CBCT Deep Learning Model to Predict Extreme Prostate Motion Verified by Ultrasound Probe
Clifford Ghee Ann Chua,
Singapore
PD-0660
Abstract
CBCT Deep Learning Model to Predict Extreme Prostate Motion Verified by Ultrasound Probe
Authors: Clifford Ghee Ann Chua1, Eric Pei Ping Pang1, Wei Yang Calvin Koh1, Azka Danish Abdul Mutalib1, Wen Chuan Chong1, Kah Seng Lew1, Ping Lin Yeap1, Khong Wei Ang1, Sung Yong Park1,2, James Cheow Lei Lee1, Jeffrey Kit Loong Tuan1,2, Hong Qi Tan1
1National Cancer Centre Singapore, Division of Radiation Oncology, Singapore, Singapore; 2Duke NUS Medical School, Oncology Academic Clinical Program, Singapore, Singapore
Show Affiliations
Hide Affiliations
Purpose or Objective
It is known that organs in pelvis including prostate are moving in milliseconds timeframe under the influence of rectal volume, bladder volume, and change of muscle tension. These intrafractional motions are known to be stochastic and could degrade the dose distributions. As such, this study aimed to investigate the hypothesis if deep learning applied on CBCT image acquired prior to actual daily treatment could predict extreme prostate motion during the treatment course.
Material and Methods
24 patients who had CBCT scans with intrafraction motion monitoring in place using Clarity (Elekta AB, Stockholm, Sweden), a 4D transperineal ultrasound device. A total of 465 pretreatment CBCT images and 58.9 hours of intrafraction prostate displacement data were used to train and test a ‘built from scratch’ 3D Convolutional Neural Network (CNN) to predict intrafractional shift in prostate. Each pretreatment CBCT images has a corresponding ultrasound time trace signal obtained during treatment. The CBCT images were