An explainable deep learning pipeline for multi-modal multi-organ medical image segmentation
PD-0314
Abstract
An explainable deep learning pipeline for multi-modal multi-organ medical image segmentation
Authors: Eugenia Mylona1, Dimitris Zaridis1, Grigoris Grigoriadis2, Nikolaos Tachos3, Dimitrios I. Fotiadis1
1University of Ioannina, Department of Biomedical Research, FORTH-IMBB, Ioannina, Greece; 2University of Ioannina, Unit of Medical Technology and Intelligent Information Systems, Materials Science and Engineering Department, Ioannina, Greece; 3University of Ioannia, Department of Biomedical Research, FORTH-IMBB, Ioannina, Greece
Show Affiliations
Hide Affiliations
Purpose or Objective
Accurate image segmentation is the
cornerstone of medical image analysis for cancer diagnosis, monitoring, and
treatment. In the field of radiation therapy, Deep Learning (DL) has emerged as
the state-of-the-art method for automatic organ delineation, decreasing workload, and improving plan quality and consistency. However, the lack of knowledge and
interpretation of DL models can hold back their full deployment into clinical routine
practice. The aim of the study is to
develop a robust and explainable DL-based segmentation pipeline, generalizable
in different image acquisition techniques and clinical scenarios.
Material and Methods
The following clinical scenarios were investigated: (i)
segmentation of the prostate gland from T2-weighted MRI of 60 patients (543
frames), (ii) segmentation of the left ventricle of the heart from CT images of
11 patients (1856 frames), and (ii) segmentation of the adventitia and lumen areas of the coronary artery from
intravascular ultrasound images (IVUS) of 42 patients (4197 frames). The workflow of the proposed DL segmentation network is
shown in Figure 1. It is inspired by the state-of-the-art ResUnet++ algorithm
with the difference that (i) no residual connections have been used and (ii)
the addition of a squeeze and excitation module to extract interchannel
information for identifying robust features. The model was trained and tested
separately for each clinical scenario in 5-fold cross-validation. The
segmentation performance was assessed using the Dice Score (DSC) and the Rand
Error index. Finally, the Grad-CAM technique was used to generate heatmaps of
feature (pixel) importance for the segmentation outcome. This is an indicator
of model uncertainty that reflects the segmentation ambiguities, allowing to
interpret the output of the model.
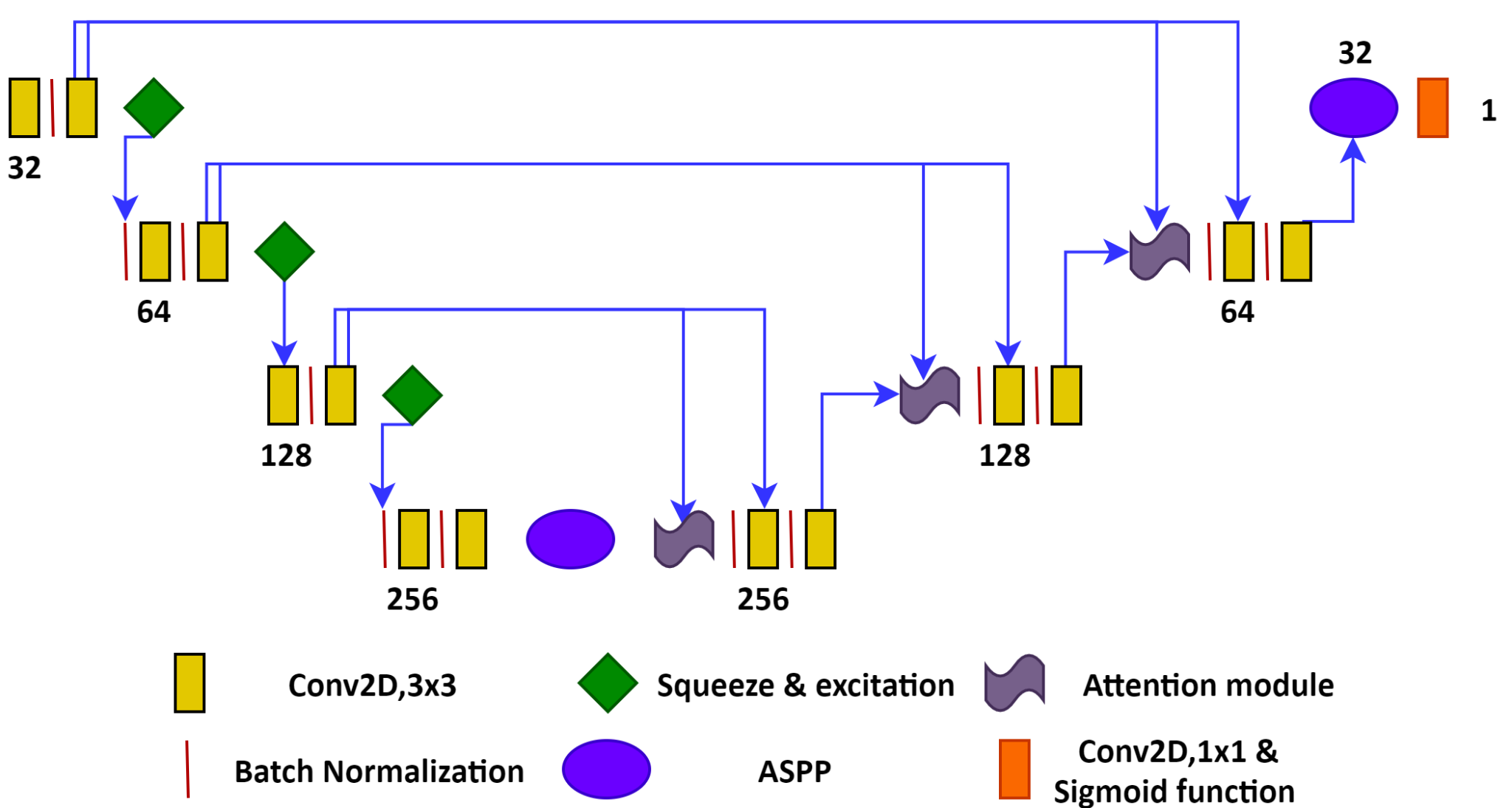
Results
The DSC for the prostate gland was 84%
± 3%, for the heart’s left ventricle it was 85% ± 2.5%, for the adventitia it
was 85%±1%, and for the lumen 90% ± 2%. The average rand error index for all
cases was less than 0.2. An example of the model performance for each clinical
scenario is shown in Figure 2, including (A) the original image with the ground
truth (orange) and the predicted (blue) contours, (B) the manual (ground truth)
annotation masks from experts, (C) the segmentation mask derived from the model
and (D) the heatmaps indicating how important are the
pixels in the image that contribute to the segmentation result.
