Towards Privacy-Preserving Federated Deep Learning infrastructure : proof-of-concept
Chong Zhang,
The Netherlands
PO-1116
Abstract
Towards Privacy-Preserving Federated Deep Learning infrastructure : proof-of-concept
Authors: Chong Zhang1, Ananaya Choudhury1, Inigo Bermejo1, Andre Dekker1
1Clinical Data Science, Maastro Clinic, GROW School for Oncology and Developmental Biology, Maastricht University Medical Centre+, Maastricht, The Netherlands
Show Affiliations
Hide Affiliations
Purpose or Objective
Deep learning (DL) has immense potential to revolutionise healthcare. Several Federated DL solutions have been proposed to access massive repositories of private data, without transferring subject data from the host devices. There remain concerns about potential privacy violation via “reconstructing” individually-identifiable subject data by exploiting model weights from host institutions. We propose a methodology for federated DL that addresses this risk through cloud-server architecture design.
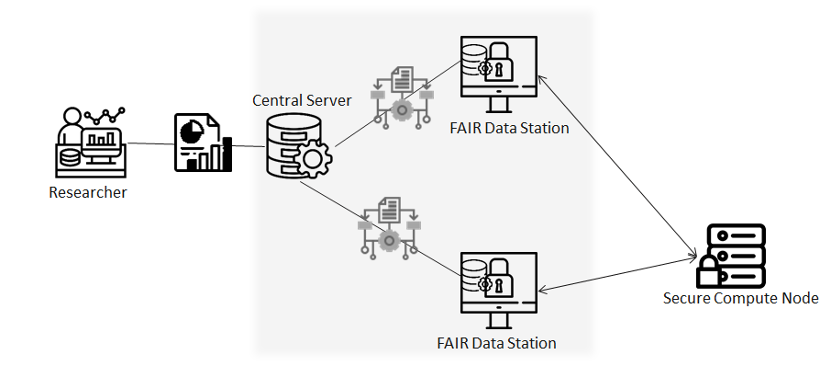
Experiments
| 1 epoch x 200 iterations
| 2 epoch x 100 iterations | 5 epoch x 40 iterations |
Training
| 0.76 (95% CI: 0.55-0.77)
| 0.70 (95% CI: 0.52- 0.75)
| 0.74 (95% CI: 0.57-0.82)
|
Validation
| 0.67 (95% CI: 0.54-0.71)
| 0.56 (95% CI: 0.51-0.68)
| 0.63 (95% CI: 0.56-0.73)
|
Material and Methods
The study is a fully federated replication of a previous distant metastasis classifier derived from 300 head-and-neck cancer patients. Two hospital cohorts were selected for training, and 2 others for validation. A “privacy-by-architecture” federated DL prototype was developed by extending the open-source distributed learning system VANTAGE6. An authenticated researcher submitted a task to a master server, that distributed a DL network to be trained by the data hosts. The DL model consisted of 3 fully-connected convolutional blocks. In each iteration of training, synchronous averaging and update of model weights were managed by a separate aggregation server connected to each data host via a key and encrypted web interfaces. The aggregation server prevented direct connections between hosts, researcher and master server during training. Once the model training was finished, final globally-averaged weights were stored on the master server for retrieval by the researcher. To find best combination of epoch and weights aggregation frequency, 3 experiments were conducted(1x200, 2x100, 4x50). Schematic diagram of the FL infrastructure is shown in Figure1.
Results
We demonstrated functional equivalence of a federated DL classifier to its centralized version, without transferring any subject-level data from data host machines. Furthermore, by preventing access to model weights, we added an additional layer of privacy protection. AUCs of 3 hyperparameter search experiments on training and validation data set are shown in Table 1. The centralized learning and federated learning models delivered statistically equivalent performance in terms of accuracy, loss and AUC. 5-fold cross validation mean AUC of centralized and federated DL models are 0.88 vs 0.85 and 0.88 vs 0.86 respectively.
Conclusion
We demonstrated a privacy-preserving federated DL methodology that enhances privacy protection by preventing visibility of model weights during training; globally-averaged model weights reduce the risk of privacy violation by " reconstruction" attacks on the data hosts. Our architecture may be further enhanced by adding differential privacy and encryption in the future. Besides, experiments with more centers should be conducted.