Error identification in time-resolved treatment verification with multiple instance learning
PD-0899
Abstract
Error identification in time-resolved treatment verification with multiple instance learning
Authors: Cecile Wolfs1, Rutger Hendrix2, Evelyn de Jong3, Frank Verhaegen1
1GROW School for Oncology - Maastricht University Medical Center, Radiation Oncology (Maastro), Maastricht, The Netherlands; 2Eindhoven University of Technology, Department of Biomedical Engineering, Medical Image Analysis group, Eindhoven, The Netherlands; 3Instituut Verbeeten, Medical Physics, Tilburg, The Netherlands
Show Affiliations
Hide Affiliations
Purpose or Objective
In recent years, artificial intelligence (AI) has been introduced for error detection and identification during radiotherapy treatment dose verification. While dynamic treatments such as IMRT and VMAT have become standard practice, dose verification is still performed in a time-integrated (TI) manner (i.e., summed over a treatment beam). However, it has been shown previously that errors can be hidden by TI verification methods, and that time-resolved (TR) methods are preferable [1]. Yet, the use of TR data, and its associated increase of dataset size and computational needs, poses challenges for interpretation of the data and training of AI models for error identification. The aim of this work was to develop an efficient AI method for identification of realistic simulated errors in TR dose verification data.
Material and Methods
Clinically realistic ranges of treatment errors (anatomical, positioning and mechanical) were simulated for 46 lung cancer patients (53 treatment plans, 106 VMAT arcs). TR portal dose images were predicted for CT images and treatment plans with and without errors, and compared using (3%, 3 mm) gamma analysis. The complete dataset consisted of 26659 TR gamma maps, with dimensions 128x128x97 pixels (i.e., 97 segments/timepoints of 2D 128x128 images). For efficient AI model training, multiple instance learning (MIL), a technique novel to the radiotherapy field, was employed. The idea behind MIL is that a data sample (in this work a TR gamma map) can be split in patches (in this work in separate segments) that are used separately as input for the AI model, but the model still learns the label (in this work the error type or magnitude) over all patches, i.e. a complete data sample. The MIL model (Figure 1) consisted of (1) a convolutional neural network (CNN) for extracting features from each segment of the TR gamma maps, (2) selection of the most informative segments, (3) a memory cell to retain the temporal information embedded in the data, and (4) an encoder-decoder for performing the final error identification. Two of these models were trained for different purposes: 1) classification of the error type (e.g., tumor regression, patient rotation or MLC systematic error) and 2) classification of the error magnitude (e.g., tumor regression > 50%).
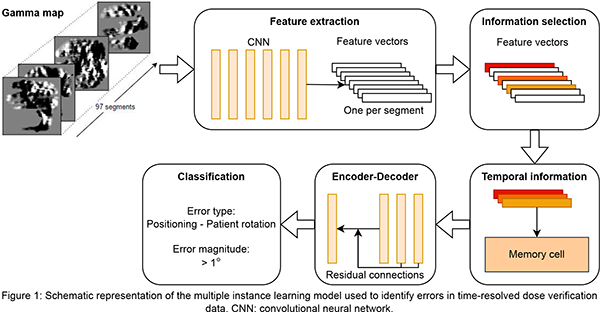
Results
Accuracy of the MIL model on TR data was 86% and 74% for error type and magnitude classification, respectively; outperforming a CNN trained on TI data, which scored 79% and 62%. Additionally, step 2 of the MIL model allows some insight into which segments/linac angles are important for the model to identify the correct errors.
Conclusion
MIL is an efficient method for identifying treatment errors in large-scale TR dose verification data. Besides being computationally efficient, it improves error identification accuracy compared to CNNs for TI data, and has the potential to provide insight into model decisions.
References
1. Schyns et al. 2016 Phys Med Biol