Interpreting generalizability of automatic GTV segmentation in locally advanced cervical cancer
PD-0333
Abstract
Interpreting generalizability of automatic GTV segmentation in locally advanced cervical cancer
Authors: Rahimeh Rouhi1,2, Stéphane Niyoteka1,2, Alexandre Carré1,2, Samir Achkar2, Pierre-Antoine Laurent1,2, Mouhamadou Bachir BA2,3, Cristina Veres1,2, Théophraste Henry1,4, Roger Sun1,2, Sophie Espenel2, Cyrus Chargari1,2, Eric Deutsch1,2, Charlotte Robert1,2
1Université Paris-Saclay, Institut Gustave Roussy, Inserm, Radiothérapie Moléculaire et Innovation Thérapeutique, Villejuif, France; 2Gustave Roussy Cancer Campus, Department of radiation oncology, Villejuif, France; 3University Hospital Center of Dalal Jamm, Radiotherapy Department, Guédiawaye, Senegal; 4Gustave Roussy Cancer Campus, Department of medical imaging, Villejuif, France
Show Affiliations
Hide Affiliations
Purpose or Objective
Locally advanced cervical cancer (LACC) is the 4th most common cause of cancer death worldwide. Automatic segmentation of Gross Tumor Volume (GTV) in T2-Weighted (T2W) MR images can be of great help to radiation oncologists by saving time and providing more robust delineation for treatment planning. Despite the promising results of deep neural networks in the automatic segmentation of organs at risk and target volumes, these methods have been only marginally applied to the segmentation of target volumes in LACC, especially since their generalization to real-world images remains a challenge. In this work, we analyzed the generalizability of a deep neural network model trained on heterogeneous data for GTV segmentation in LACC, with the aim to link segmentation results with inter-image differences evaluated based on radiomic features. This strategy could be time-saving during treatment planning by selecting images to which automatic segmentation leads a success/failure.
Material and Methods
We collected two cohorts of pre-radiotherapy images taken by, respectively, 30 and at least 7 different scanners, from 115 and 32 patients, treated for LACC at different centers. The former was used for training using a 5-fold cross-validation and the latter for testing. The segmentation was done by ensemble 2D SegResNet and the results were evaluated using the Dice Similarity Coefficient (DSC) and 95th Hausdorff distance (95HD). Different classification methods were trained considering radiomic features extracted from segmented GTVs of the training cohort as inputs with the goal to determine images for which DSC≥0.7 or DSC<0.7. Subsequently, the resulting model was applied to GTVs of the test set.
Results
The ensemble model segmented successfully 56% of test images with DSC>=0.70 (Figure 1). In classification, we obtained a precision (𝓟) of 0.738, an F1-score (𝓕) of 0.656, and an accuracy (𝓐) of 0.673 using linear discriminant analysis on the test set, resulting in a correct identification of 0.41 and 0.90 of segmented cases with DSC <0.70 and DSC>=0.70, respectively.
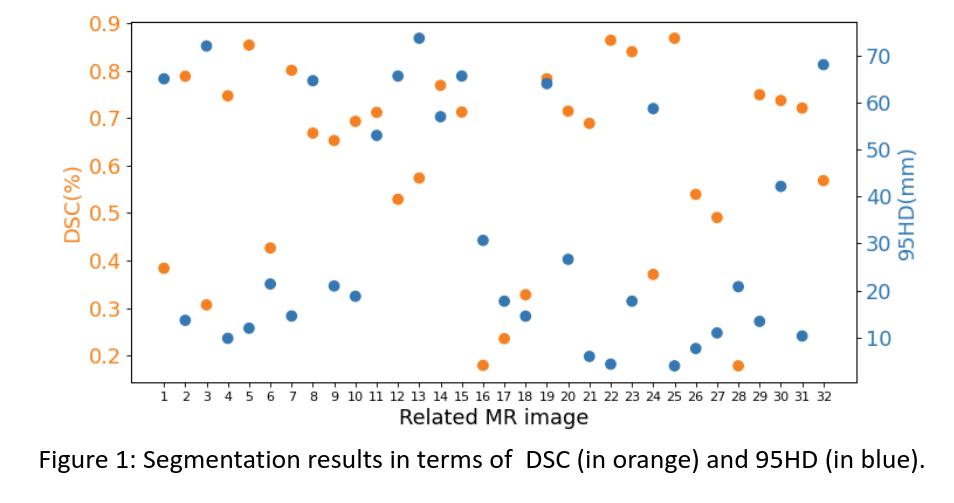
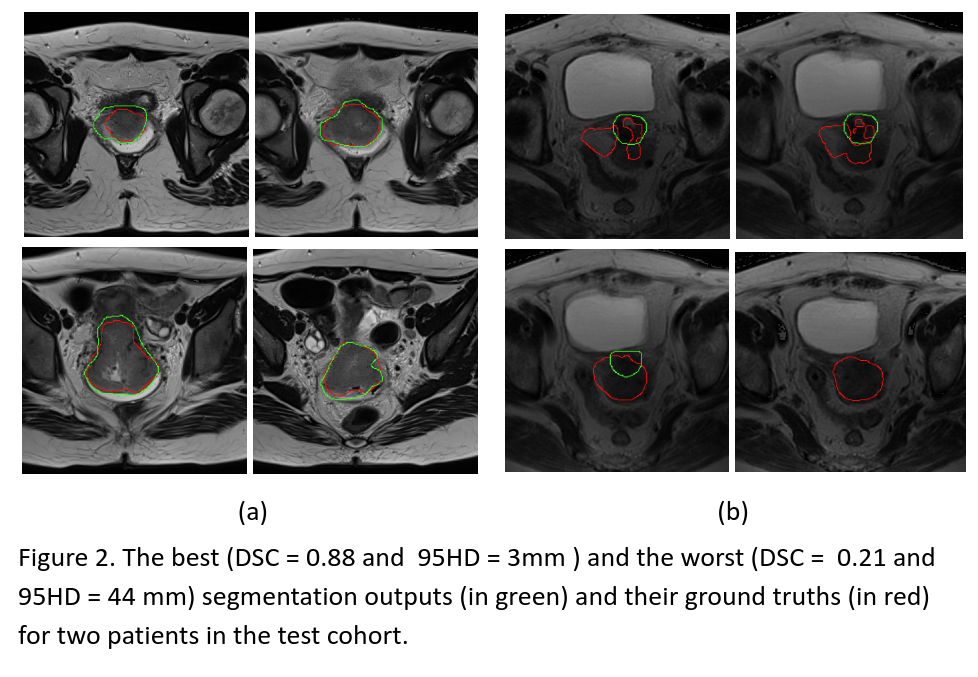
Conclusion
In addition to the developed network that tackled the very complex task of automatically defining target volumes from MRI images, we presented an interpretation of the generalizability of automatic GTV segmentation in LACC trained on heterogeneous MR images, which is of primary interest for real-world applications. In our future work, we will extract complementary quality-based features to enhance the identification of segmentation failures.