binarily classified based on the corresponding intrafraction motion ultrasound time trace during treatment. When the vector length exceeds a threshold of 5 mm for 5 seconds, the CBCT image is classified as an extreme prostate motion event. The images were stratified by treatment fractions and the model was trained and tested on every image through a 5-fold cross validation. The architecture of the 3D CNN used is adapted from Hasib et al (2020). The area under the curve (AUC) of receiver operating characteristic (ROC) curve was used for the model evaluations and Brier Score was calculated to measure the accuracy of probabilistic predictions. Gradient-weighted Class Activation Mapping (Grad-CAM) of the model was generated to provide insights of model's learning activity.
Results
The mean AUC score of 0.82 ± 0.02 (mean ± std) and Brier Score of 0.182 on validation dataset and AUC score 0.78 ± 0.03 and Brier Score of 0.201 on test dataset with the Brier Score were shown in Figure 1. Grad-CAM images from 2 true negative and 2 true positive case in Figure 2 suggest that the bladder, femur and rectum could be a factor in predicting prostate motion. An interesting note is the prostate does not seem contribute as much in the prediction.
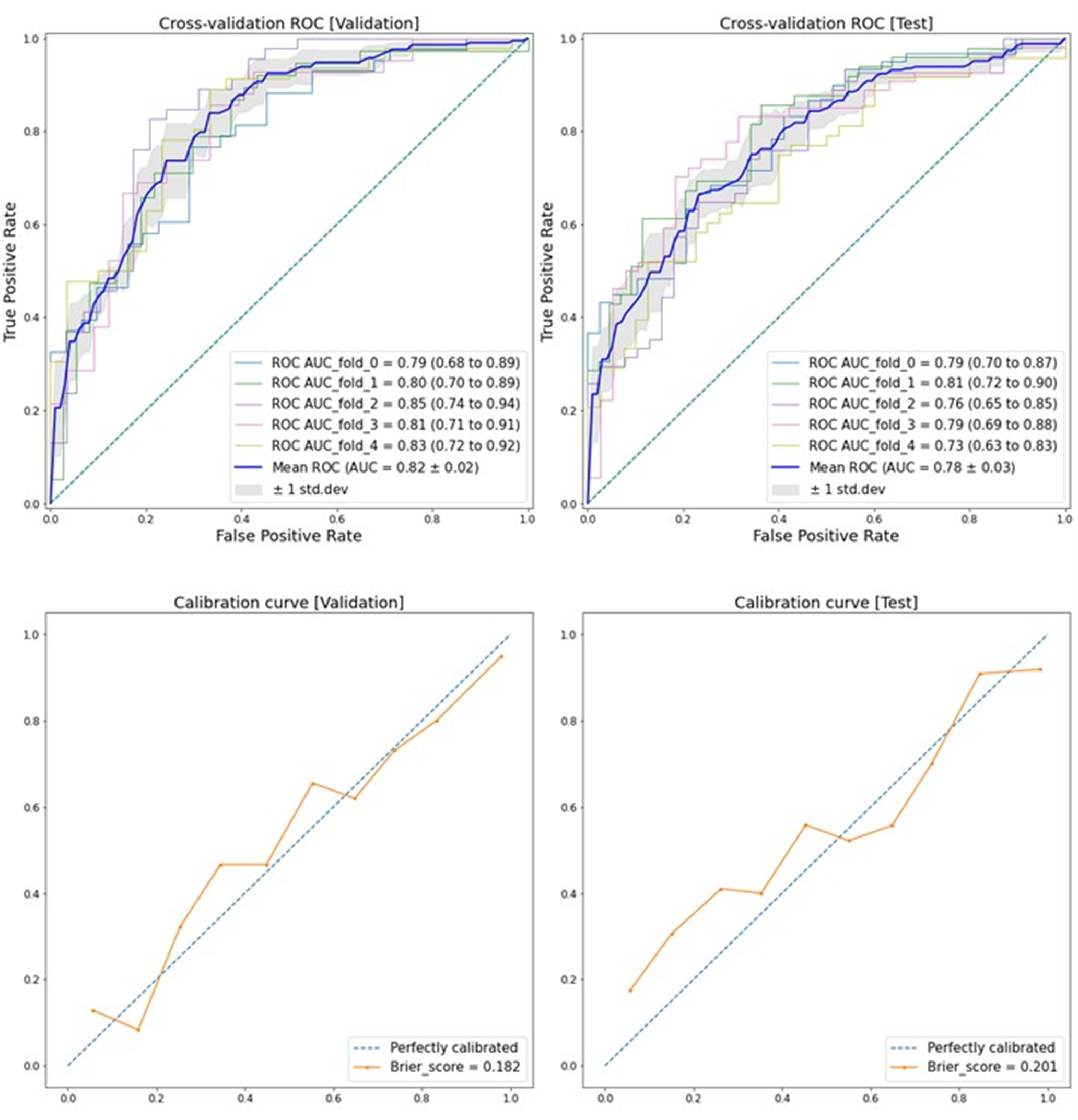
Figure 1
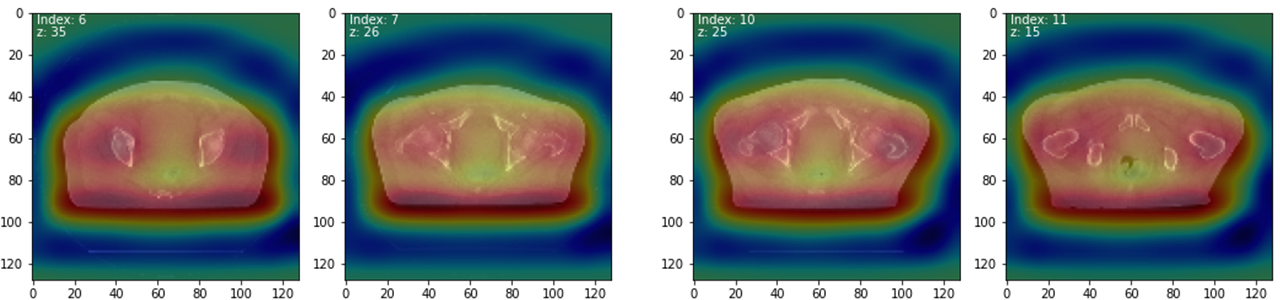
Figure 2
Conclusion
The result of this study indicates the feasibility of predicting intrafractional prostate motion from CBCT images with high accuracy of probabilistic predictions. However, it would require at least 1 fraction to be treated first. As such this may help with quality assurance tests and possibly improve patient’s overall treatments by implementing adaptive planning.