QA of deep learning-based synthetic CTs for adaptive proton therapy using uncertainty estimation
Arthur Jr Galapon,
The Netherlands
PO-1942
Abstract
QA of deep learning-based synthetic CTs for adaptive proton therapy using uncertainty estimation
Authors: Arthur Jr Galapon1, Adrian Thummerer1, Dirk Wagenaar1, Mihnea-Gabriel Steiu1, Johannes Langendijk1, Stefan Both1
1University Medical Center Groningen, Radiation Oncology, Groningen, The Netherlands
Show Affiliations
Hide Affiliations
Purpose or Objective
MR imaging offers high-quality soft tissue contrast which is useful for organ-at-risk and target delineations. However, unlike CT images, MR images are not suitable for proton dose calculations. To address this issue, deep learning (DL) techniques are capable of converting MR images into synthetic CTs (sCT). To verify the quality of sCTs, a ground truth CT image is required. In a clinical MR-only workflow such a ground-truth CT is not available. One way of assuring the quality of sCTs is through the use of uncertainty maps. This work aims to investigate the suitability of Monte Carlo dropout-based uncertainty maps to identify and quantify uncertainties of sCTs in the context of adaptive proton therapy.
Material and Methods
A dataset containing 23 same-day registered CT and MR images of head and neck cancer patients was used to train a cycleGAN model for synthetic CT generation. The model utilized a UNET as generator and a PatchGAN classifier as discriminator. Monte Carlo dropout was implemented by activating the dropout layers of the generator during inference. For each patient, the final synthetic CT was the mean of the inferred sCT (n=40) and the model uncertainty map was obtained from the variance.
