Deep learning auto-segmentation vs human inter observer variability of normal tissue in the brain
Jesper Kallehauge,
Denmark
MO-0217
Abstract
Deep learning auto-segmentation vs human inter observer variability of normal tissue in the brain
Authors: Jesper Kallehauge1, Camilla Skinnerup Byskov2, Christian Rønn Hansen3, Yasmin Lassen-Ramshad1, Anouk Trip1, Slavka Lukacova2, Lene Haldbo-Classen2, Ebbe Laugaard Lorenzen4
1Aarhus University Hospital, Danish Centre for Particle Therapy, Aarhus N, Denmark; 2Aarhus University Hospital, Department of Oncology, Aarhus N, Denmark; 3Odense University Hospital, Laboratory of Radiation Physics, Odense, Denmark; 4Odense University Hospital, Laboratory of Radiation Physics, Odense, Denmark
Show Affiliations
Hide Affiliations
Purpose or Objective
Automatic
segmentations of organs at risk (OAR) are often compared to single observer
delineations, which especially for small organs may result in higher
inter-observer disagreement. A more fair comparison is to validate the auto-segmentation
to a dataset where multiple observers have defined the same structures. Hence
the objective of this study was to validate a Deep Learning semantic
segmentation of selected OAR according to the Danish Neuro Oncology (DNOG)
guidelines on multi-observer gold standard segmentations.
Material and Methods
A
3D U-Net architecture was trained to segment Brainstem, Hippocampi, Chiasm,
Pituitary, and Optical Tracts on 58 glioma patients' 3D T1w MRI of the brain
(46 patients were scanned on a 3 T MRI and 12 were scanned at 1.5 T). This
network was subsequently evaluated on a holdout test set consisting of 13
patients (nine patients were scanned at 3T MRI and four were scanned at 1.5T
MRI). The performance of the automatic segmentation was finally evaluated using
Dice similarity
coefficient (DSC) and 95% Hausdorff distance (HD95) on
three randomly chosen patients from the gold standard data having 13 patients
in total. Each structure for each of the three patients were defined on average
by 8 radiation oncologists with experience in neuro-oncology. The automatic
segmentation was compared to each of the 8 expert segmentations and the mean metric
HD95 and DSC was determined and compared to the mean expert metrics.
Results
The
auto-segmented structures performed similar to expert delineated structures in
respect to DSC and HD95. (figure 1 and figure 2). Although, the optical tracts
appear to perform slightly worse when comparing DSC. The auto-segmentation
showed similar or reduced variability especially for the pituitary gland but
generally across all the investigated structures. Few expert segmentations were
identified with large deviations from the median metrics indicative of being
outliers. Especially some
segmentations with HD95 well beyond the interquartile range are suspicious. 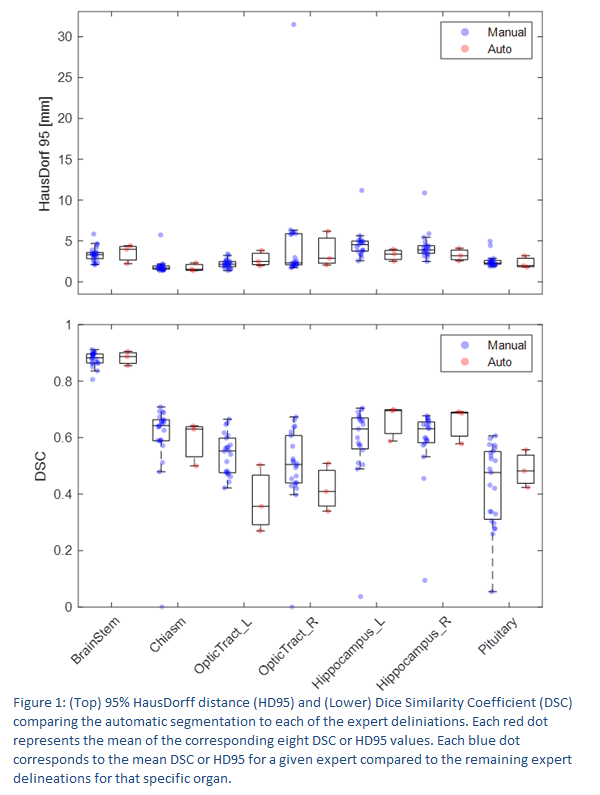
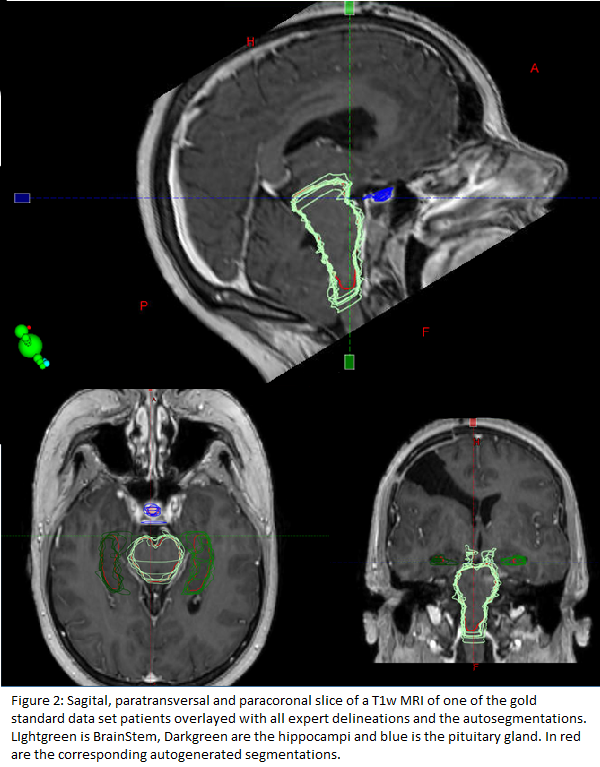
Conclusion
The
proposed Deep Learning segmentation algorithm performed generally very well
showing similar performance as the experts for all structures except the
optical tracts when comparing DSCs. This
discrepancy may be attributed to the small volume of these structures where the
DSC is known to be especially sensitive to small variations in delineations. The expert segmentations with large deviation
from the general trend of the expert segmentations (HD95 well beyond the interquartile range)
were
visually inspected and was found not to adhere to the DNOG guidelines. The auto
segmentations were on the other hand in good agreements with the general expert
trend and a potential added benefit of auto segmentation could be outlier
detection of subpar segmentations for example in retrospective datasets. To
conclude, this deep learning network is of such high quality as to
be used in clinical workflow and shows potential as a tool for delineation
outlier detection.