autoencoder-based quality assurance of deep learning segmentation of parotid glands in HNC patients
Alessia De Biase,
The Netherlands
PO-1656
Abstract
autoencoder-based quality assurance of deep learning segmentation of parotid glands in HNC patients
Authors: Sytze Wolter Zijlstra1, Alessia de Biase2,3, Charlotte Brouwer2, Stefan Both2, Johannes Langendijk2, Peter van Ooijen2
1University of Twente, Faculty of Science and Technology, Enschede, The Netherlands; 2University Medical Centre Groningen (UMCG), Department of Radiotherapy, Groningen, The Netherlands; 3University Medical Centre Groningen (UMCG), Data Science Centre in Health (DASH), Groningen, The Netherlands
Show Affiliations
Hide Affiliations
Purpose or Objective
In radiotherapy, the pre-treatment planning of head and neck cancer (HNC) patients includes the segmentation of organs at risk (OARs) as observed on Computed Tomography (CT) images. In our clinical practice, Deep Learning Contours (DLCs) of OARs are generated by commercial DL algorithms which require a quality assurance and, in some cases, manual adjustments performed by the clinicians. This subjective and time consuming process explains the demand for an automated and objective contour quality assessor. In this study we investigated the feasibility of a convolutional autoencoder (AE) for this task, which was trained on approved, manually adjusted contours (MACs) and tested on the DLCs. The aim of this study was to test the hypothesis that the AE error in reconstructing the DLC correlates well with the experts’ manual adjustments.
Material and Methods
The right parotid gland of 101 HNC patients from our institute was used. CT images were acquired using the same CT scanner and imaging protocol. DLCs and MACs reviewed by different observers were collected. The training and validation sets consisted of 61 and 20 patients. The test set included 20 cases. The AE was trained for 1,000 epochs, with a batch of 2 and the mean squared error (MSE) loss function. The input of the network was of two channels corresponding to CT and contour data. Firstly, the DL framework was trained and validated using MAC data as contour data. Secondly, the best performing model was evaluated on CT and DLC data from the training, validation and test sets. The manual adjustments were quantified by computing the MSE of the difference between the MACs and original DLCs. By considering the approved MACs as the ground truth, the DLC reconstruction error was hypothesised to be a measure of DLC quality. The reconstruction errors were finally correlated with the manual adjustments. To investigate the influence of the interobserver variability, the results were compared among the three RTTs responsible for the MACs.
Results
The AE showed comparable performance in reconstructing both MAC and DLC (see Table 1) and it was able to distinguish a gradient of good to bad DLCs. A moderate correlation was observed between the adjustments and the reconstruction errors for both DLC (0.5) (see Figure 1) and MAC (0.7) data in the test set, suggesting that the correlation can be primarily attributed to their shared CT input. The presence of outliers in the limited of the test set heavily affected the results. Interobserver variance did not seem to play a role in the reconstruction quality.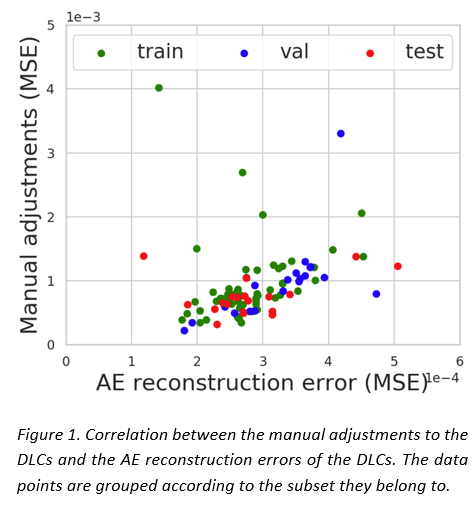
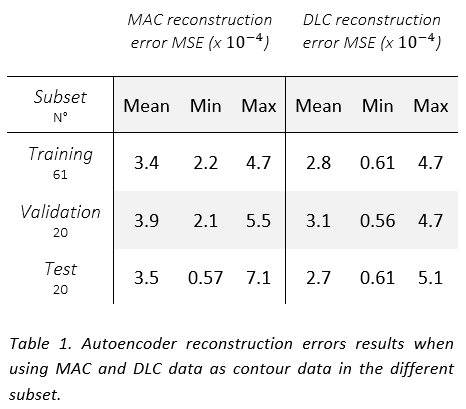
Conclusion
A significant but moderate correlation was observed between the AE reconstruction errors on the DLC and the manual adjustments to DLC performed by experts. This encourages future research including more data and organs to quantify trends. If a range of acceptable manual adjustments to the DLC could be defined, threshold values for the AE reconstruction errors could be of benefit to classify acceptable from unacceptable DLCs.