Three test patients were used to verify the useability of the uncertainty maps. For each test patient, the uncertainty maps were compared to the image difference (sCT – planning CT (pCT)) and the range error map simulated using the proton radiography toolbox of openREGGUI. To facilitate a consistent comparison with the range error maps, the uncertainty maps and image difference were projected along a 90-degree angle, similar to the angle of the proton radiography simulation. Pearson’s correlation coefficient was calculated between the projected uncertainty map and image difference/range error to quantify the correlation.
Results
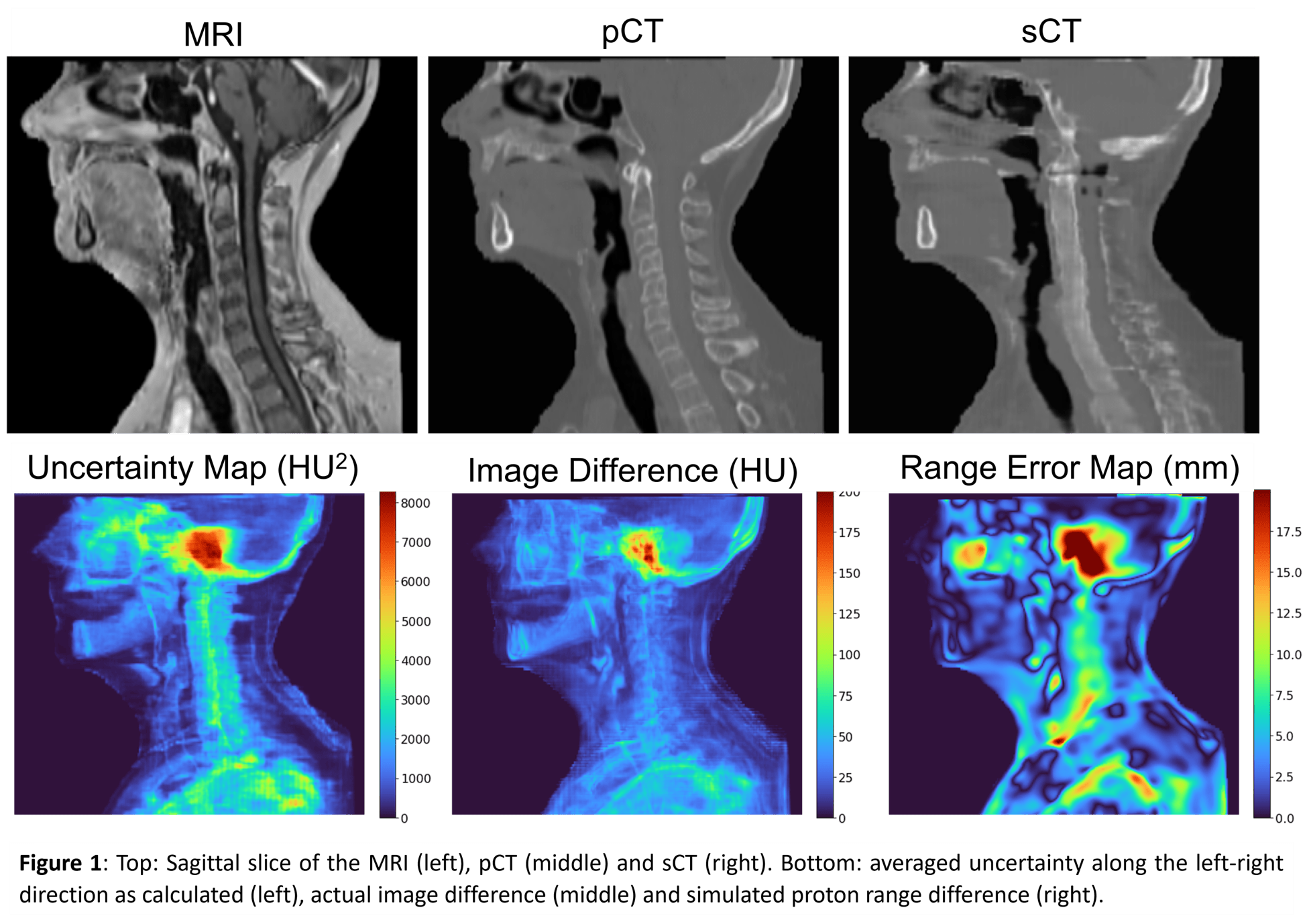
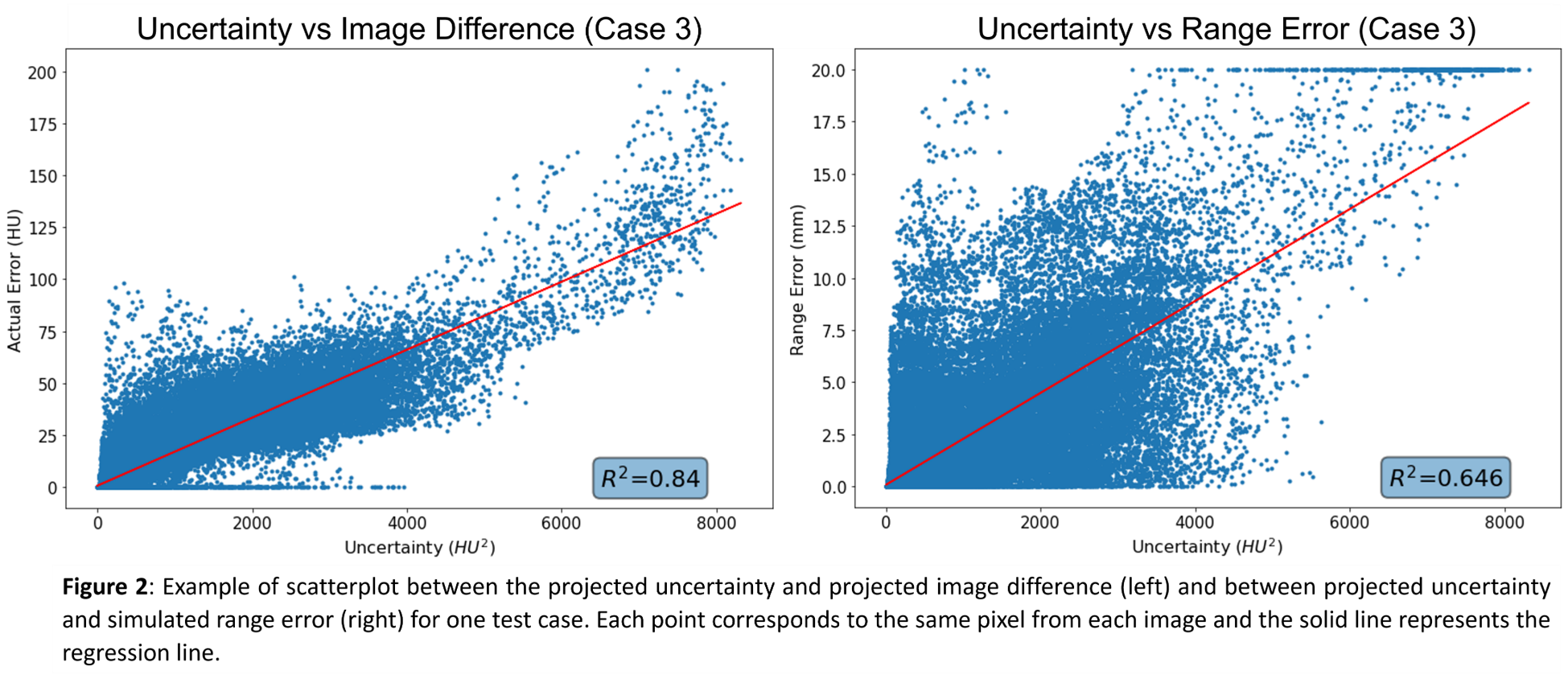
Visual inspection of figure 1 shows that regions of high uncertainties correspond to regions of high errors in the image difference and range error maps, respectively. Evaluation of the correlation coefficient shows a positive correlation between the projected uncertainties and the projected difference, with r1=0.919, r2=0.839, and r3=0.917 (p<0.001) for each test patient, respectively. Comparison with the projected range error map shows a lower correlation coefficient for all test patients, with r1=0.538, r2=0.596, and r3=0.804 (p<0.001), respectively. Figure 2 shows the scatterplot and the R2-value of the regression line for test case 3.
Conclusion
A correlation between the regions of uncertainty and the actual error was observed and shows the potential of using uncertainty maps as a quality assurance tool for DL-based sCT in clinical adaptive proton therapy workflows. However, further analysis is required to evaluate the reliability of using uncertainty maps in assessing the HU accuracy of sCTs .