Cranial irradiation leads to nearly 3x accelerated biological aging in glioma patients
Selena Huisman,
The Netherlands
OC-0456
Abstract
Cranial irradiation leads to nearly 3x accelerated biological aging in glioma patients
Authors: Selena Huisman1, Fia Cialdella1, Arthur van der Boog1, Joost Verhoeff1, Szabolcs David1
1UMC Utrecht, Radiation Oncology, Utrecht, The Netherlands
Show Affiliations
Hide Affiliations
Purpose or Objective
Post-radiation
morphological changes in the brain have been investigated, but remain challenging
to quantify. Observed changes, such as tissue atrophy, have often been compared
to chronological age and could be linked to cognitive decline. This study aims
to provide an interpretable score to quantify post-radiation changes in the
brain using the framework “brain age gap estimation (BrainAGE)”, which is free of any pre-engineered features, like cortical thickness or regional volumes (e.g.:
hippocampus). BrainAGE utilizes deep learning models trained on healthy MRI
scans to predict the biological age for patients. By obtaining ages for
multiple longitudinal scans, the rate of change within the brain can be
quantified with an interpretable score, represented by an aging rate.
Material and Methods
129 longitudinal
MRI scans that have been collected from 32 glioma patients who received radiotherapy at UMC Utrecht were
analyzed retrospectively using a neural network-based deep learning model for
brain age prediction. This model was pre-trained on 14503 healthy MRI scans
from the UK Biobank. An age was predicted for each MRI scan, which was fed into
a linear mixed effects model to predict aging rates for every patient. Saliency
maps were extracted from the model and averaged from the whole study population
in order to provide insight into which parts of the brain contribute the most
to the estimated brain age. Hotspots from the saliency maps were quantified via
existing MNI space-available brain atlases, like the Oxford-Harvard cortical
and subcortical atlas.
Results
Fig. 1/A shows
an example of a 42-year-old female patient with an increase in the predicted
brain age as time progresses, indicating radiation-induced accelerated aging.
Furthermore, Fig. 1/B shows the population average saliency map, indicating
which areas have relatively high contribution in estimating the biological age
according to the neural network. Among the most contributing regions, numerous
anatomically well-defined areas can be found such as the Heschl’s gyrus and Middle
Cerebellar Peduncle. Fig. 2 shows the predicted aging rate for each individual
patient and the group aging rate in red. The full cohort of patients show the
mean aging rate of 2.78 years per year (95% CI = 2.54-3.02), significantly
higher than the normal aging rate of 1 (p = 6.72e-16).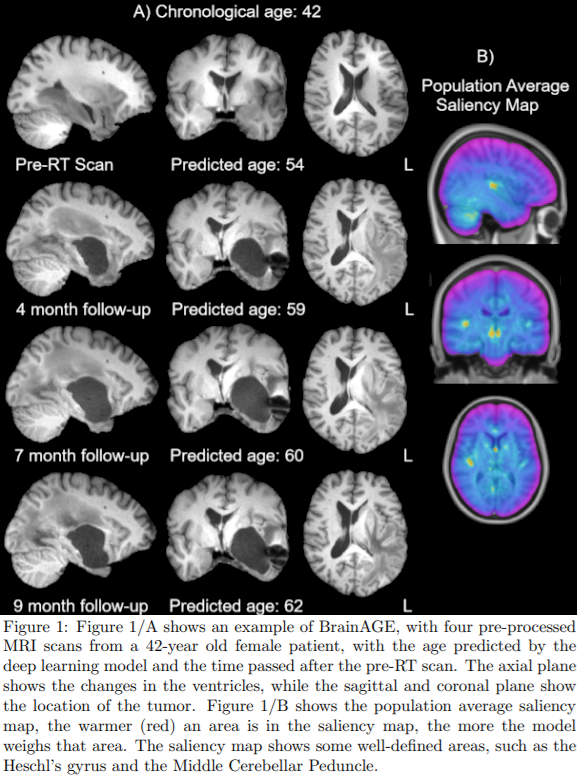
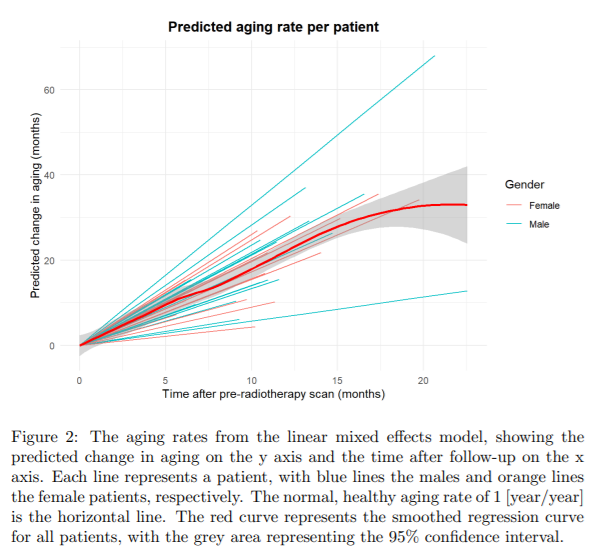
Conclusion
The BrainAGE
framework shows a significantly higher rate of biological aging for glioma
patients after radiotherapy. Due to the lack of pre-engineered features,
BrainAGE can provide an overview of the spatial post-radiation changes in the
brain, taking the entire brain volume into account. The well-defined areas obtained
from the saliency maps could indicate importance for future research into post-radiotherapy changes in the brain. Applying this framework provides further insights into radiation-induced
accelerated aging. Use of BrainAGE may
eventually guide radiation therapy planning and contribute to expectation
management of treatment side effects.