Human validation of a Deep Learning MRI-based Synthetic CT for RT Planning
PD-0903
Abstract
Human validation of a Deep Learning MRI-based Synthetic CT for RT Planning
Authors: Leonardo Crespi1,2, Samuele Camnasio1, Damiano Dei3,4, Nicola Lambri3,5, Pietro Mancosu5, Marta Scorsetti3,5, Daniele Loiacono1
1Politecnico di Milano, Dipartimento di Elettronica, Informazione e Bioingegneria, Milan, Italy; 2Human Technopole, Center for Health Data Science, Milan, Italy; 3Humanitas University, Department of Biomedical Sciences, Pieve Emanuele (MI), Italy; 4IRCCS Humanitas Research Hospital, Department of Radiotherapy and Radiosurgery, Rozzano (MI), Italy; 5IRCCS Humanitas Research Hospital, Medical Physics Unit - Radiotherapy and Radiosurgery Department, Rozzano (MI), Italy
Show Affiliations
Hide Affiliations
Purpose or Objective
MRI-based planning typically requires (a) MRI sequences to exploit the MRI high soft tissue contrast and (b) CT series with electron density, for dose calculation. However, the use of both imaging modalities results in a more complex and time-consuming RT workflow (e.g., image registrations, multiple acquisitions…). In this work, we proposed a deep learning (DL) model to generate a synthetic CT (sCT) from in-phase (IP) and out-of-phase (OOP) MRI sequences to streamline the MRI-based planning workflow.
Material and Methods
CycleGAN, a DL model consisting of two generative adversarial networks (GAN) trained to generate synthetic images across different modalities, was used for the generation of sCTs from IP/OOP MRI pairs. Two different models were trained. The first one was trained on the Chaos grand-challenge dataset, including 1300 T1-weighted IP/OOP MRI slices, 1087 T2-weighted MRI slices, and 6407 CT slices acquired from the abdominal-thoracic region of 40 patients. The second model was trained using an internal dataset as the source for the CT images, including 5970 CT slices acquired in the abdominal-thoracic region of 100 