139 HNC patients with an 18F-FDG PET/CT scan acquired before
radiotherapy were included. The input data consisted of 11 clinical factors, 3 PET
parameters (SUVpeak, MTV, TLG) and 468 IBSI-listed radiomics
features extracted from the primary tumor volume in the PET and CT images. All
numeric features were preprocessed using z-score normalization. Three different
groups of input data were used to tune separate fully connected deep learning
architectures (Fig. 1a, Table 1 models M1-M3): Input data 1 (D1) 11 clinical
factors; Input data 2 (D2) 3 PET parameters, 60 1st order
statistical & shape features; Input data 3 (D3) 408 textural features. D2
and D3 were defined as radiomics features. A dropout layer, which randomly deactivated
25% of the nodes, was added to the end of each architecture to prevent
overfitting. The prediction targets DFS, LRC and OS were treated as binary
responses. Local or regional failure was counted as an LRC event, whereas DFS
also included metastatic disease or death as a DFS event.
Deep learning architectures designed separately for D1, D2
and D3 (Fig. 1a), were then concatenated in the second last layer, creating
four additional models (Table 1 M4-M7) with multiple input paths (Fig. 1b)
trained on input data D1 & D2, D1 & D3, D2 & D3 and D1 & D2 &
D3. Ensembles (Table 1 M8-M12, Fig. 1c) based on the mean predicted class probability
of models M1-M3 and M6 were also evaluated.
All models were trained using five-fold cross-validation, where
the folds were stratified to conserve the proportion of stage I+II vs. III+IV patients
(8th edition AJCC/UICC) in the full dataset. The Area Under the
Receiver Operating Characteristic Curve (ROC-AUC) was used to evaluate model
performance.
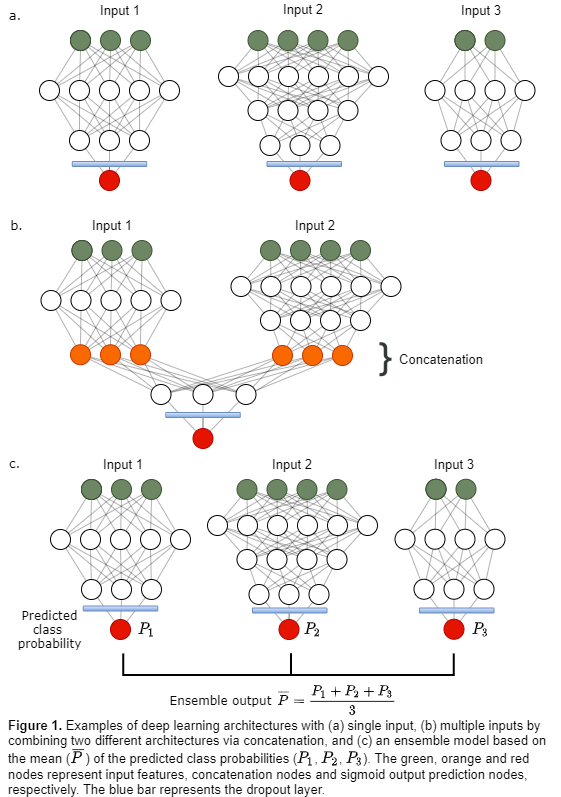