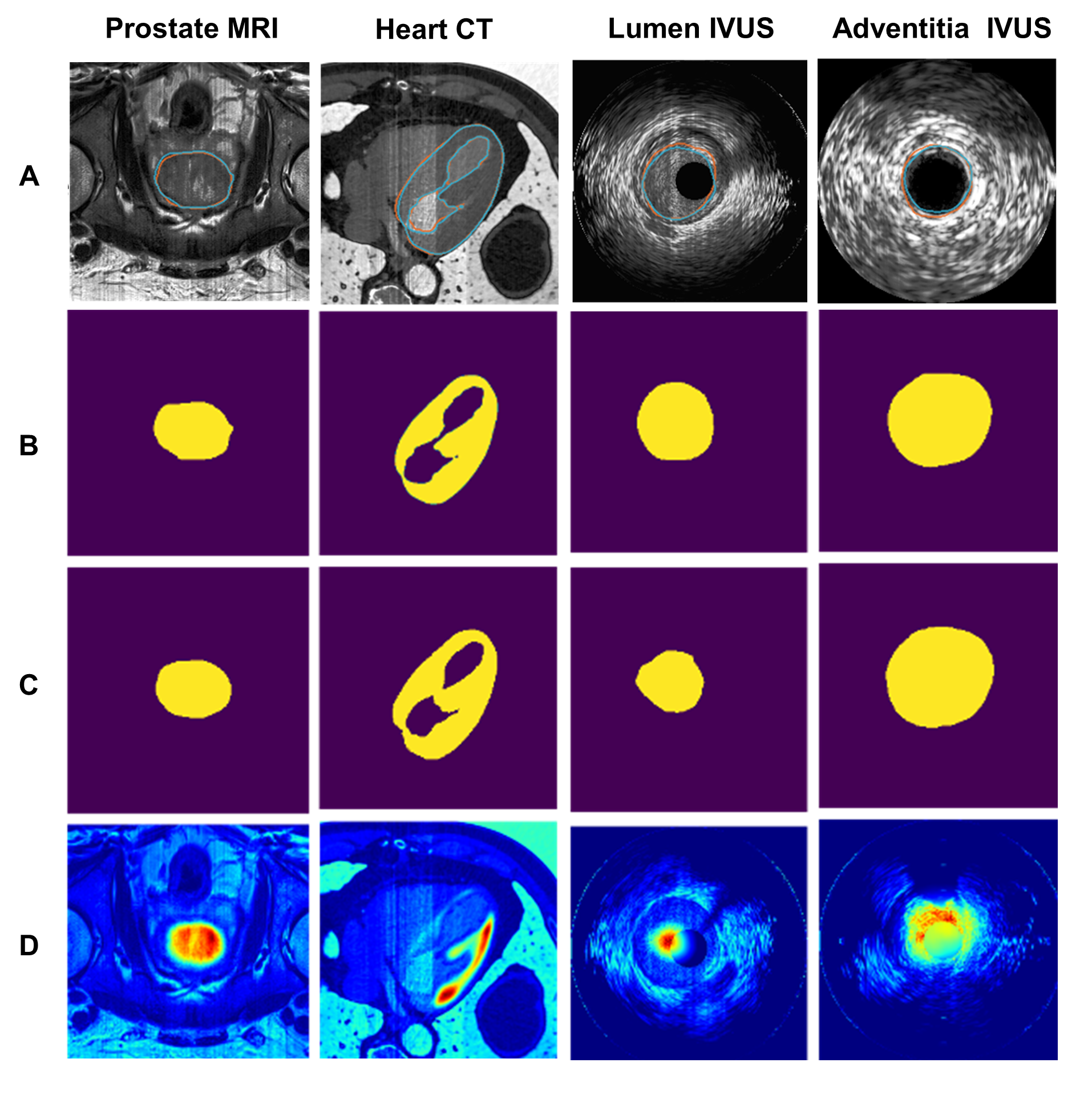
Conclusion
A generic DL-based segmentation
architecture is proposed with state-of-the-art performance. A module for model
explainability was introduced aiming to improve the consistency and efficiency
of the segmentation process by providing qualitative information on the model’s
predictions, thereby promoting clinical acceptability. In the future, incorporating
explainability measures in the entire treatment planning workflow, such as
registration and dose prediction, will lead to a potential improvement in
clinical practice and patient treatment.