patients. The two models were used to generate consistent sCTs with the respect to the real CTs available in the two different datasets. To assess the models’ performance, the sCTs generated from MRI images of 20 test patients not included in the training data were used. The following metrics were considered to compare the generated images to the real ones: Frechet inception distance (FID), Kullback-Leibler divergence (KL), histogram correlation (HC). Finally, 12 RT experts (i.e., radiation oncologists and medical physicists) from a single center blindly evaluated real and synthetic CT images.
Results
Both models were rather accurate and realistic (see some examples in Figure 1). Figure 2 shows the FID, KL, and HC metrics computed on sCTs, grouped in 10 different cranial-caudal axial views along the abdominal-thoracic region (where FID and HC are normalized w.r.t. values of metrics on real images). The sCTs image quality depended on the position: the slices generated from the central part of the MRI package were better than those generated at the cranial-caudal periphery. However, on average FID, KL, and HC metrics (respectively 1.03, 1.93, and 0.97 on the AuToMI dataset and 1.33, 2.09, and 0.95 on the Chaos dataset) suggest that the quality of images is good and reasonably close to real images (especially for AuToMI dataset). Finally, the RT experts were not able to distinguish between real and synthetic CT images: the statistical analysis performed on their evaluations showed no significant differences between real and synthetic CT images for both models (p-value 0.933 and 0.930).
Fig. 1
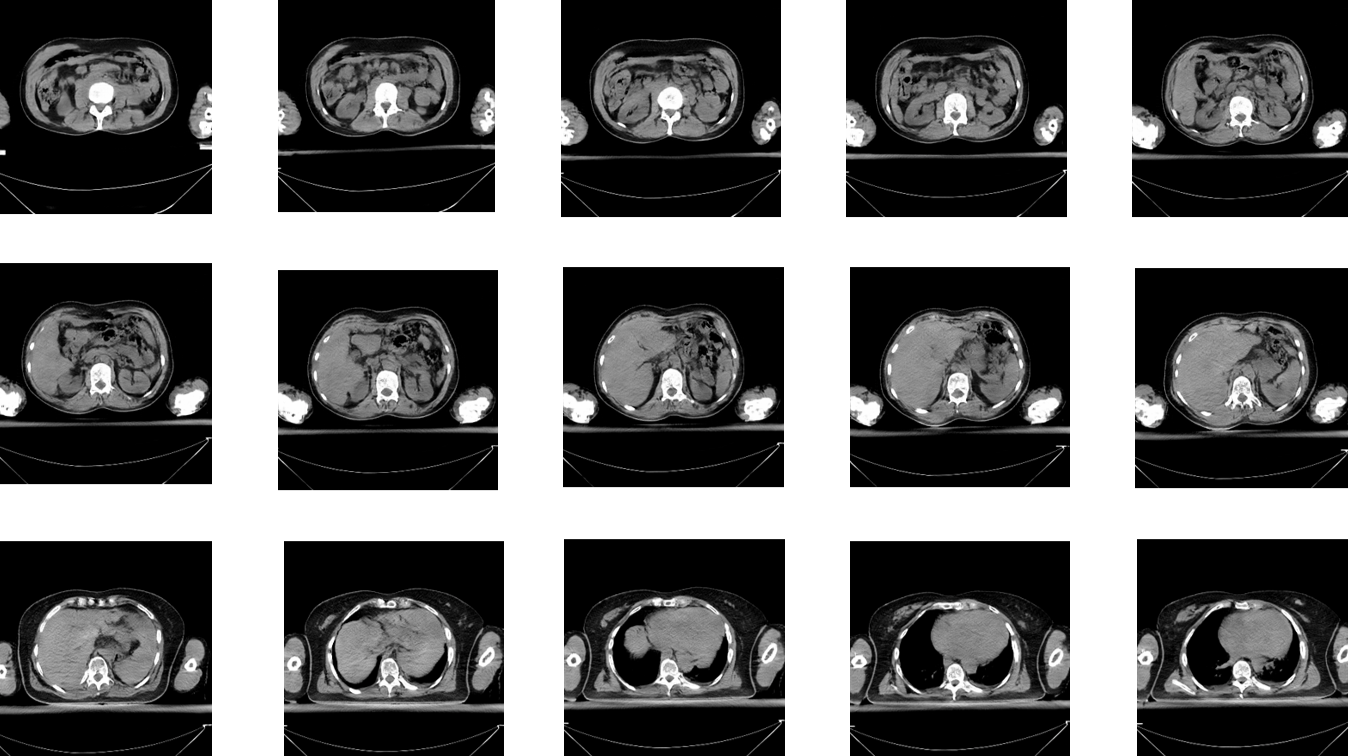
Fig. 2
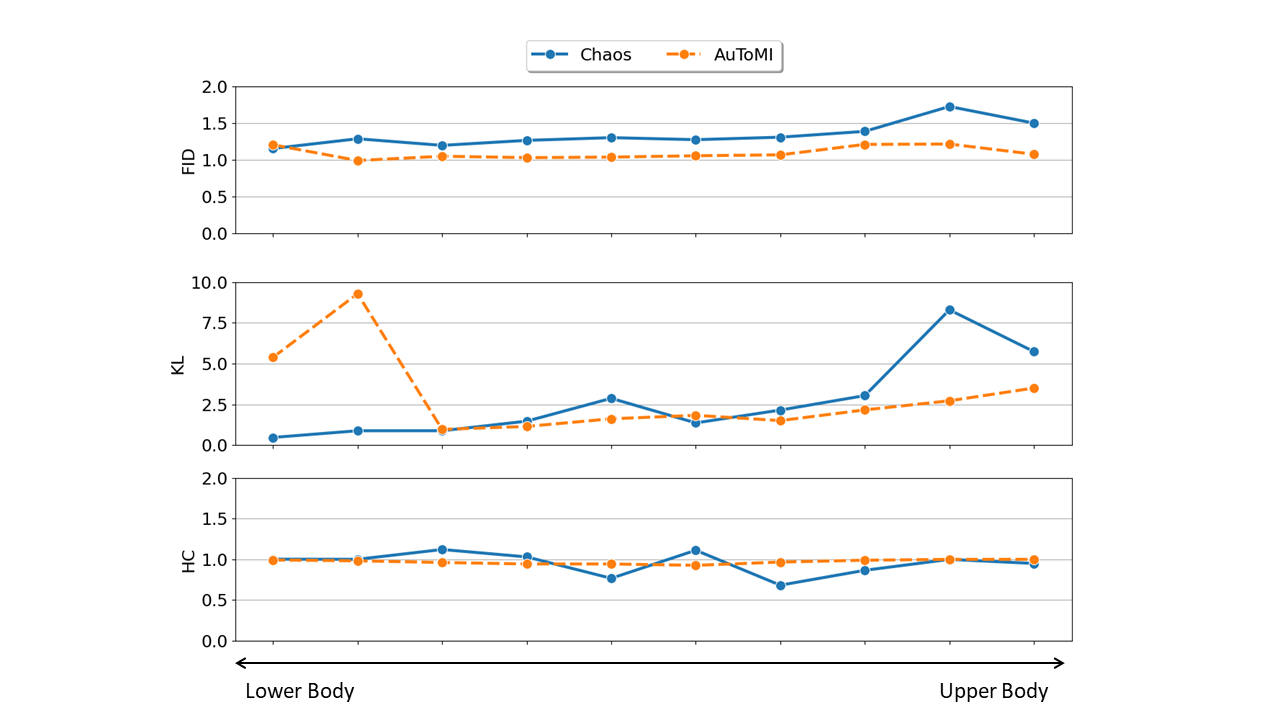
Conclusion
Human validation indicated that DL approaches can generate realistic images. However, further validation is required to assess whether the images can be used in clinical practice to streamline the whole RT process.
This work was supported by grant GR-2019-12370739.