Robust scheduling for a One Stop Shop palliative radiotherapy clinic using genetic algorithms
Nienke Hoffmans-Holtzer,
The Netherlands
PO-1040
Abstract
Robust scheduling for a One Stop Shop palliative radiotherapy clinic using genetic algorithms
Authors: Nienke Hoffmans-Holtzer1, Luuk Smolenaers2, René Peeters2, Nathalie Swart1, Olijn Tims1, Ilse De Pree1, Cleo Slagter1, Manouk Olofsen - van Acht1, Mischa Hoogeman1, Marleen Balvert2, Steven Petit1
1Erasmus MC, Department of Radiotherapy, Rotterdam, The Netherlands; 2Tilburg School of Economics and Management, Department of Econometrics and Operations Research, Tilburg, The Netherlands
Show Affiliations
Hide Affiliations
Purpose or Objective
At our dedicated
one-stop-shop (OSS) outpatient clinic for palliative RT, each day 4 patients are
scheduled from intake to treatment in one working day. Patients arrive in the
morning and are treated at the end of
the day, spending roughly 6 hours from start of intake to start of treatment
(SIST). It is expected that by optimizing the sequence of the 12 preparation
steps per patient (48 in total, in combination referred to a schedule) the average
SIST (aSIST) over the 4 patients could be reduced considerably. However,
minimizing the aSIST increases the vulnerability of the process for unexpected
delays and therefore risk of overtime (RoO). Hence our goal was to develop a
method to automatically optimize schedules to balance aSIST and RoO.
Material and Methods
The OSS is
ran by 1 dedicated RO and 2 all-round RTTs. The mean execution times of the 12 different
steps were extracted for 663 patients treated between October 2019 and October 2020
(Table 1). A Kolmogorov-Smirnov test determined execution time distributions for
the 12 steps, which were randomly sampled 250 times for the 48 steps.
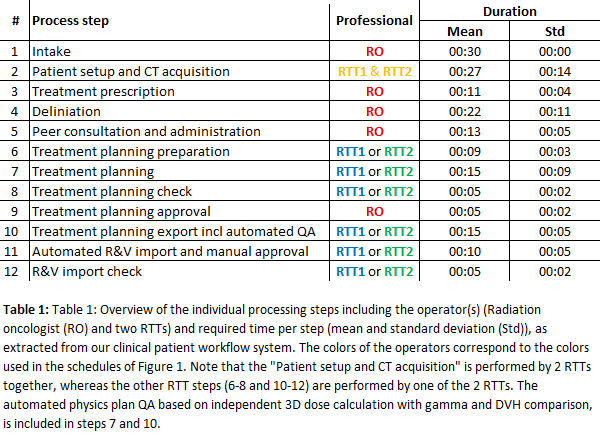
First, 200 schedules
were generated at random (1st generation) as input for a non-dominated
sorting genetic algorithm (NSGA-II). For each schedule a linear program found the
optimal starting time of each step, lunch time and optimal operator by minimizing
the expected aSIST. To calculate RoO, each schedule was evaluated 250 times for
pre-sampled execution times. From the 1st generation, the 100 most
promising parents were selected based on tradeoff fronts between expected aSIST
and RoO. Next, the NSGA-II algorithm created 100 offspring (i.e. novel
schedules) by random point cross-over and mutation (1-5 pairs, randomly picked)
and the expected aSIST and RoO were calculated. The 100 parents and their 100 offspring
formed the next generation. The NSGA-II was run for 2000 generations. The expected
aSIST and RoO were also calculated for the current clinical schedule.
Results
Figure 1
shows the tradeoff front between expected aSIST and RoO and how it converges
with increasing generations. The expected aSIST was smallest (189 min) for Schedule
1 but would lead to 100% RoO. Decreasing RoO to 5% could be achieved but at large
increase in expected aSIST of 103 min (Schedule 3). Schedule 2 represented an acceptable
balance between RoO of 14% with expected aSIST of 242 min (53 min more than
schedule 1). Note that schedule 2 outperformed the clinical schedule (RoO and
aSIST) both in RoO and aSIST.
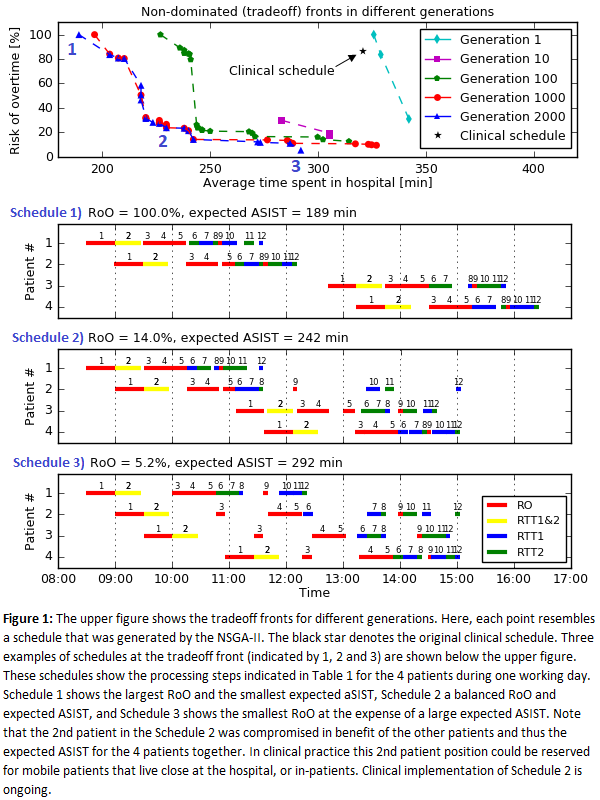
Conclusion
Robust optimization for scheduling indicates that large reductions in patients’
occupation time can be achieved in our one-stop-shop out-patient clinic. The tradeoff
front allowed selection of the schedule that balanced best the average throughput
time and the risk of overtime (Schedule 2). Schedule 2 is currently being
implemented clinically. These results strongly support further exploration of
scheduling optimization for RT preparation also outside a one-stop-shop
setting.