Generalizability of deep-learning-based CBCT image enhancement with respect to anti-scatter grids.
Xander Staal,
The Netherlands
OC-0774
Abstract
Generalizability of deep-learning-based CBCT image enhancement with respect to anti-scatter grids.
Authors: Xander Staal1, Jan-Jakob Sonke1
1Netherlands Cancer Institute, Radiation Oncology, Amsterdam, The Netherlands
Show Affiliations
Hide Affiliations
Purpose or Objective
In
recent years, the neural network (NN) has emerged as a promising tool to
enhance Cone Beam Computed Tomography (CBCT) images. Among them, NNs based on
the cycle consistent generative adversarial network (CycleGAN) architecture
have been shown to suppress imaging artifacts in CBCT images. Such a NN is
applied to a CBCT image, resulting in a synthetic CT (sCT), with processing
times of a few seconds. These sCTs should allow accurate dose calculations and
(re-)contouring, which is an important step toward online adaptive radiotherapy
with conventional linacs.
A common concern with NNs is generalizability.
When a trained NN is deployed, it is hard to predict how it will perform on
out-of-distribution data, e.g. acquired in a different institute or with a different
machine. This study quantifies the performance of a NN, trained on CBCTs acquired
on machines without anti-scatter grid (ASG), when applied to CBCTs acquired on machines
with ASG.
Material and Methods
The
NN was a pretrained, CycleGAN-based model, deployed in the Advanced Medical
Image Registration Engine (ADMIRE, Elekta) v3.34. It was trained on CBCTs of
the male pelvis region, acquired without ASG, downsampled to 1.64x1.64mm in-plane voxel size. The testing dataset contained 895 CBCTs
(Elekta Versa HD) from 47 prostate cancer patients, each with a planning CT (Siemens
Somatom go.Open Pro). Of these CBCTs, 336 and 559 were acquired with and without
ASG respectively.
For each CBCT, a sCT was generated by
the NN and two reference CTs were generated by deformably registering the
planning CT to the CBCT and sCT respectively. The CBCT and sCT were compared to
their reference CT with the structural similarity (SSIM) index, mutual
information (MI), mean error (ME), mean absolute error (MAE) and the absolute
percentage difference between their histograms (histogram error). The sharpness
of the images was evaluated as the full width at half maximum (FWHM) value of
the compact bone of the pelvis.
Statistical significance of the results was
evaluated using the Wilcoxon rank-sum test for clustered data.
Results
Examples of CBCTs, sCTs and a planning
CT are shown in figure 1. Calculated metrics are summarized in table 1.
The sCTs outperformed their
corresponding CBCTs on all metrics, except FWHM (P<.001 for all metrics). This shows that the NN improves correspondence
with the reference CT, but loses some sharpness with respect to the CBCT.
The CBCTs with ASG outperformed the CBCTs
without, except in FWHM (P<.05
for all metrics). The sCTs with ASG outperformed the sCTs without, except in
FWHM (P<.05 for all metrics
except MAE).
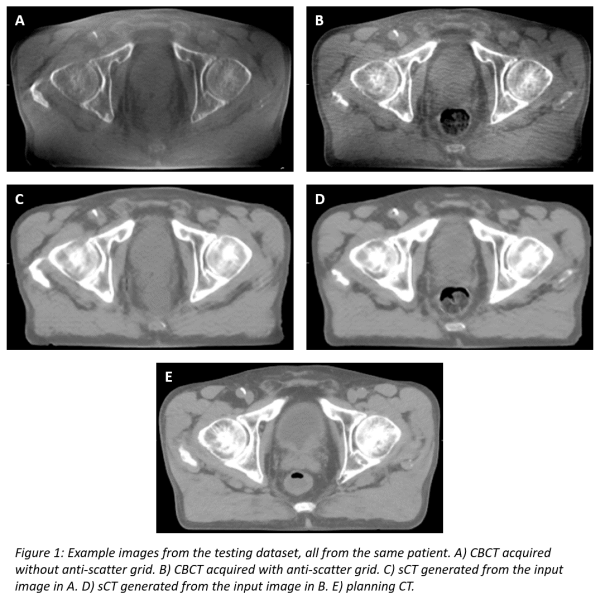
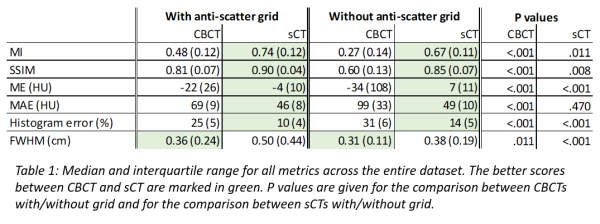
Conclusion
The NN in Admire reliably enhanced the
CBCT images. We observed some loss of sharpness, which may be explained by the downsampling
of the training data. The NN benefitted from the higher quality in CBCTs with ASG,
even though it was trained exclusively on data without ASG. We expect, however,
that the differences between sCTs with/without ASG may not be dosimetrically relevant.