Deep learning for accuracy prediction of deformably registered contours in prostate radiotherapy
PO-1934
Abstract
Deep learning for accuracy prediction of deformably registered contours in prostate radiotherapy
Authors: Ping Lin Yeap1, Yun Ming Wong2, Ashley Li Kuan Ong1, Jeffrey Kit Loong Tuan1, Eric Pei Ping Pang1, Sung Yong Park1, James Cheow Lei Lee1, Hong Qi Tan1
1National Cancer Centre Singapore, Division of Radiation Oncology, Singapore, Singapore; 2Nanyang Technological University, School of Physical and Mathematical Sciences, Singapore, Singapore
Show Affiliations
Hide Affiliations
Purpose or Objective
Automatic deformable image registration (DIR) is a critical step in adaptive radiotherapy. Manually delineated OAR contours on planning CT (pCT) scans are deformably registered onto daily CBCT scans for delivered dose accumulation. However, evaluation of registered contours requires human experts, which is time-consuming and subjects to high inter-observer variability. This work proposes a deep learning model that allows accurate prediction of Dice Similarity Coefficients (DSC) of registered contours.
Material and Methods
Our dataset comprises 20 prostate patients with 37-39 daily CBCT scans each (n=760). OARs were manually delineated by a radiation oncologist on every CBCT scan. The corresponding pCT scans were deformably registered to each CBCT scan using RayStation v10A (RaySearch Laboratories, Sweden) to generate virtual CT (vCT) scans. DSC between the registered and manual contours were computed. DIR parameters such as the similarity metric and final resolution were varied to determine settings that give the widest range of DSC. Data was augmented by mirroring each scan along the vertical axis, giving 1520 vCT-CBCT pairs in total. A Siamese neural network was trained on the pre-processed vCT-CBCT pairs through a 10-fold cross validation. Given the small dataset, transfer learning using the pre-trained ResNet-50 model was used. Figure 1 shows the network architecture.
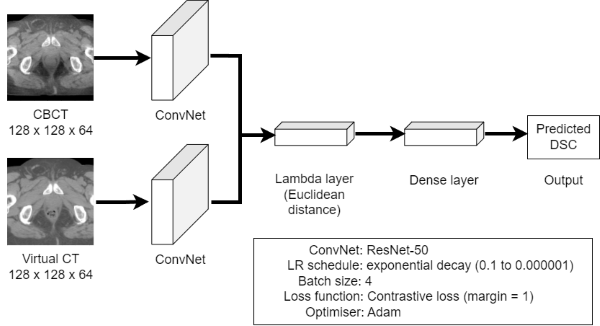
Figure 1. Network architecture, hyperparameters and an input image pair comprising CBCT (top) and deformed vCT (bottom) scans
Results
Table 1. Evaluation metrics of predicted DSC
| Rectum | Prostate | Bladder |
Root mean squared error (RMSE)
| 0.070 | 0.079 | 0.118 |
R² | 0.53 | 0.06 | 0.17 |
Classification threshold | 0.6 | 0.8 | 0.8 |
Accuracy | 0.92 | 0.82 | 0.81 |
% event in test & cross-val set | 87.8%, 87.1% | 82.6%, 84.1% | 83.2%, 78.9% |
Sensitivity | 0.97 | 0.94 | 0.97 |
Specificity | 0.59 | 0.23 | 0.04 |
Positive predictive value (PPV) | 0.95 | 0.85 | 0.83 |
Negative predictive value (NPV) | 0.71 | 0.44 | 0.20 |
As seen in Table 1, the model showed promising results for predicting DSC, giving RMSE of 0.070, 0.079 and 0.118 for rectum, prostate, and bladder respectively on the holdout test set. Clinically, a low RMSE implies that the predicted DSC can be reliably used to determine if further DIR assessment from the physician is required. Taking rectum for instance, considering the event where a registered contour is classified as poor if its DSC is below 0.6 and good otherwise, the model achieves an accuracy of 92%. A sensitivity of 0.97 suggests that the model can correctly identify 97% of poorly registered contours, allowing manual assessment of DIR to be triggered. Figure 2 shows the model predictions on the training and test sets.
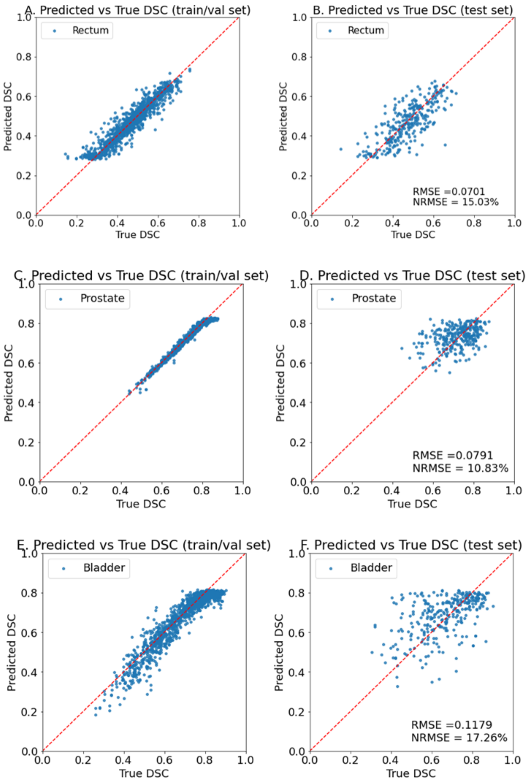
Figure 2. Model predictions on training (left) and holdout set (right) for rectum (top), prostate (middle) and bladder (bottom)
Conclusion
We propose a neural network capable of predicting DSC of deformably registered OAR contours using vCT and CBCT scans as inputs. The model gives reasonably low RMSE and can be used to evaluate accuracy of deformed contours and their eligibility for plan adaptation.