Sub-second speed 4D-CT image registration using deep learning
Thomas van der Meulen,
The Netherlands
PD-0657
Abstract
Sub-second speed 4D-CT image registration using deep learning
Authors: Thomas van der Meulen1, Oscar Pastor-Serrano1, Steven Habraken2, Zoltán Perkó1
1Delft University of Technology, Radiation Science and Technology, Delft, The Netherlands; 2Erasmus University Medical Center, Department of Radiotherapy, Rotterdam, The Netherlands
Show Affiliations
Hide Affiliations
Purpose or Objective
From contour propagation to dose accumulation, fast image registration is crucial for treating moving targets. Traditional registration methods optimize an objective function per pair of images, typically resulting in minutes of computation times, making them inapplicable in online and real-time adaptive treatment schemes. To overcome this challenge, our work presents a deep learning based accurate and robust registration model that can register pairs of images in few tens of milliseconds.
Material and Methods
We use a Laplacian Pyramid Image Registration Network (LaPy) for image deformation and contour propagation between breathing phases of 4DCT scans from non-small cell lung cancer patients. The network takes a fixed and moving image as input and predicts the full deformation field warping the moving image to match the fixed one. The LaPy model is trained using 65 planning and repeat 4D-CT scans from 20 different patients. Each 4D-CT is discretized into 10 phases per breathing cycle, resulting in 100 image pairs (90 combinations between phases plus 10 identity registrations). During training, all images are normalized to the range [0,1] and shifted ±10 mm for data augmentation. For model evaluation, 7 additional 4D-CTs unseen during training from 7 patients from the same dataset are