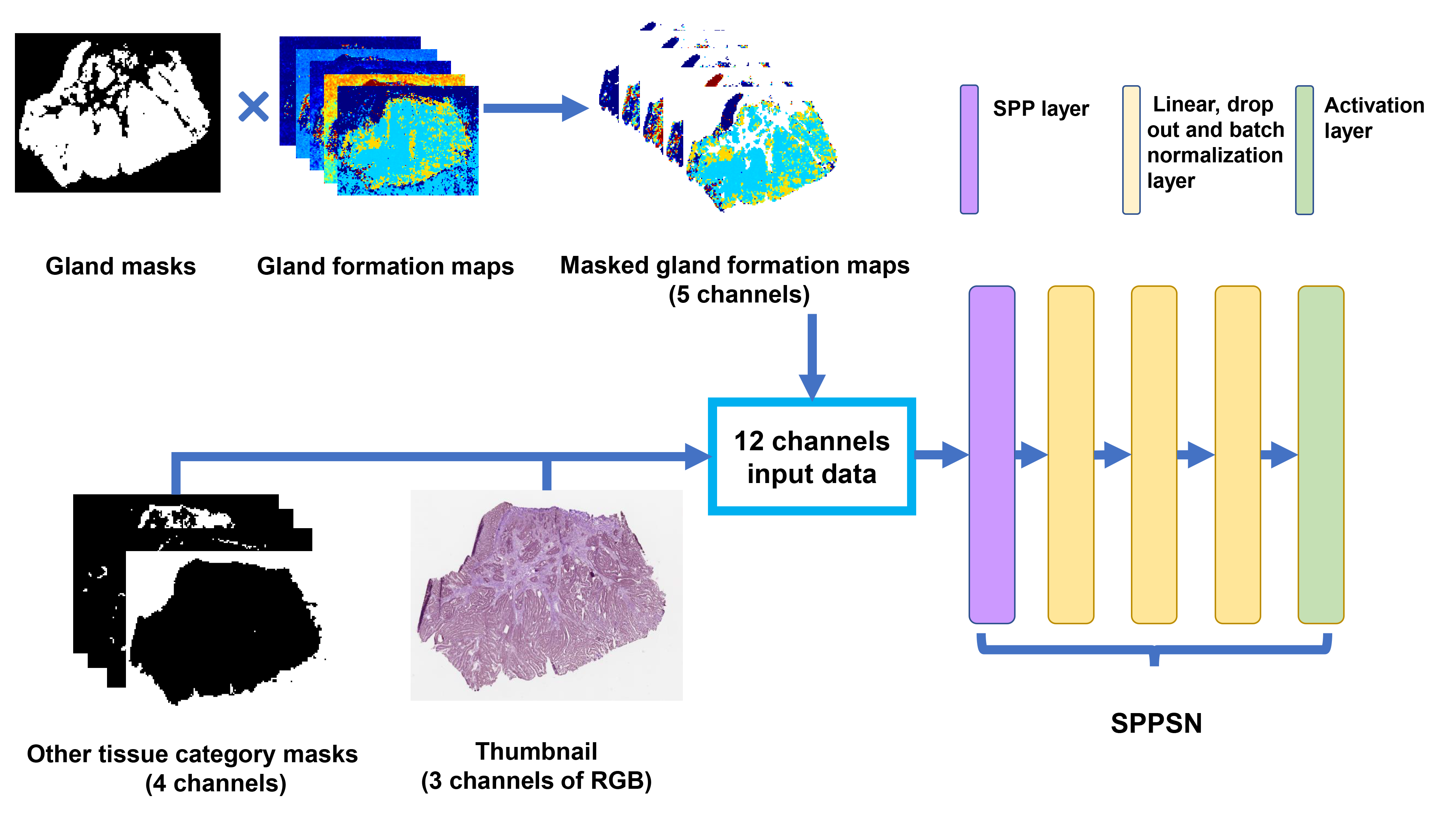
Fig1. (A) is the work flow of developing gland formation and tissue
category classifiers. (B) is the
work flow of calculating differentiation grade and deep survival grade.
