Towards automated treatment planning for robotic stereotactic radiosurgery
PO-1643
Abstract
Towards automated treatment planning for robotic stereotactic radiosurgery
Authors: Marcel Nachbar1, Gueliz Acker2,3,4, Carolin Senger2, Keven Mauersberger2, Reinhard Schild2, Wolf-Michael Kuschke2, Daniel Zips5,6
1Charité-Universitätsmedizin Berlin , Department of Radiation Oncology and Radiotherapy, Berlin, Germany; 2Charité-Universitätsmedizin Berlin, Department of Radiation Oncology and Radiotherapy, Berlin, Germany; 3Charité-Universitätsmedizin Berlin, Department of Neurosurgery, Berlin, Germany; 4Berlin Institute of Health, Berlin Institute of Health, Berlin, Germany; 5Charité-Universitätsmedizin Berlin, Department of Radiation Oncology and Radiotherapy, Berlin, Germany; 6German Cancer Consortium (DKTK), , Partner Site Berlin, German Cancer Research Center (DKFZ), Heidelberg, Germany
Show Affiliations
Hide Affiliations
Purpose or Objective
Automated planning is aiming for robust radiotherapy treatment planning allowing extensive simulations. While solutions are available for inverse optimization of treatment plans by iteratively changing constraints, there is no complete solution for CyberKnife (CK) treatment planning. Whereas, constraint based approaches can be used in a second step, the CK requires a definition of collimators prior to optimization. Therefore, within this work an automated analysis of target structures and identification of best possible collimator combinations for treatment plan optimization was developed. This solution was evaluated within a planning study in comparison with clinically used combinations.
Material and Methods
The developed solution was programmed in Python 3.9. A 2D visualization of the planning target volume (PTV) from every 60 degrees in all three dimensions was generated and the coverage of different collimator combinations analyzed. Collimator positions were defined as usable, if within the 2D-Visualisation 90% of the collimator Beams-eye-view (BEV) was covered by the target structure. For each combination and orientation, the number of available, usable collimator positions and the overall structure coverage were calculated. The most effective collimator combination, which covers 98% of the target on over 85% of the 2D BEV with the least number of necessary collimator positions was chosen for further analysis. In a treatment planning study, the clinical treatment (CP) plan of ten meningioma patients was re-optimized with the new collimator combinations and clinical used constraints. Resulting treatment times, PTV coverage, and conformality index (CI) of this automated plan (AP) were analyzed.
Results
For all treatment plans, a suitable collimator combination could be detected with a 2D-coverage of over 98% in over 85% of the evaluated beam angles. In none of the treatment plans the selected collimators corresponded to the collimators used in clinical routine (cf. Table 1).
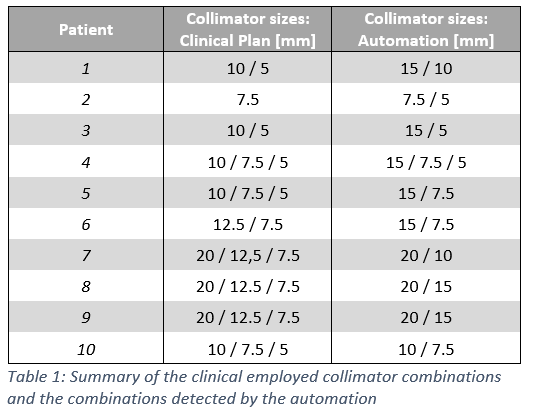
The newly optimized treatment plans revealed a median treatment time of 31 minutes (AP) compared with 34 minutes in CP . The PTV coverage decreased from median 99.2% (CP) to 98.2% (AP) and the CI increased from in median 1.17 (CP) to 1.18 (AP) (cf. Figure 1).
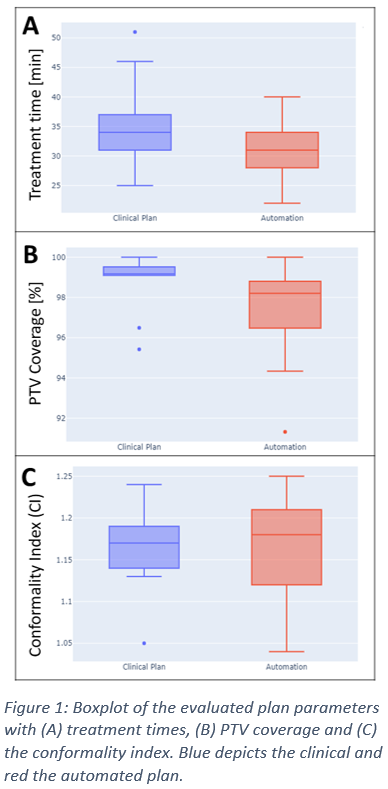
Conclusion
This planning study demonstrates automatic collimator determination as a first step for complete automation of CyberKnife treatment planning. While no reproduction of collimators used in clinical routine was possible, the planning study showed a comparable median PTV coverage with shorter treatment times. However, for specific treatments with critical adjacent OARs, the automated treatment plan failed to reproduce the clinical coverage within 2%. Whereas the defined settings within this evaluation were set for fast treatment times, a change of allowed collimator overlap leads to higher conformality with greater treatment times. Therefore integrating this parameter as a function of adjacent OARs needs to be evaluated in further studies.