used to compare the LaPy model to the registration software Elastix (computing b-spline deformations with parameters from a previously published study). We use the mean squared error (MSE) between the fixed and warped normalized image values to measure image similarity, and the mean surface distance (MSD) and Dice score to assess contour overlap for the tumor, lungs, heart, carina and esophagus. To test model robustness, we additionally evaluate the MSE on 2 datasets of 7 and 5 patients with 4D-CTs recorded with a different machine.
Results
The LaPy model can accurately infer the deformation field between the 4D phases for the 7 testing patients, achieving very low MSE of 6.029E-5±6.065E-5, comparable to Elastix’s error of 6.026E-5±3.014E-5. For the 2 external datasets from a different machine and location, the MSE is similarly low, 6.96E-5±3.95E-5 and 7.02E-5±3.80E-5, respectively. Table 1 shows that the model can reach significantly lower MSD and higher Dice score compared to the input moving images, demonstrating LaPy’s ability to model breathing anatomical deformations. Figure 1 further underlines the excellent agreement between the predicted and fixed images. With average GPU prediction times of 23±2 ms, the LaPy model significantly outperforms Elastix’s computing times of 248±11 s.
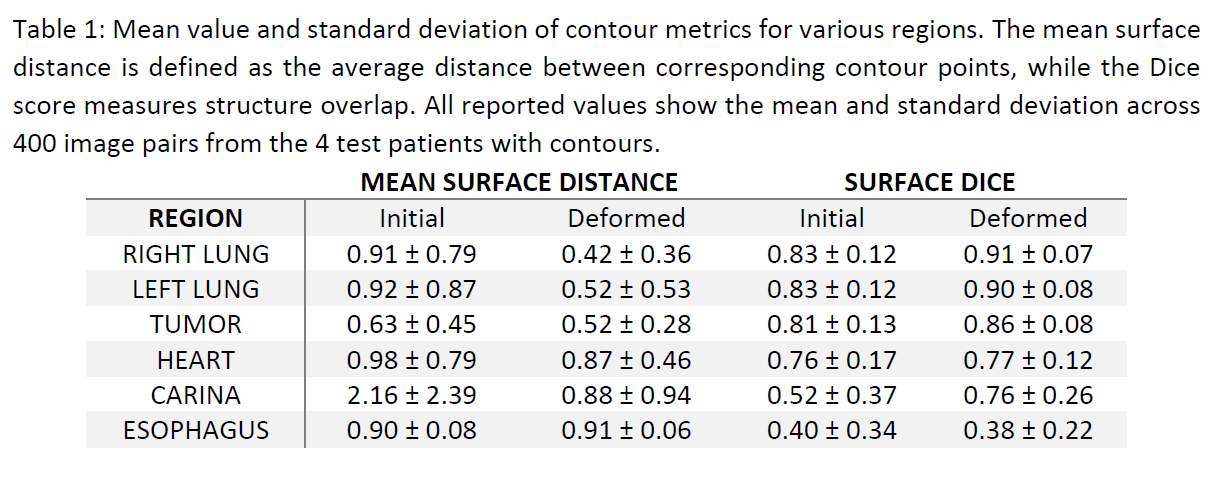
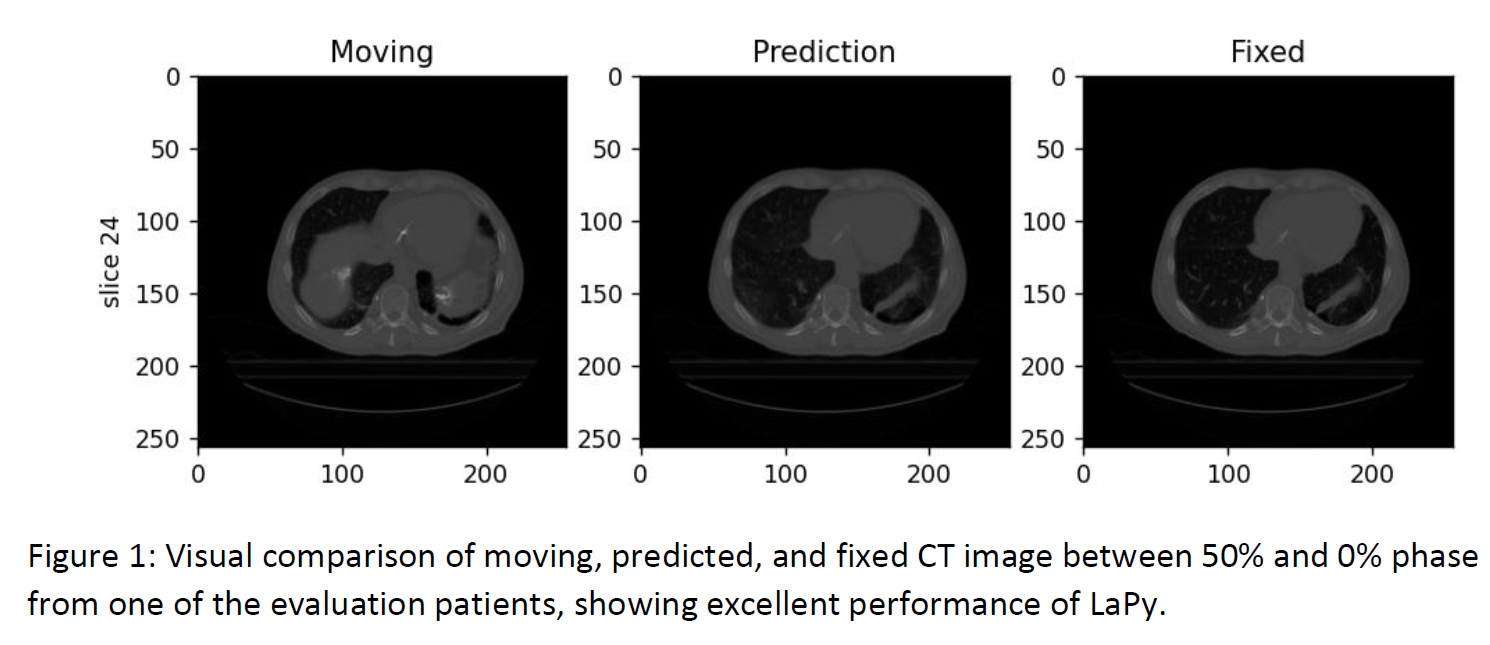
Conclusion
Being several orders of magnitude faster than conventional methods, LaPy can be applied to speed-up 4D-CT workflows, providing accurate deformations between phases in a few tens of milliseconds. Thus, it can be highly useful for treating moving tumors, helping to derive internal target volumes or serving as a QA tool providing fast dose accumulation in charged particle treatments affected by interplay effects.