Results
The training process was performed on TCGA
COAD dataset and TCGA READ dataset. The combination of COAD and READ dataset was
randomly split into training set (923 WSIs) and validation set (230 WSIs). A
local institutional dataset with 108 WSIs was enrolled as external test set. In
validation set and test set, c index of deep survival grade from SPPSN were
0.64 and 0.64, respectively. In Kaplan-Meier curves, WSIs were split into low
risk group and high risk group by median cut off point. The log rank test of Kaplan-Meier
curves (Fig2) was performed on validation set and test set, the p values were
both lower than 0.05.
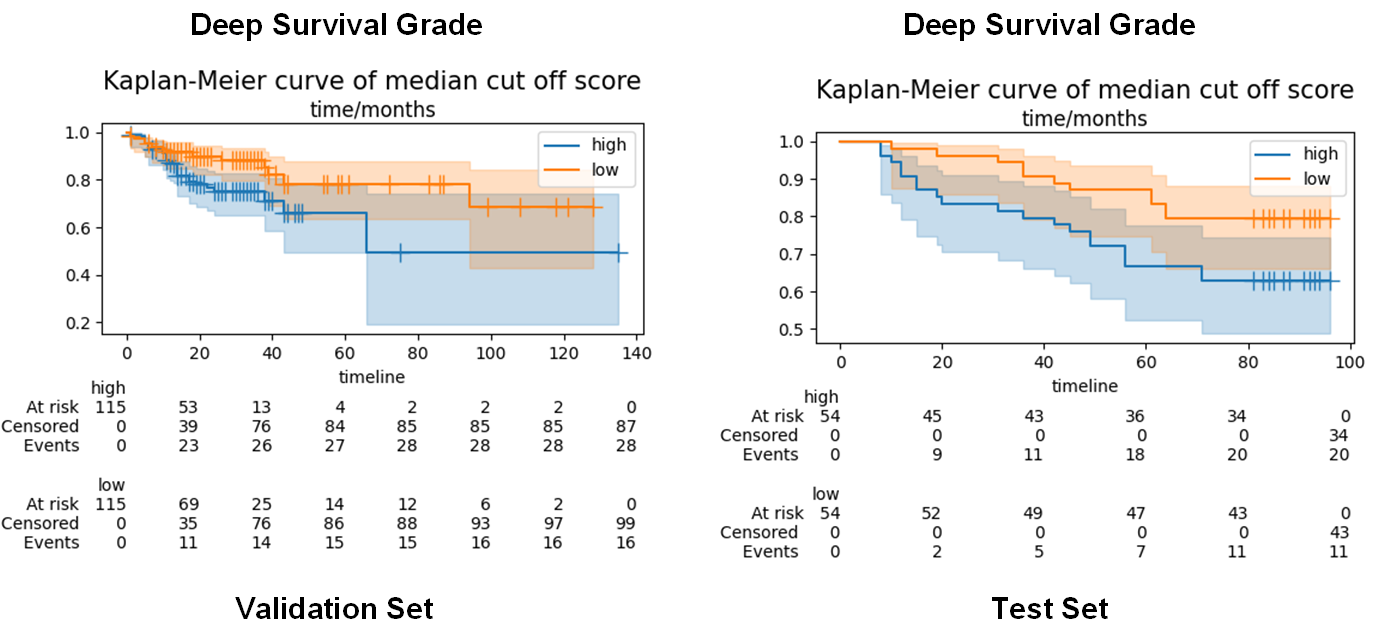
Fig2 Kaplan-Meier curves of SGFR and deep survival grade in validation
set and test set.
Conclusion
The SPPSN can successfully extract risk
information from WSIs for survival outcomes prediction. Our method provide a
new way to handle WSIs and create a new predictor which may potentially benefit
therapy decision making for colorectal cancer.