A data mining-based approach to predict the growth of brain structures after pediatric radiotherapy
PD-0070
Abstract
A data mining-based approach to predict the growth of brain structures after pediatric radiotherapy
Authors: Renata Georgia Raidou1, Ludvig P. Muren2, Laura V. Toussaint3, Danny Indelicato4, Caroline Magg1
1TU Wien, Institute of Visual Computing & Human-Centered Technology, Vienna, Austria; 2Danish Centre for Particle Therapy, Department of Medical Physics, Aarhus, Denmark; 3Danish Centre for Particle Therapy, Department of Medical Physics, Department of Medical Physics, Aarhus, Denmark; 4University of Florida, Department of Radiation Oncology, Gainesville, FL, USA
Show Affiliations
Hide Affiliations
Purpose or Objective
Radiotherapy (RT) to children with brain tumors influences the growth and cognitive development of nearby structures. Studies relating longitudinal brain changes to the doses delivered to critical structures require re-segmentation. The aim of this study was to develop a data mining-based method to predict the growth of structures in pediatric brain tumor patients treated with RT.
Material and Methods
Co-registered Computed Tomography (CT) pre-treatment scans and 1-3 post-treatment Magnetic Resonance (MR) scans from 20 patients with brain tumors treated with RT were used. A total of 21 brain structures were selected based on potential association to cognitive function. Our approach consisted of three steps (Fig.1): (i) predicting segmentations of structures post-treatment based on pre-treatment data; (ii) quantifying the prediction accuracy; and (iii) visualizing structure evolution and its accuracy. In the first step, pre-treatment masks were used to predict post-treatment masks on MR images. For this, an active contour model was initialized with a dilated version of the pre-treatment masks. The parameters of the contour model and the selected dilation kernel might influence the prediction, and thus a two-step hyperparameter search was used to determine their optimal values by quantifying the divergence from ground truth. In the second step, a support vector regressor (SVR) with 35 features (related to geometry, intensity, and gradients) was trained on the pre-treatment data and ground truth information (Fig.2). The accuracy of post-treatment predictions was quantified using the Jaccard index (taking values from 0 to 1, with 1 indicating complete similarity). In the third step, a distance transform operation was employed to calculate the distances between pre-and post-treatment data. This was used to visualize the predicted growth of a structure and its respective accuracy.
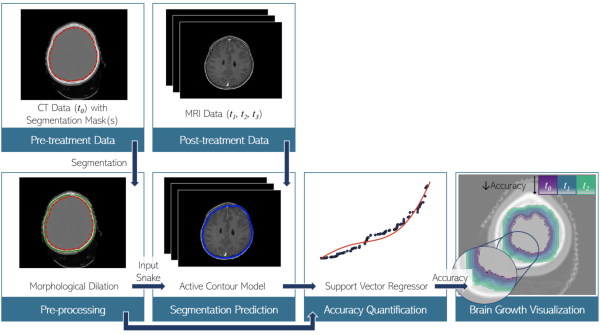
Fig.1: The proposed approach.
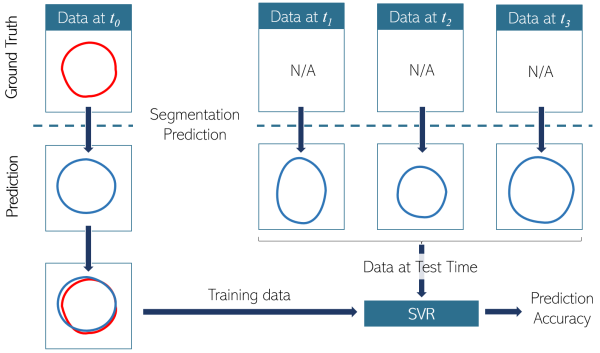
Fig.2: SVR Training.
Results
Three parameters (dilation kernel size, smoothness, and maximal number of iterations) influenced the outcome of the segmentation the most. Segmentations of larger structures were more accurately predicted, but overall the prediction accuracy behavior was learned correctly for all structures. The Jaccard index was higher for large structures, such as the brain (0.81) and temporal lobe (0.74), as compared to smaller structures, such as the thalamus anterior (0.09) and hypothalamus (0.11). The support vector regression predicted the Jaccard distance with a mean squared error between 0.03-0.08, i.e., the behavior observed in the training dataset was learned correctly. The visualization showed the predicted growth patterns and their accuracy.
Conclusion
We have developed a pipeline to predict segmentations for post-treatment data based on pre-treatment scans, including a method to evaluate the prediction accuracy. The method provided good results, especially for larger brain structures, while the prediction accuracy behavior of all structures was learned correctly.