Comparison of SPArc algorithm efficiency using beamlet-based and beamlet-free optimization schemes
PO-1982
Abstract
Comparison of SPArc algorithm efficiency using beamlet-based and beamlet-free optimization schemes
Authors: Sophie Wuyckens1, Guillaume Janssens2, Lewei Zhao3, Xuanfeng Ding3, Macarena Chocan Vera1, Hamdiye Ozan1, Ana Barragan Montero1, Edmond Sterpin1,4, John Lee1, Kevin Souris5,1
1UCLouvain, MIRO, Brussels, Belgium; 2Ion Beam Applications SA, R&D, Louvain-La-Neuve, Belgium; 3Beaumont Health, Department of Radiation Oncology, Royal Oak, USA; 4KULeuven, Department of Oncology, Leuven, Belgium; 5Ion Beam Applications SA, IBA Dosimetry, Louvain-La-Neuve, Belgium
Show Affiliations
Hide Affiliations
Purpose or Objective
In 2016, spot-scanning arc proton therapy (SPArc) algorithm [1] was introduced to solve the energy sequencing problem raised by the proton arc modality. The large number of irradiation angles translates indeed into a long beam delivery time if conventional plan optimization is performed. To stay within a reasonable timing, SPArc starts from a plan with very few irradiation directions and then iteratively splits the beams until a desired arc sampling frequency is reached. A filtering operation is then performed to restrict the number of energy layers to one in each angular sector. SPArc produced clinical arc plans competing with the conventional treatments in different disease sites. However, one of the obstacles to implementing SPArc in the clinic is its computing efficiency (time and memory usage). The main reason is that at each splitting operation, the beamlet matrix (influence map) has to be recomputed for all the spots due to the restructuring of the plan. To address this problem, we propose another method that avoids computing huge beamlet matrices, namely, the beamlet-free optimizer, an in-house Monte Carlo dose computation.
Material and Methods
The two approaches (SPArc beamlet vs SPArc beamlet-free) are compared and evaluated on three patients: a brain, a lung, and a liver tumor case. The optimizations run in an in-house treatment planning system, OpenTPS. The beamlet-based method uses a commercial linear programming solver through the Python API while the beamlet-free is directly coded in the Monte Carlo dose engine, MCsquare [2], where the successive proton batches are used both to accumulate the dose and to adjust the spot weights in a stochastic gradient descent approach to optimize the plan objectives.
Results
For each disease site, the beamlet-free optimization algorithm is able to bring a similar target coverage as its opponent using the beamlets, while keeping the dose to the organs within the clinical constraints (Figure 1, liver case). Nevertheless, it is computed in a much more efficient way. For example, it reduces the computation time and the memory usage by 87.5% and 60% respectively in the liver case (Table 1). Moreover, it could be even further improved considering that the beamlet-based optimization was using a coarse grid while the beamlet-free was optimizing on the CT grid .
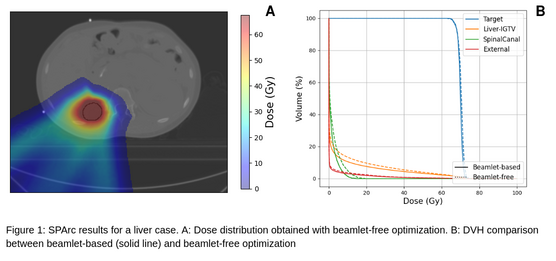
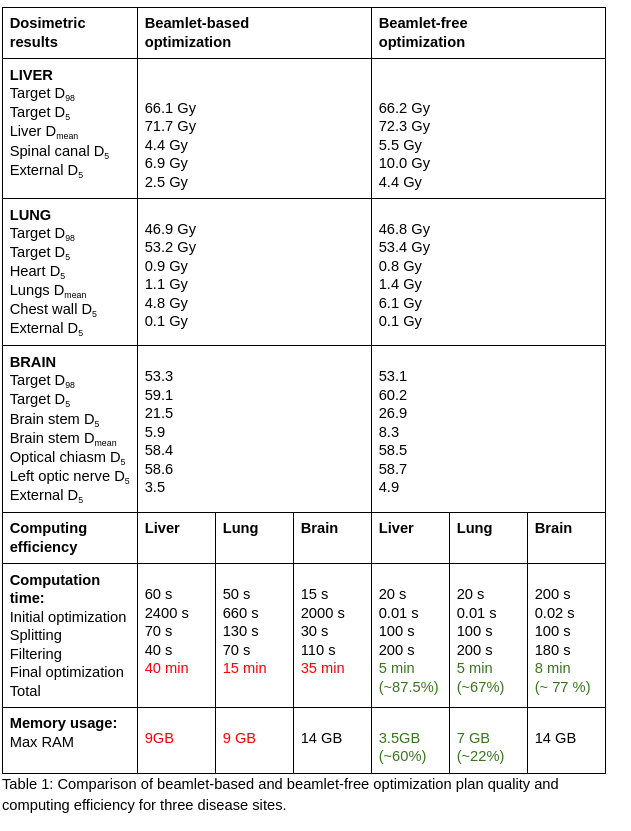
Conclusion
The deployment of SPArc in the clinic could be facilitated by using beamlet-free combined dose computation and optimization in the treatment planning system, which increases the speed and computing efficiency significantly.
[1] Ding X et al. Spot-Scanning Proton Arc (SPArc) Therapy: The First Robust and Delivery-Efficient Spot-Scanning Proton Arc Therapy. Int J Radiat Oncol Biol Phys. 2016;96(5):1107-1116.
[2] Souris K, Lee JA, Sterpin E. Fast multipurpose Monte Carlo simulation for proton therapy using multi- and many-core CPU architectures. Med Phys. 2016;43(4):1700.