For CE, median Dice scores were 0.81 (95% CI 0.71-0.83) and 0.82 (95% CI 0.74-0.84), while median HD95 were 5.91 (95% CI 2.8-16.4) and 3.16 (95% CI 2.8-7.1) for Operator-1 and Operator-2, respectively (Figure 2 A). For NE, median Dice scores were 0.65 (95% CI 0.56-0,69) and 0.63 (95% CI 0.57-0.67), while median HD95 were 16.1 (95% CI 10.6-22.2) and 16.7 (95% CI 9.4-23.2), respectively (Figure 2 C).
Comparing volume sizes, we found excellent ICC of 0.90 (p<0.001) and 0.95 (p<0.001), for CE, respectively, and 0.97 (p<0.001) and 0.90 (p<0.001), for NE, respectively. Moreover, there was a strong Spearman’s correlation of 0.83 (p<0.001) between RANO-volumes and HD-GLIO-volumes.
Taken together, we found that for CE-volumes, the Dice similarity coefficients and HD95 had better scores between operator and HD-GLIO segmentation, than for inter-operator scores. This indicates that the HD-GLIO segments had a shape and location somewhat intermediate between the Operator-1 and Operator-2 manual delineations. Adding dilations further increased the Dice-scores and reduced the relative performance difference between individuals. For NE-volumes, we found that Dice similarity and HD95 showed poorer agreement between operator and HD-GLIO than inter-operator scores. This was largely because manual NE-delineations held substantially larger volumes than HD-GLIO predictions
Average processing time was < 6 minutes per dataset.
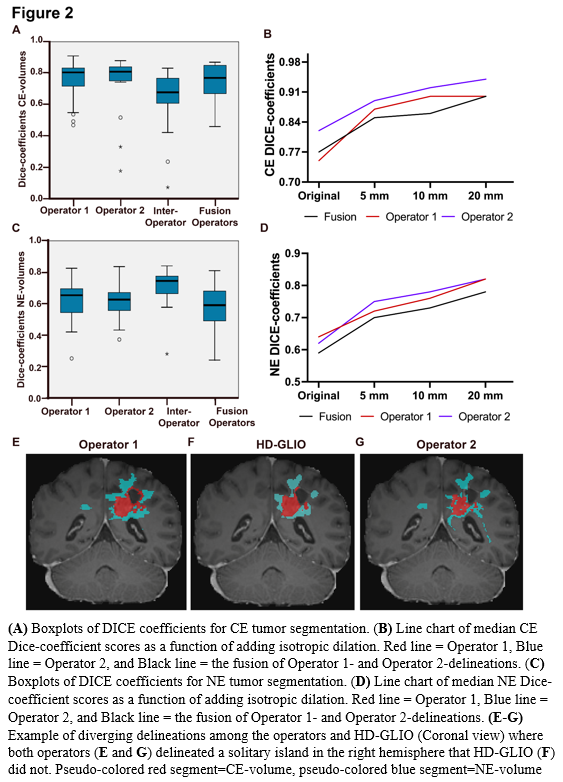