Auto-segmentation of low contrast organs at risk improves with minimal prior delineation input
Mathis Ersted Rasmussen,
Denmark
OC-0769
Abstract
Auto-segmentation of low contrast organs at risk improves with minimal prior delineation input
Authors: Mathis Ersted Rasmussen1,2,3,4, Jasper Albertus Nijkamp1,4, Jesper Grau Eriksen2, Stine Sofia Korreman1,3,4
1Aarhus University Hospital, Danish Center for Particle Therapy, Aarhus, Denmark; 2Aarhus University Hospital, Department of Experimental Clinical Oncology, Aarhus, Denmark; 3Aarhus University Hospital, Department of Oncology, Aarhus, Denmark; 4Aarhus University, Department of Clinical Medicine, Aarhus, Denmark
Show Affiliations
Hide Affiliations
Purpose or Objective
Deep learning auto-segmentation of organs-at-risk (OAR) in head-neck cancer performs well for structures with high visual contrast such as parotid glands, mandibles, spinal cord and brain. However, OARs such as glottic larynx and pharyngeal constrictor muscles (PCMs) can have low or no visual contrast which results in inaccurate auto-segmentation. We aim to improve segmentation performance of these challenging OARs by including minimal manual delineations as input to deep learning auto-segmentation models.
Material and Methods
We used a data set with planning CTs and manual delineations of OARs from 301 patients previously treated with radiotherapy for head-neck cancer at our institution. 30 cases were randomly selected for an external test set, and thus 271 cases were eligible for training. Our training data did not contain all OARs for all patients. Hence, models were trained and tested only on patients with the OARs of interest present (Table 1).
To simulate minimal manual delineation (MMD) input, we extracted the most cranial and caudal slice of each OAR from our data set and input these along with CT in 3D full resolution nnUNets [Isensee, F et al. 2020] (CT+MMD). We included lower, middle and upper PCM, glottic larynx and parotid glands. For reference we also trained nnUNets without MMD (CT-only). All models consisted of a single fold (1000 epochs) nnUNet with default parameters. Parotid glands were trained and evaluated as one OAR to avoid segmentation of the contra lateral gland.
We obtained Dice-Sørensen Coefficient (DSC) and Hausdorff distance 95th percentile (HD95) on the external test set with nnUNet’s build-in evaluation function. Test for similarity between the paired models for each OAR was done with a two-sided Wilcoxon signed-rank test with a confidence level of 5 %.
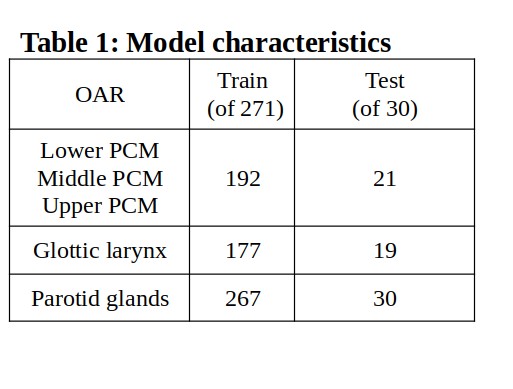
Results
DSC and HD95 were significantly better for all OARs with CT+MMD compared to CT-only (p ≤ 0.001, figure 1). Low and no contrast OARs (PCMs and glottic larynx) benefited most, whereas the effect was marginal for the high contrast OAR (parotid glands). Increases in median DSC were 0.56 to 0.86 for glottic larynx, 0.64 to 0.83 for lower PCM, 0.65 to 0.83 for the middle PCM, 0.61 to 0.78 for upper PCM and 0.83 to 0.86 for parotid glands.
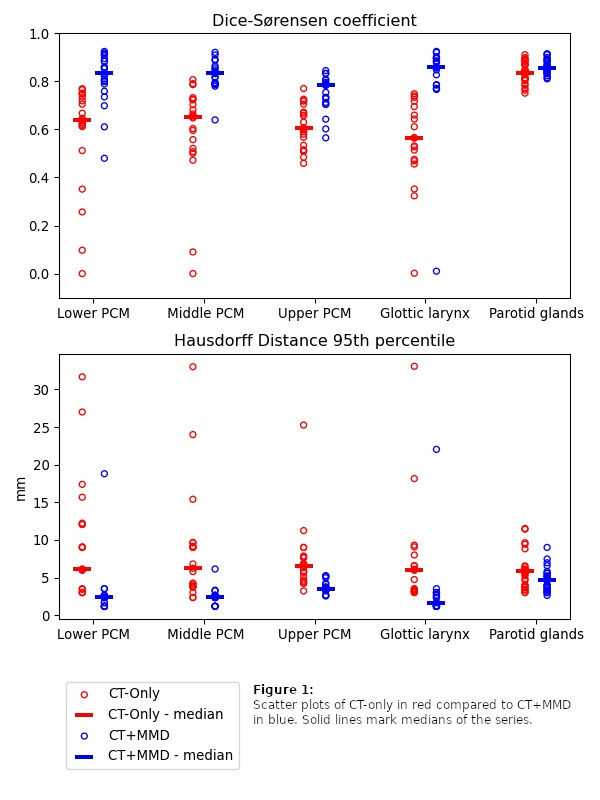
Conclusion
By training deep learning auto-segmentation models on CT along with just two manually delineated slices per OAR, we demonstrate major improvements of segmentation metrics for low and no contrast OARs such as PCMs and glottic larynx compared to CT-only models. For the included high contrast OAR (parotid glands) CT-only performed well, and no substantial improvements were observed with CT+MMD.
Our findings show that it may be beneficial for clinicians to manually delineate a few selected slices of low and no contrast OARs prior to running deep learning auto-segmentation models, as the subsequent predictions are likely to require much less revision than for predictions of fully automated models.