Deep Learning-Based Automatic Segmentation for Brain OARs: Accuracy and Dosimetric Impact
PO-1648
Abstract
Deep Learning-Based Automatic Segmentation for Brain OARs: Accuracy and Dosimetric Impact
Authors: Andrada Turcas1,2,3,4, Cristina Gheara2,5, Daniel Leucuta6, Cristina Balan2,7, Cernea Dana2
1University of Medicine and Pharmacy and Medicine "Iuliu Hatieganu", Oncology Department, Cluj-Napoca, Romania; 2Oncology Institute "Prof. Dr. Ion Chiricuta", Radiotherapy Department, Cluj-Napoca, Romania; 3SIOP Europe – The European Society for Paediatric Oncology (SIOPE), QUARTET project, Brussels, Belgium; 4The European Organisation for Research and Treatment of Cancer (EORTC) Headquarters, RTQA, Brussels, Belgium; 5 “Babes-Bolyai” University, Faculty of Physics, Cluj-Napoca, Romania; 6University of Medicine and Pharmacy “Iuliu Hatieganu” , Department of Medical Informatics and Biostatistics, Cluj-Napoca, Romania; 7“Babes-Bolyai” University, Faculty of Physics, Cluj-Napoca, Romania
Show Affiliations
Hide Affiliations
Purpose or Objective
The management of primary brain tumors is challenging and complex, with several entities requiring irradiation. Acute toxicities can be dose-limiting and late effects, especially neuro-cognitive impairment, can be detrimental for patients. The ability to spare healthy tissue relies on a proper delineation of OARs. While manual contouring can largely vary between observers and is time consuming, especially with an increasing number of structures that require delineation, AI auto-contouring could be a solution to simplify workflows, shorten planning times and harmonize practice. The aim of this study is to evaluate the accuracy of automatic segmentation for brain OARs and the impact of geometric variations on plan quality and dose distribution.
Material and Methods
Thirty adult patients with primary brain tumors that underwent radiotherapy in a tertiary cancer center were randomly selected and retrospectively, manually recontoured. For each case, a second segmentation was performed using a first version of a deep-learning, guideline-based AI segmentation software (MVision GBAIS™). For 15 selected cases, VMAT plans were generated for each structure set (Manual_Plan/AI_Plan) using the same optimization criteria. For geometric comparison, Dice Similarity Coefficient (DSC), Average and 95% Hausdorff Distance (HD) were calculated. To assess the dosimetric impact, Gamma Analysis (3%/3mm,10% dose cut-off) and DVH comparison were performed,with ΔDmean being the difference between AI_Dmean and Manual_Dmean for each OAR. For non-normal distributed data, a Wilcoxon signed-ranks test was performed for paired data and correlations were assessed with the Spearman correlation coefficient(ρ).
Results
Delineation of the 22 selected brain OARs was significantly faster using the automatic tool, with a median time of 1.13min for AI and 20min for manual (p<0.001). Median DSC and Average HD were 0.69(0.53-0.82) and 1.05mm(0.74-1.54). Median Average/95% HD were highest for MedullaOblongata(1.87/5.05mm) and lowest for Hippocampus_R(0.6/2.63). Highest DSC was found for Brain, Cerebellum and Brainstem (Median 0.96,0.92,0.98) and lowest for OpticTract_L/R, OpticNrv_L/R and OpticChiasm (Median 0.34/0.45, 0.48/0.53, 0.48). DSC was strongly correlated with the structure volume (ρ=0.76, p<0.001), with a higher DSC for large OARs. The median gamma pass rate was 88.44% (85.57-90.83) (Figure1), with no correlation with the median DSC(ρ=0.03,p = 0.913). A significant correlation between ΔDmean and DSC was only found in OpticNrv_L/R and OpticTract_L (ρ0.75/0.54 and 0.52, p<0.05)(Figure2).
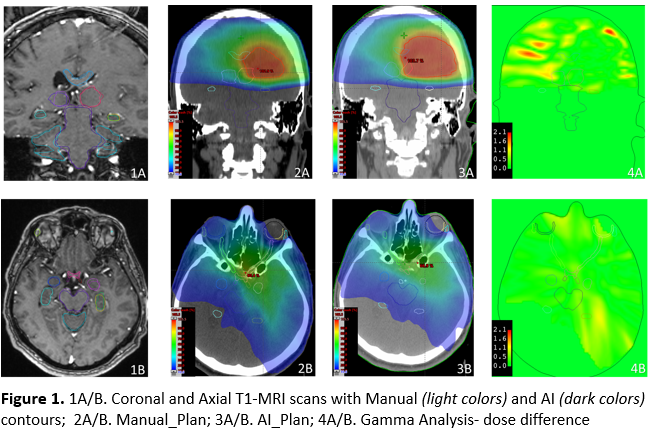
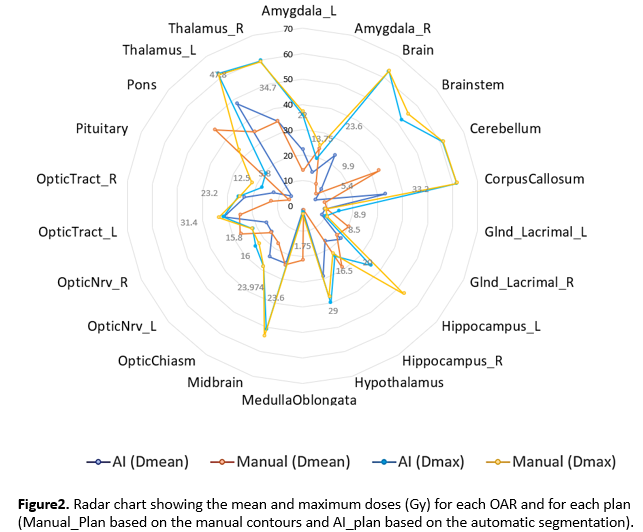
Conclusion
AI-based segmentation is significantly faster than manual delineation. The geometric accuracy is high for large structures, which are also the most laborious to manually delineate, with lower accuracy in small structures, especially the optic apparatus. Despite the geometric differences, DVH parameters showed no difference in most structures, which is also suggested by the high γ index.